Towards A More Stable Traffic Flow Performance: Applying And Calibrating The Intelligent Driver Model
TRAFFIC AND GRANULAR FLOW '17(2019)
摘要
The surrounding conditions (e.g., weather condition) under which a roadway segment operates may affect its performance, impairing its capacity level and consequently resulting into the formulation and propagation of congestion. In this study, we translated the traffic data from continuous count station (CCS, administrated by Virginia Department of Transportation-VDOT) in Northern Virginia into fundamental diagrams (I-Ds). By overlapping such data with weather data (available on Weather Channel), two travel condition types were defined, namely normal commute condition and inclement weather condition. The conditional I-Ds indicate a significant decrease in capacity under inclement weather condition compared to normal commute condition. To reveal the underlying factors leading to such capacity decrease, we obtained the parameters from intelligent driver model (IDM, as the car-following algorithm) and minimizing overall braking induced by lane changes (MOBIL, as the lane-changing algorithm), trained with real traffic data to simulate multilane behavior and weather conditions, adjusted to match the characteristics in the capacity breakdown situations. It is found that reaction time will affect the capacity level at a location of roadway and thus the congestion formulation while the lane-changing will influence the traveling of shock waves over a length of roadway and thus the congestion propagation.
更多查看译文
关键词
stable traffic flow performance,intelligent driver model
AI 理解论文
溯源树
样例
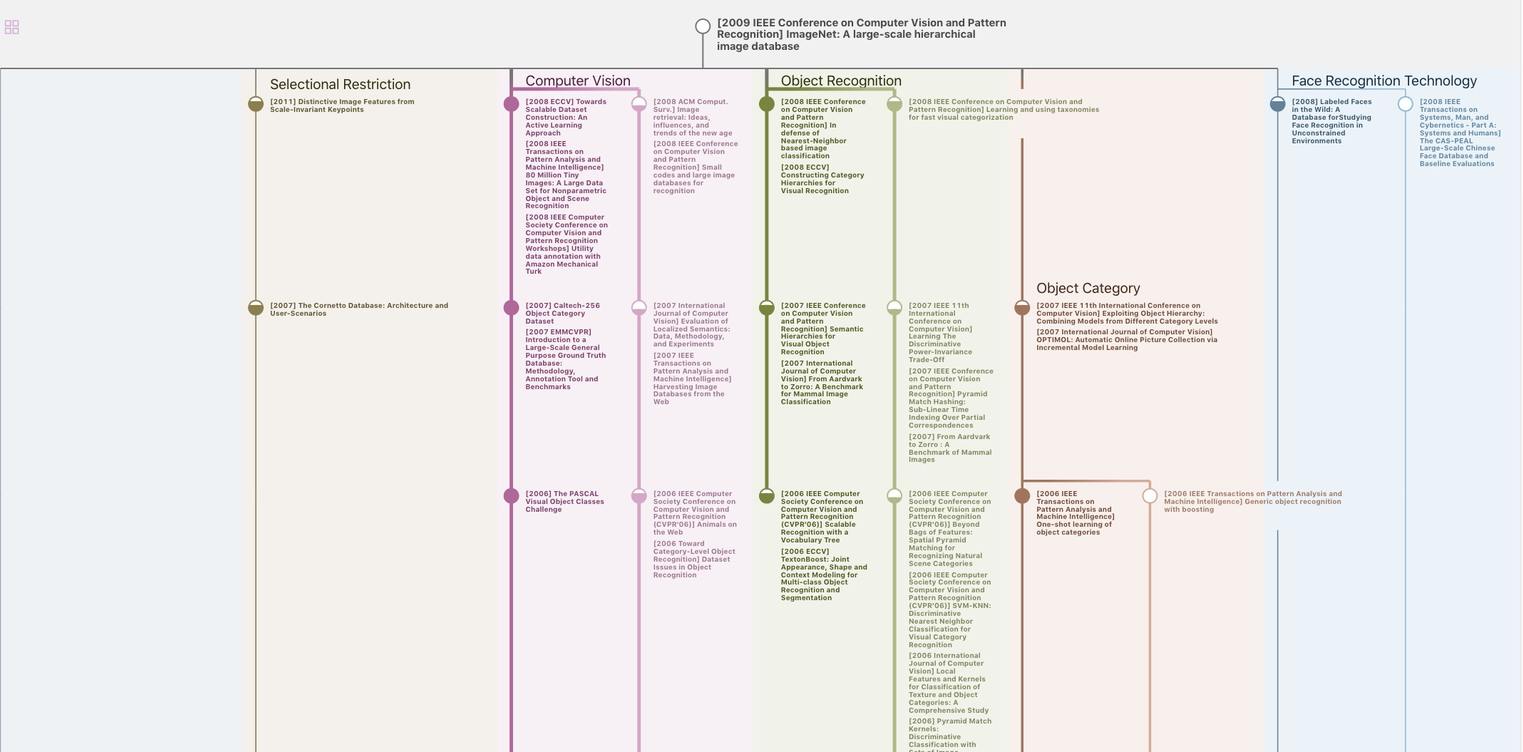
生成溯源树,研究论文发展脉络
Chat Paper
正在生成论文摘要