Extending Alpha-Expansion To A Larger Set Of Regularization Functions
2015 IEEE INTERNATIONAL CONFERENCE ON IMAGE PROCESSING (ICIP)(2015)
摘要
Many problems of image processing lead to the minimization of an energy, which is a function of one or several given images, with respect to a binary or multi-label image. When this energy is made of unary data terms and of pairwise regularization terms, and when the pairwise regularization term is a metric, the multi-label energy can be minimized quite rapidly, using the so-called alpha-expansion algorithm. alpha-expansion consists in decomposing the multi-label optimization into a series of binary sub-problems called move. Depending on the chosen decomposition, a different condition on the regularization term applies. The metric condition for alpha-expansion move is rather restrictive. In many cases, the statistical model of the problem leads to an energy which is not a metric. Based on the enlightening article [1], we derive another condition for beta-jump move. Finally, we propose an alternated scheme which can be used even if the energy fulfills neither the alpha-expansion nor beta-jump condition. The proposed scheme applies to a much larger class of regularization functions, compared to alpha expansion. This opens many possibilities of improvements on diverse image processing problems. We illustrate the advantages of the proposed optimization scheme on the image noise reduction problem.
更多查看译文
关键词
Minimization,Discrete optimization,Regularization,Markov Random Field,alpha-expansion,Graphcuts,Denoising,Noise reduction
AI 理解论文
溯源树
样例
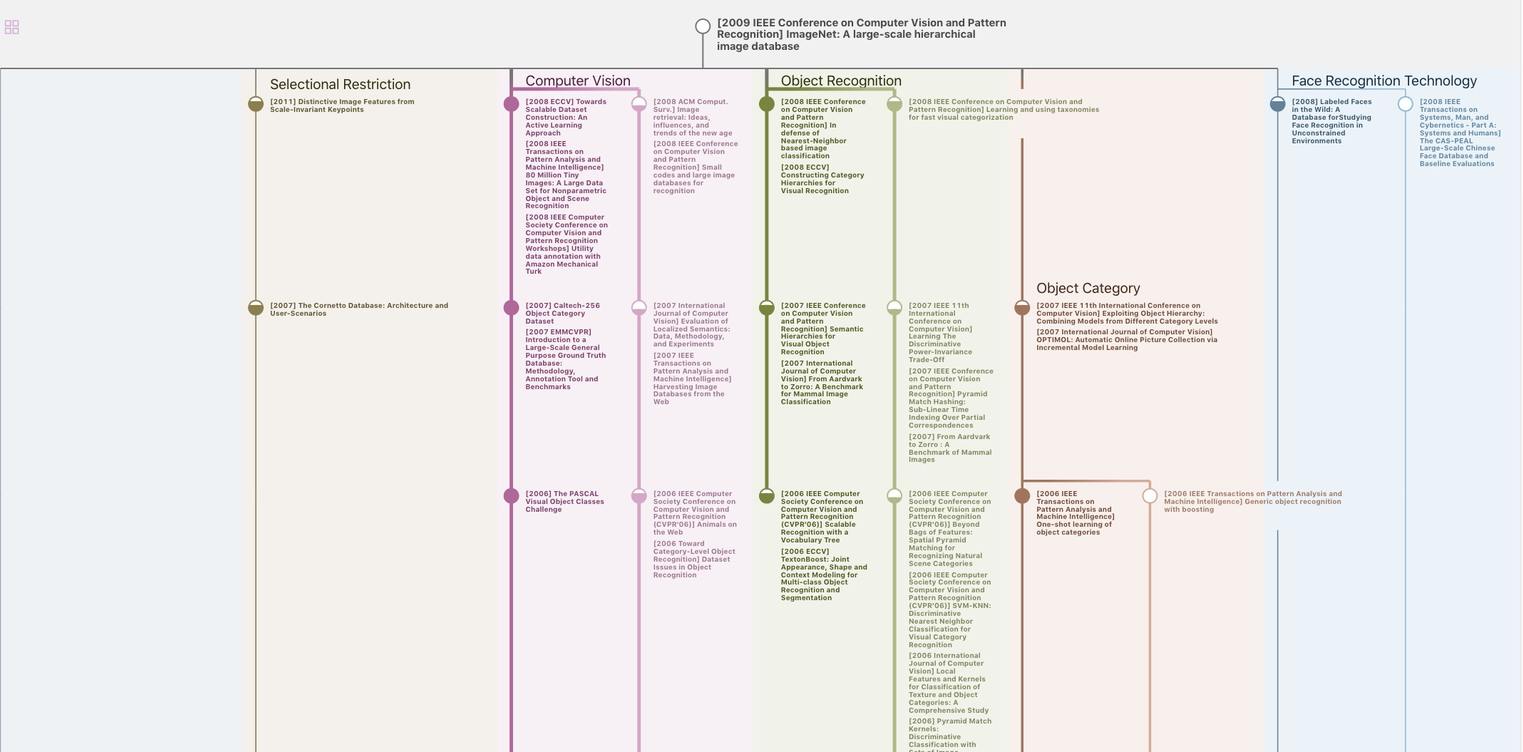
生成溯源树,研究论文发展脉络
Chat Paper
正在生成论文摘要