Classification of Pre-ictal and Interictal Periods Based on EEG Frequency Features in Epilepsy
2016 32nd Southern Biomedical Engineering Conference (SBEC)(2016)
摘要
A seizure prediction system has the potential to significantly help patients with epilepsy. For a seizure forecasting system to work effectively, computational algorithms must reliably identify periods with high probability of seizure occurrence. We herein report results of a classification approach based on machine learning of EEG features in the frequency domain and aimed at differentiating between pre-ictal (close to seizure onsets) and interictal (far away from seizures onset) periods in long-term intracranial EEG recordings from the brain of 5 epileptic dogs. Evaluation of performance by the area under the ROC curve ranged from 0.84 to 0.96 in four dogs, while for the fifth dog was considerably less (0.55), resulting to a global value of 0.87 across dogs. These results offer supporting evidence that seizures may be predictable with a proper analysis of the EEG.
更多查看译文
关键词
RUSTON
AI 理解论文
溯源树
样例
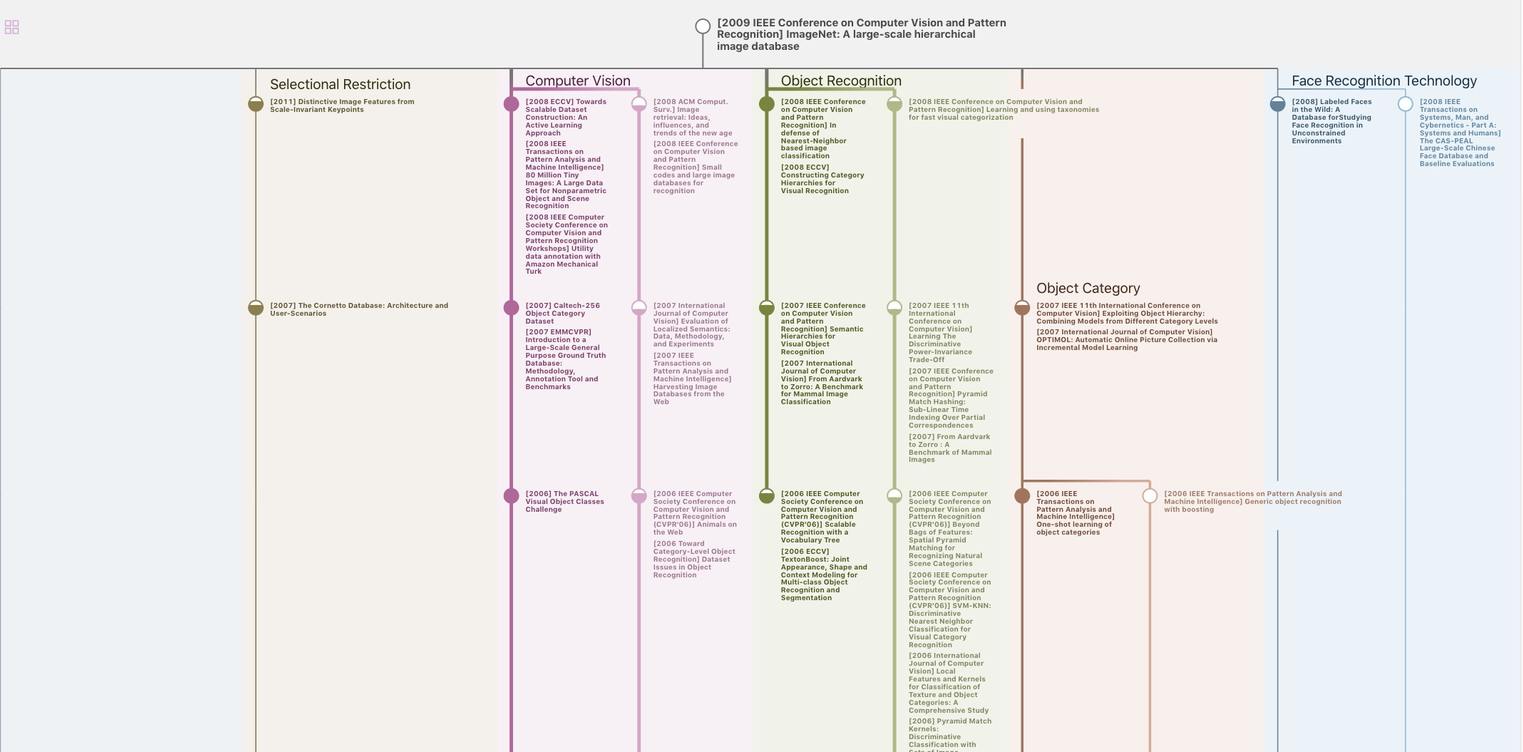
生成溯源树,研究论文发展脉络
Chat Paper
正在生成论文摘要