Metis: Robustly Optimizing Tail Latencies Of Cloud Systems
PROCEEDINGS OF THE 2018 USENIX ANNUAL TECHNICAL CONFERENCE(2018)
摘要
Tuning configurations is essential for operating modern cloud systems, but the difficulty arises from the cloud system's diverse workloads, large system scale, and vast parameter space. Building on previous space exploration efforts of searching for the optimal system configuration, we argue that cloud systems introduce challenges to the robustness of auto-tuning. First, performance metrics such as tail latencies can be sensitive to non-trivial noises. Second, while treating target systems as a black box promotes applicability, it complicates the goal of balancing exploitation and exploration. To this end, Metis is an auto-tuning service used by several Microsoft services, and it implements customized Bayesian optimization to robustly improve auto-tuning: (1) diagnostic models to find potential data outliers for re-sampling, and (2) a mixture of acquisition functions to balance exploitation, exploration and re-sampling. This paper uses Bing Ads key-value store clusters as the running example - compared to weeks of manual tuning by human experts, production results show that Metis reduces the overall tuning time by 98.41%, while reducing the 99-percentile latency by another 3.43%.
更多查看译文
AI 理解论文
溯源树
样例
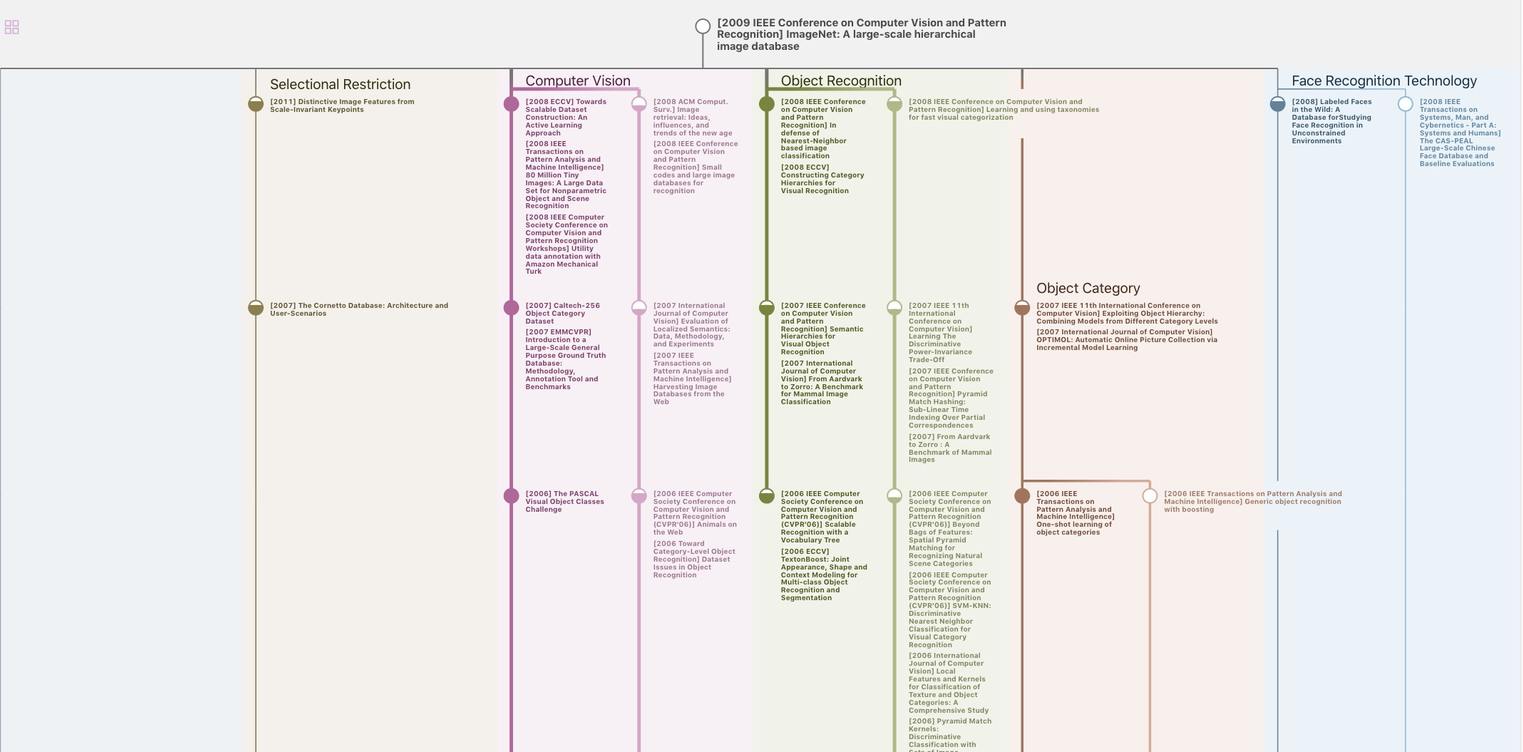
生成溯源树,研究论文发展脉络
Chat Paper
正在生成论文摘要