Quantitative Analysis of Petroleum Hydrocarbon Contaminated Soils using Spectroscopy, Spectral Unmixing and Deep Neural Networks
IMAGE AND SIGNAL PROCESSING FOR REMOTE SENSING XXIV(2018)
摘要
Oil spill can be described as a global issue, which causes serious concern to human life and the environment, therefore early identification and remedial measures taken at an early stage is very important. Spectral Unmixing is the process of identifying the constituent spectra of a mixed pixel referred to as endmembers and computing the corresponding proportions or abundances within each pixel in a given image. Many spectral unmixing methods have being proposed in the literature based on linear or nonlinear models. Deep neural networks allow computational models that are composed of multiple processing layers to learn representations of data with multiple levels of abstractions. Deep neural networks have shown excellent performance on various task on image processing with better accuracy compared to shallow learning networks and are increasingly gaining popularity with Hyperspectral imaging. Here we propose to use deep neural network to quantify different Hydrocarbon (HCs) substances in sandy clay loam soil type. Hyperspectral data sets have been acquired using mixtures of different HCs with the soil type. Vertex Component Analysis (VCA) algorithm was used to identify the endmembers and deep neural network was used to predict the quantity of each endmember. Experimental result shows the effectiveness of the proposed method with high accuracy.
更多查看译文
关键词
Spectroscopy, Hydrocarbons, Spectral unmixing, Hyperspectral imaging, Deep neural networks
AI 理解论文
溯源树
样例
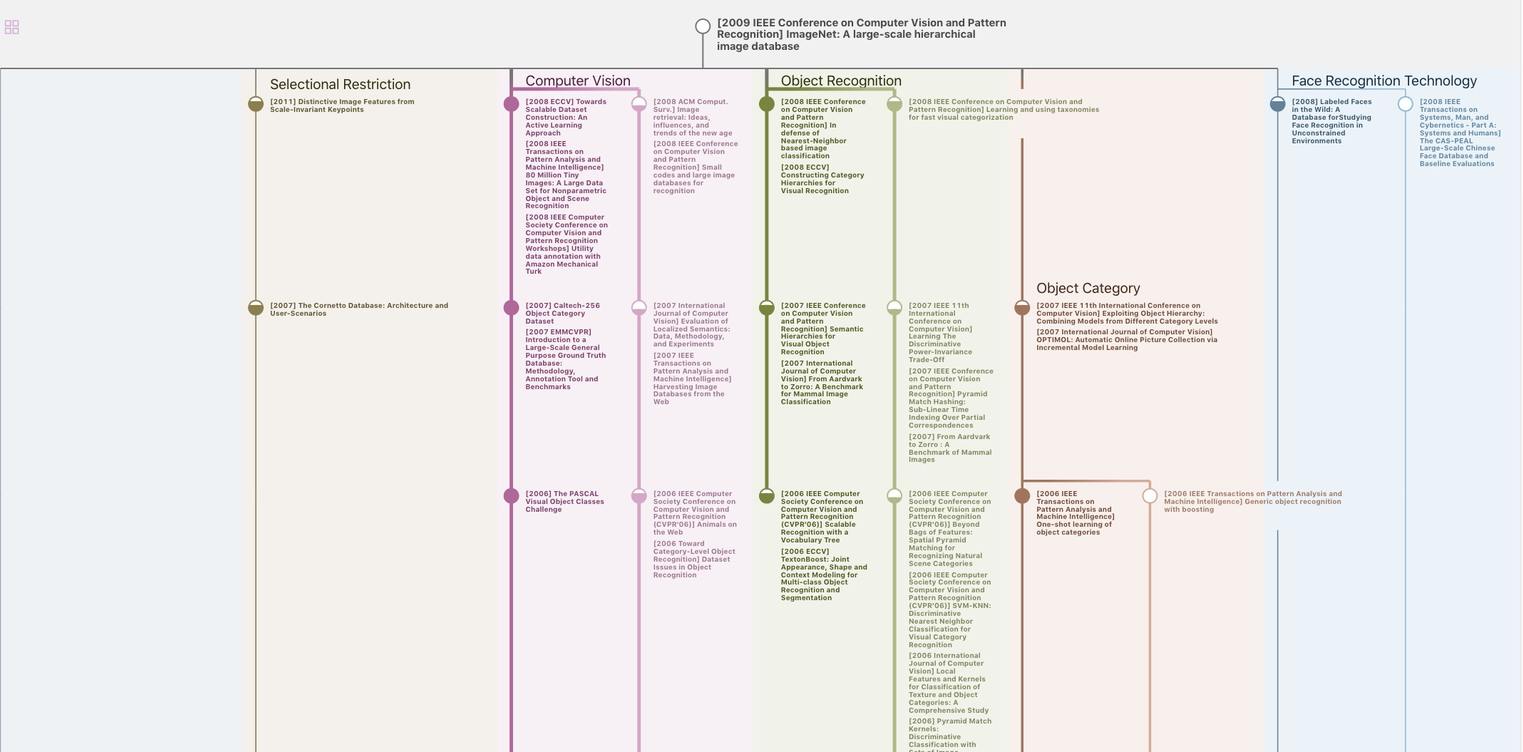
生成溯源树,研究论文发展脉络
Chat Paper
正在生成论文摘要