Conditional Random Fields Based On Weighted Feature Difference Potential For Remote Sensing Image Classification
ADVANCES IN INFORMATION AND COMMUNICATION, VOL 2(2020)
摘要
Image pixel-wise classification is an important challenge in the field of remote sensing. Some traditional methods, e.g., SVM and random forest, tend to ignore the contextual information, which may fail to process ambiguous samples. Conditional random fields (CRF) is an effective approach which eliminates semantic ambiguity via considering the relationship between adjacent pixels. In CRF model, the pairwise potential reflects the contextual relationship. However, the existed pairwise potential often equally treats each feature and does not highlight the roles of key features. In this work, we propose a CRF model with weighted feature difference pairwise potential which makes the key features play important roles. Thus, we can meticulously depict the contextual relationship. We further employ a max-margin method to optimize the CRF model. Experimental results on the hyper-spectral and multi-spectral remote sensing image datasets demonstrate that our approach has the better classification performance.
更多查看译文
关键词
Remote sensing, Image classification, Conditional random fields, Weighted feature difference potential
AI 理解论文
溯源树
样例
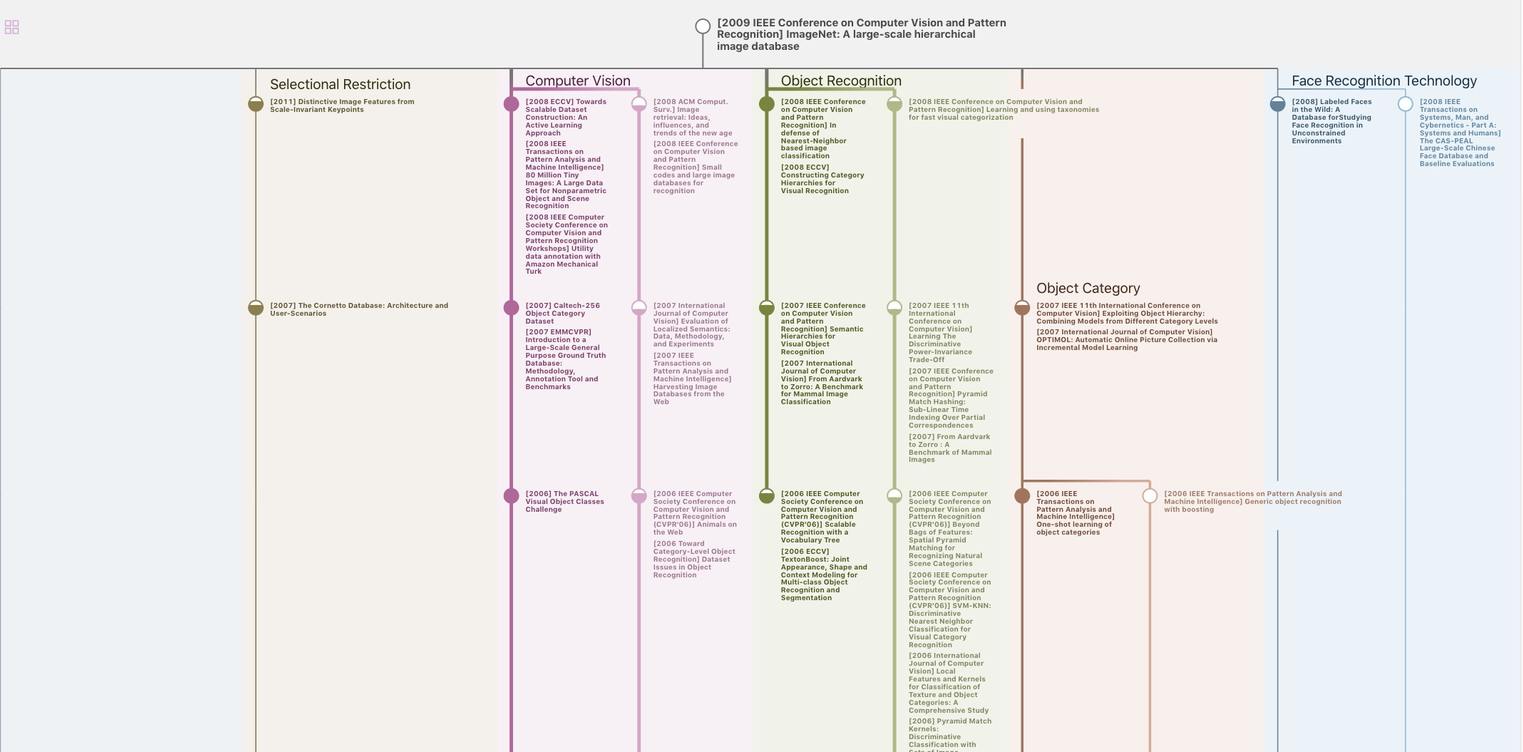
生成溯源树,研究论文发展脉络
Chat Paper
正在生成论文摘要