A Joint Cross-Modal Super-Resolution Approach For Vehicle Detection In Aerial Imagery
ARTIFICIAL INTELLIGENCE AND MACHINE LEARNING FOR MULTI-DOMAIN OPERATIONS APPLICATIONS II(2020)
摘要
Vehicle detection in aerial imagery is still an open research challenge although it has received some breakthroughs in the computer vision research community. Most of the existing state-of-the-art vehicle detection algorithms have ignored to consider some major factors which may have a great influence on the detection task. The low-resolution characteristic of aerial images is considered one of the major factors. Although the super-resolution technique can resolve this problem which learns a mapping between the low-resolution (LR) images and their corresponding high-resolution (HR) counterparts, however, the problem still remains when detection needs to take place at night or in a dark environment. Therefore, RGB-based detection can be another vital problem specifically for the detection task in a dark environment. For such environment infrared (IR) imaging becomes necessary which again may not be available during training an IR detector. To address these challenges, we propose a joint cross-modal and super-resolution framework based on the Generative Adversarial Network (GAN) for vehicle detection in aerial images. Our proposed joint network consists of two deep sub-networks. The first sub-network utilizes the GAN architecture to generate super-resolved (SR) images across two different domains (cross-domain translation). The second sub-network performs detection on these cross-domain translated and super-resolved images using one of the state-of-the-art object detectors i.e., You Only Look Once version 3 (YOLOv3). To evaluate the efficacy of our proposed model, we conduct several experiments on a publicly available Vehicle Detection in Aerial Imagery (VEDAI) dataset. We further compare our proposed network with state-of-the-art image generation methods to show the adequacy of our model.
更多查看译文
关键词
Super-resolved (SR), Infrared (IR), Generative Adversarial Network (GAN), You Only Look Once-version3 (YOLOv3)
AI 理解论文
溯源树
样例
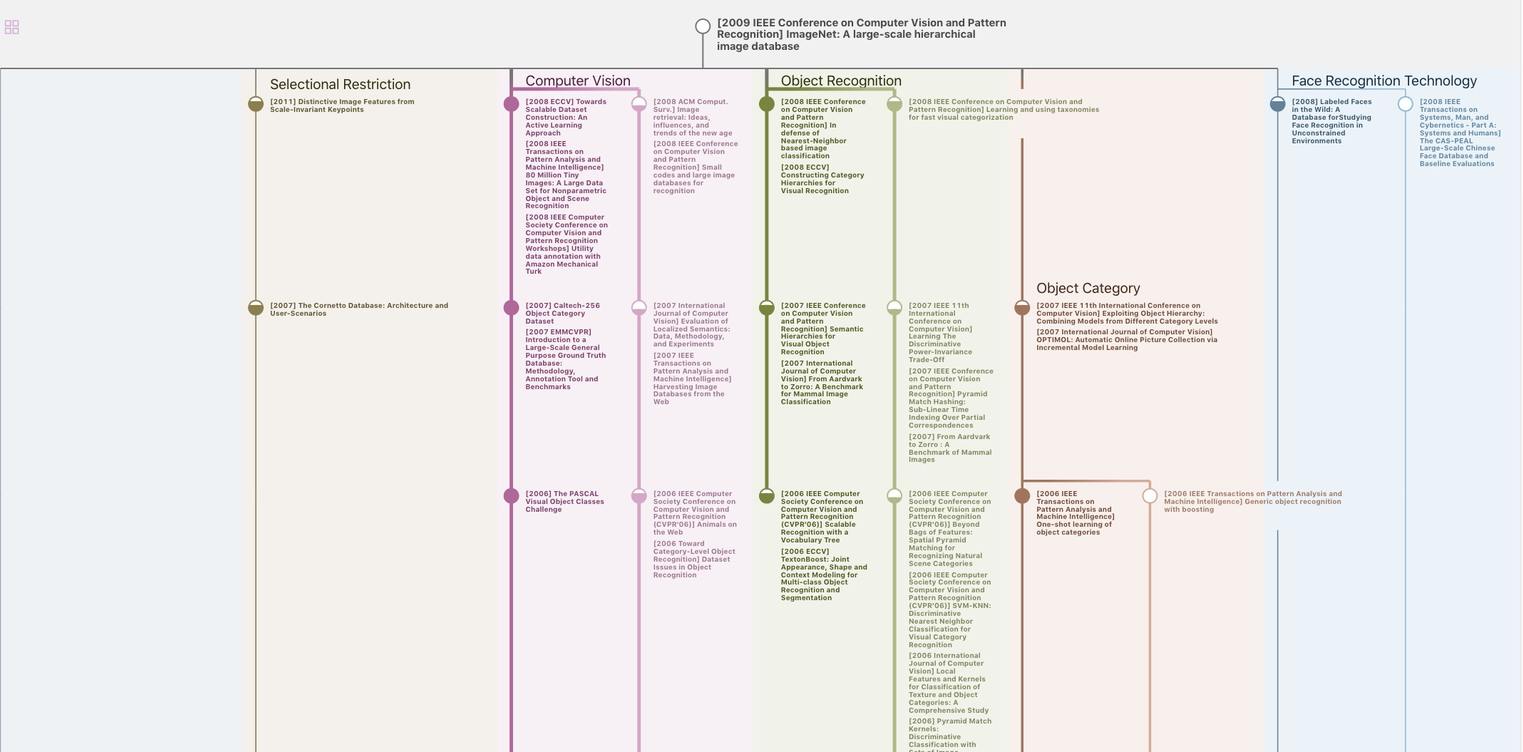
生成溯源树,研究论文发展脉络
Chat Paper
正在生成论文摘要