An Integrated Perception Pipeline For Robot Mission Execution In Unstructured Environments
ARTIFICIAL INTELLIGENCE AND MACHINE LEARNING FOR MULTI-DOMAIN OPERATIONS APPLICATIONS II(2020)
摘要
Visual perception has become core technology in autonomous robotics to identify and localize objects of interest to ensure successful and safe task execution. As part of the recently concluded Robotics Collaborative Technology Alliance (RCTA) program, a collaborative research effort among government, academic, and industry partners, a vision acquisition and processing pipeline was developed and demonstrated to support manned-unmanned teaming for Army relevant applications. The perception pipeline provided accurate and cohesive situational awareness to support autonomous robot capabilities for maneuver in dynamic and unstructured environments, collaborative human-robot mission planning and execution, and mobile manipulation. Development of the pipeline involved a) collecting domain specific data, b) curating ground truth annotations, e.g., bounding boxes, keypoints, c) re-training deep networks to obtain updated object detection and pose estimation models, and d) deploying and testing the trained models on ground robots. We discuss the process of delivering this perception pipeline under limited time and resource constraints due to lack of a priori knowledge of the operational environment. We focus on experiments conducted to optimize the models despite using data that was noisy and exhibited sparse examples for some object classes. Additionally, we discuss our augmentation techniques used to enhance the data set given skewed class distributions. These efforts highlight some initial work that directly relates to learning and updating visual perception systems quickly in the field under sudden environment or mission changes.
更多查看译文
关键词
robot visual perception, object detection, keypoint detection, learning from small data, operation in unstructured environments
AI 理解论文
溯源树
样例
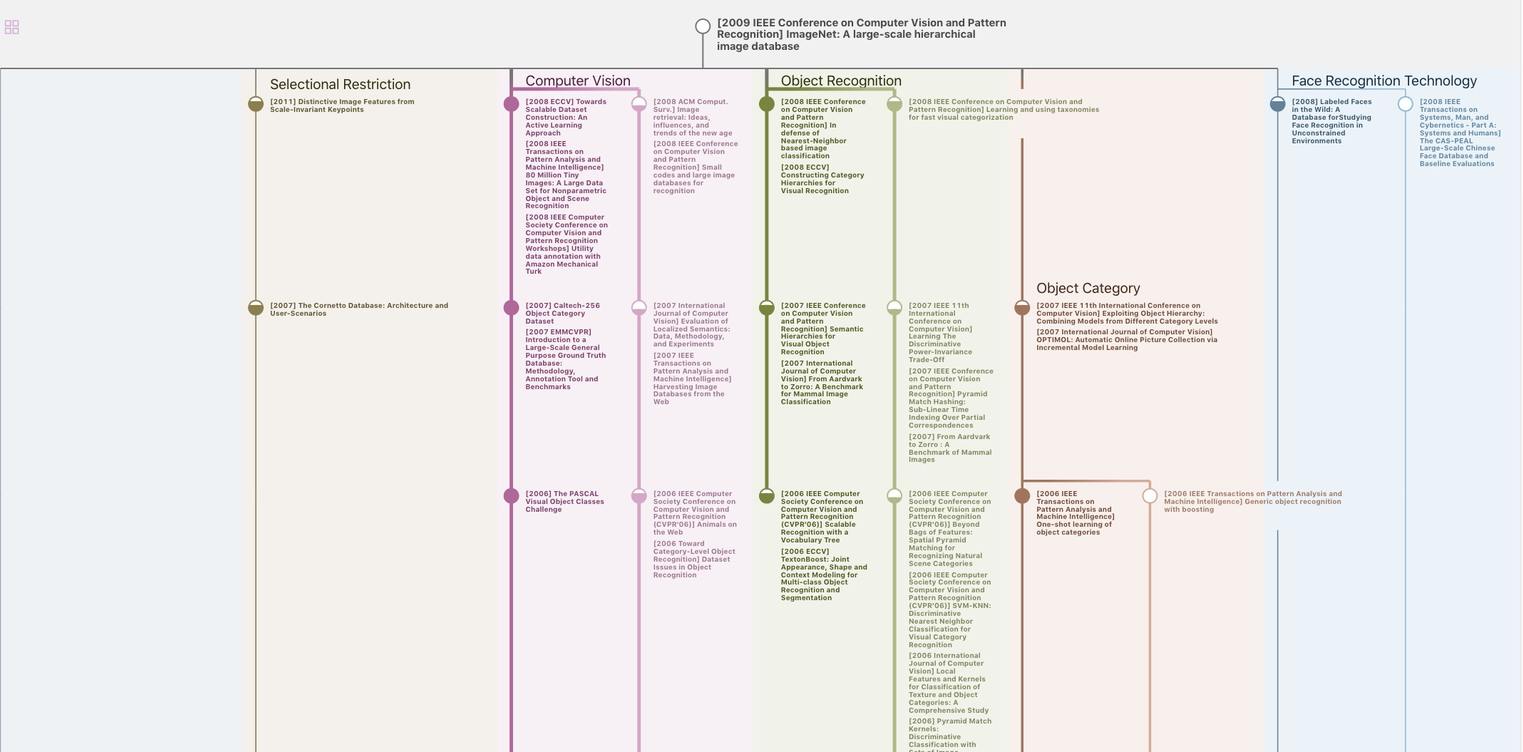
生成溯源树,研究论文发展脉络
Chat Paper
正在生成论文摘要