Clustering And Classification To Evaluate Data Reduction Via Johnson-Lindenstrauss Transform
ADVANCES IN INFORMATION AND COMMUNICATION, VOL 2(2020)
摘要
A dataset is a matrix X with n x d entries, where n is the number of observations and d is the number of variables (dimensions). Johnson and Lindenstrauss assert that a transformation exists to achieve a matrix with n x k entries, k << d, such that certain geometric properties of the original matrix are preserved. The property that we seek is that if we look at all pairs of points in matrix X, the distance between any two points should be the same within a given small acceptable level of distortion as the corresponding distance between the same two points in the reduced dataset. Does it follow that semantic content of the data is preserved in the transformation? We can answer in the affirmative that meaning in the original dataset was preserved in the reduced dataset. This was confirmed by comparison of clustering and classification results on the original and reduced datasets.
更多查看译文
关键词
Data reduction, High dimensional data, Clustering, Classification, Johnson-Lindenstrauss Transform
AI 理解论文
溯源树
样例
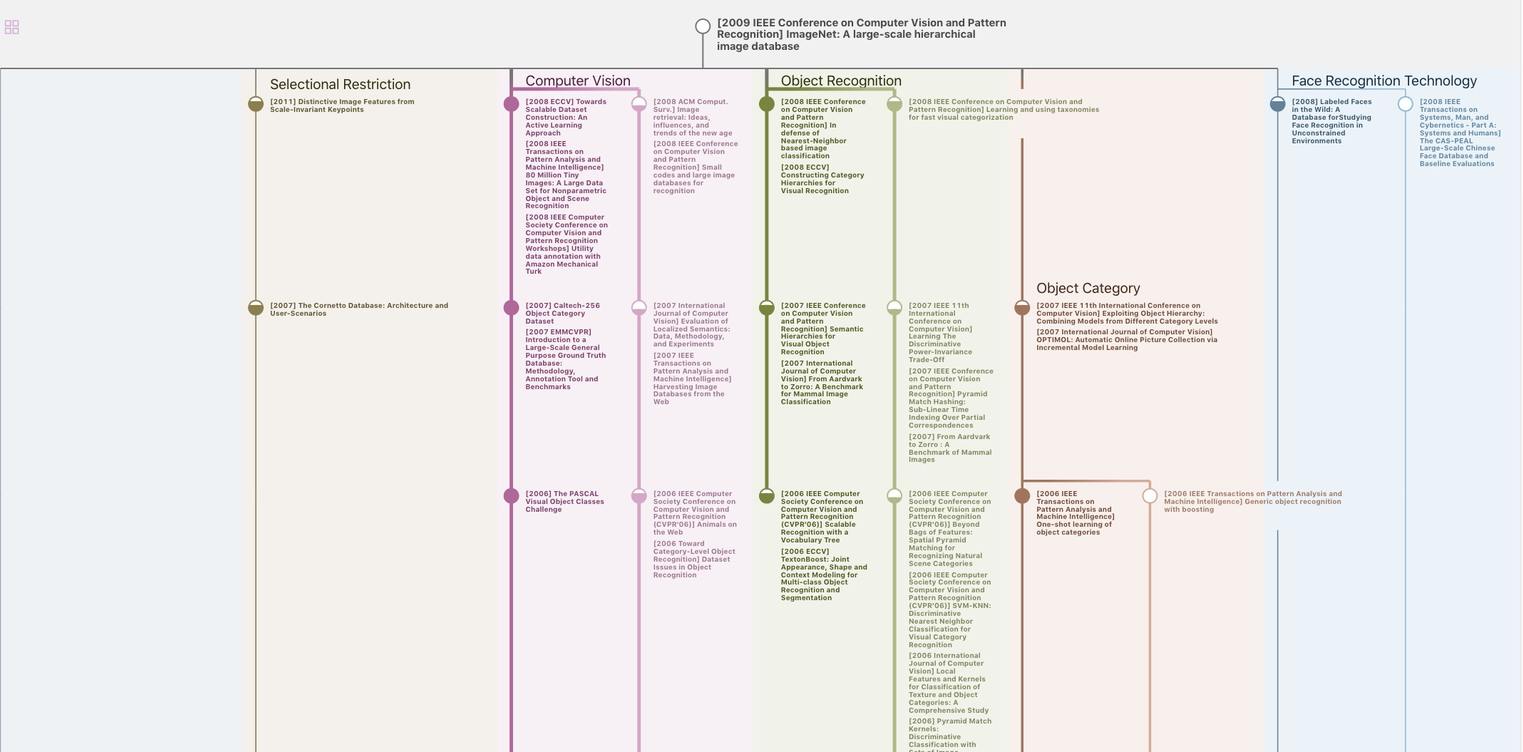
生成溯源树,研究论文发展脉络
Chat Paper
正在生成论文摘要