Efficient Quasi-Monte Carlo Sampling For Quantum Random Walks
APPLICATION OF MATHEMATICS IN TECHNICAL AND NATURAL SCIENCES (AMITANS 2020)(2020)
摘要
Quantum random walks are the natural counterpart of the classical random walk, arising when quantum effects are taken into consideration. Many Monte Carlo methods use the classical Brownian motion as a building block. Although it appears naturally in various problems of computational physics, it is also used extensively in the stochastic models used for pricing of financial assets. When quantum random walks are used instead, more complex behaviors can be observed. However, the simulation of a quantum random walk requires more computational resources. By using low-discrepancy sequences instead of pseudorandom numbers, one may hope to decrease the statistical error of the simulation. Another approach for improved efficiency is to use the power of computational accelerators, which are suitable for problems, where a well-ordered sequence of computations is to be performed repeatedly. In our work we concentrated on using GPGPU computing in order to speed-up the operations involved in the generation of the low-discrepancy sequence as well as the subsequent sampling of the quantum random walks. In this work we explain our sampling algorithm and demonstrate its efficiency on model problems from the domain of option pricing.
更多查看译文
AI 理解论文
溯源树
样例
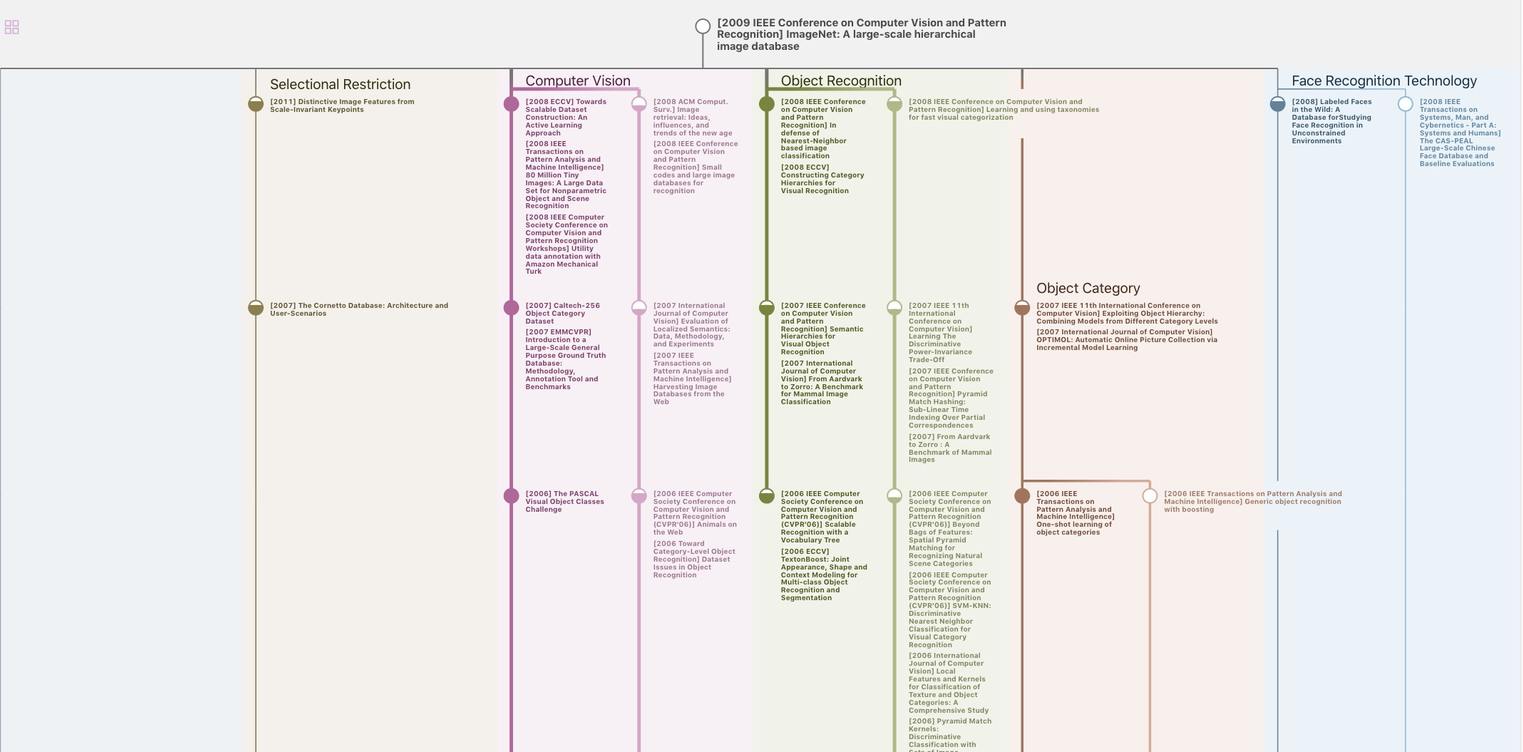
生成溯源树,研究论文发展脉络
Chat Paper
正在生成论文摘要