Tractable Bayesian Teaching
BIG DATA IN COGNITIVE SCIENCE(2017)
摘要
The goal of cognitive science is to understand human cognition in the real world. However, Bayesian theories of cognition are often unable to account for anything beyond the schematic situations whose simplicity is typical only of experiments in psychology labs. For example, teaching to others is commonplace, but under recent Bayesian accounts of human social learning, teaching is, in all but the simplest of scenarios, intractable because teaching requires considering all choices of data and how each choice of data will affect learners' inferences about each possible hypothesis. In practice, teaching often involves computing quantities that are either combinatorially implausible or that have no closed-form solution. In this chapter we integrate recent advances in Markov chain Monte Carlo approximation with recent computational work in teaching to develop a framework for tractable Bayesian teaching of arbitrary probabilistic models. We demonstrate the framework on two complex scenarios inspired by perceptual category learning: phonetic category models and visual scenes categorization. In both cases, we find that the predicted teaching data exhibit surprising behavior. In order to convey the number of categories, the data for teaching phonetic category models exhibit hypo-articulation and increased within-category variance. And in order to represent the range of scene categories, the optimal examples for teaching visual scenes are distant from the category means. This work offers the potential to scale computational models of teaching to situations that begin to approximate the richness of people's experience.
更多查看译文
AI 理解论文
溯源树
样例
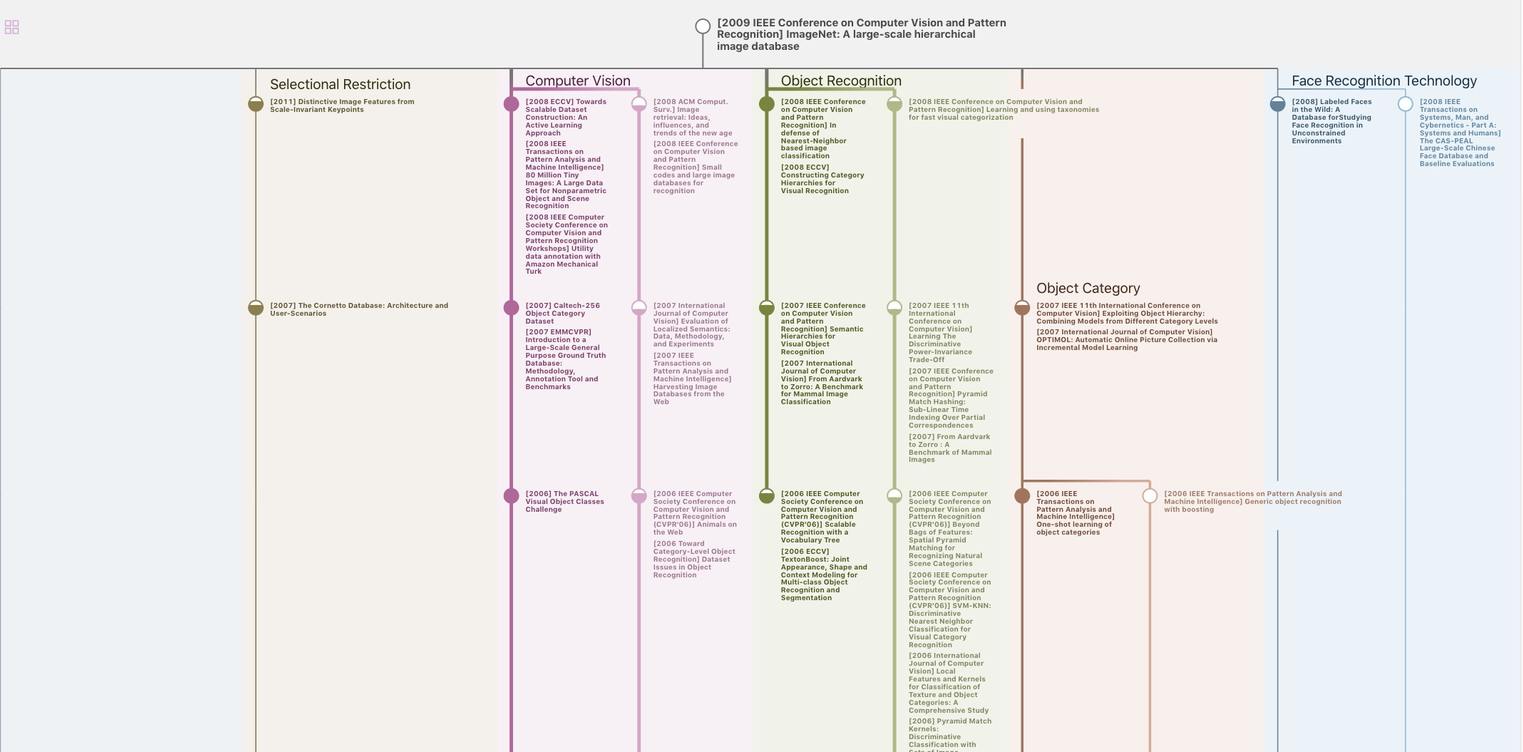
生成溯源树,研究论文发展脉络
Chat Paper
正在生成论文摘要