A Generative Adversarial Neural Network For Beamforming Ultrasound Images
2019 53RD ANNUAL CONFERENCE ON INFORMATION SCIENCES AND SYSTEMS (CISS)(2019)
摘要
Plane wave ultrasound imaging is an ideal approach to achieve maximum real-time frame rates. However, multiple plane wave insonifications at different angles are often combined to improve image quality, reducing the throughput of the system. We are exploring deep learning-based ultrasound image formation methods as an alternative to this beamforming process by extracting critical information directly from raw radio-frequency channel data from a single plane wave insonification prior to the application of receive time delays. In this paper, we investigate a Generative Adversarial Network (GAN) architecture for the proposed task. This network was trained with over 50,000 Field-II simulations, each containing a single cyst in tissue insonified by a single plane wave. The GAN is trained to produce two outputs - a Deep Neural Network (DNN) B-mode image trained to match a Delay-and-Sum (DAS) beamformed B-mode image and a DNN segmentation trained to match the true segmentation of the cyst from surrounding tissue. We systematically investigate the benefits of feature sharing and discriminative loss during GAN training. Our overall best performing network architecture (with feature sharing and discriminative loss) obtained a PSNR score of 29:38 dB with the simulated test set and 14:86 dB with a tissue-mimicking phantom. The DSC scores were 0:908 and 0:79 for the simulated and phantom data, respectively. The successful translation of the feature representations learned by the GAN to phantom data demonstrates the promise that deep learning holds as an alternative to the traditional ultrasound information extraction pipeline.
更多查看译文
关键词
Deep Learning, Generative Adversarial Network, Ultrasound Image Formation, Beamforming, Image Segmentation, Machine Learning
AI 理解论文
溯源树
样例
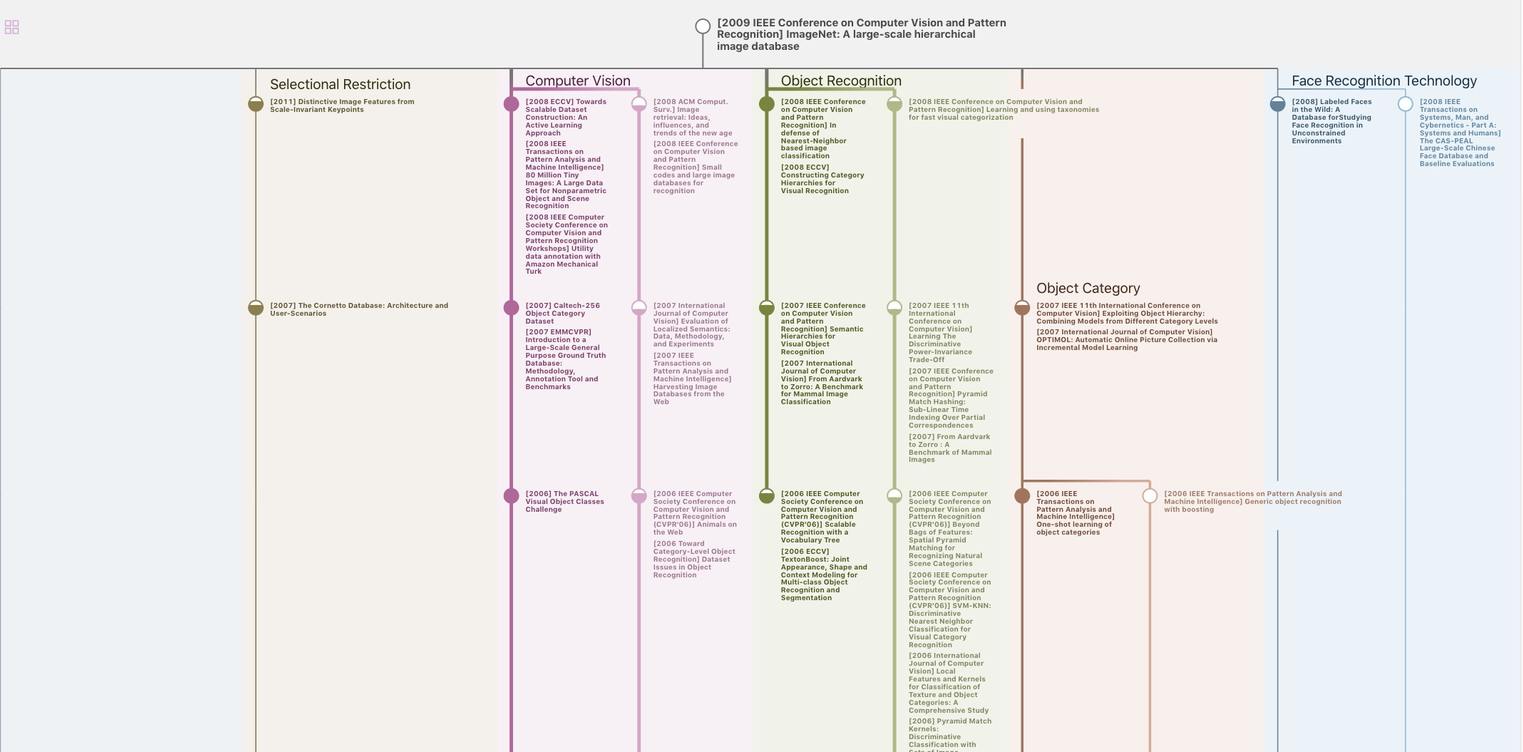
生成溯源树,研究论文发展脉络
Chat Paper
正在生成论文摘要