Quality Prediction In Injection Molding Neural Networks Geometric Dimension Prediction On Raw Signals And Thermographic Images
2017 IEEE INTERNATIONAL CONFERENCE ON COMPUTATIONAL INTELLIGENCE AND VIRTUAL ENVIRONMENTS FOR MEASUREMENT SYSTEMS AND APPLICATIONS (CIVEMSA)(2017)
摘要
Injection molded part quality can be improved by precise process adjustment, which could rely on in-situ measurements of part quality. Geometrical and appearance quality (visually and sensory) requirements are increasing. However, direct measurement is often not feasible industrially. Therefore, process control must rely on a prediction of parts quality attributes. This study compares prediction performances of diverse neural networks architectures with "classical" regression algorithms. Dataset comes from inline industrial measurements. Regression was performed on 97 scalar statistical features extracted from multiple acquisitions sources: thermographic images and analog signals. Haralick features were extracted. Convolutional Neural Networks were trained on thermographic images and Long Short Term Memory networks were trained on raw signals. Although the dataset was small, neural networks show better predictions scores than other regression algorithms.
更多查看译文
关键词
injection molding, quality prediction, thermography, Convolutional Neural Networks, LSTM
AI 理解论文
溯源树
样例
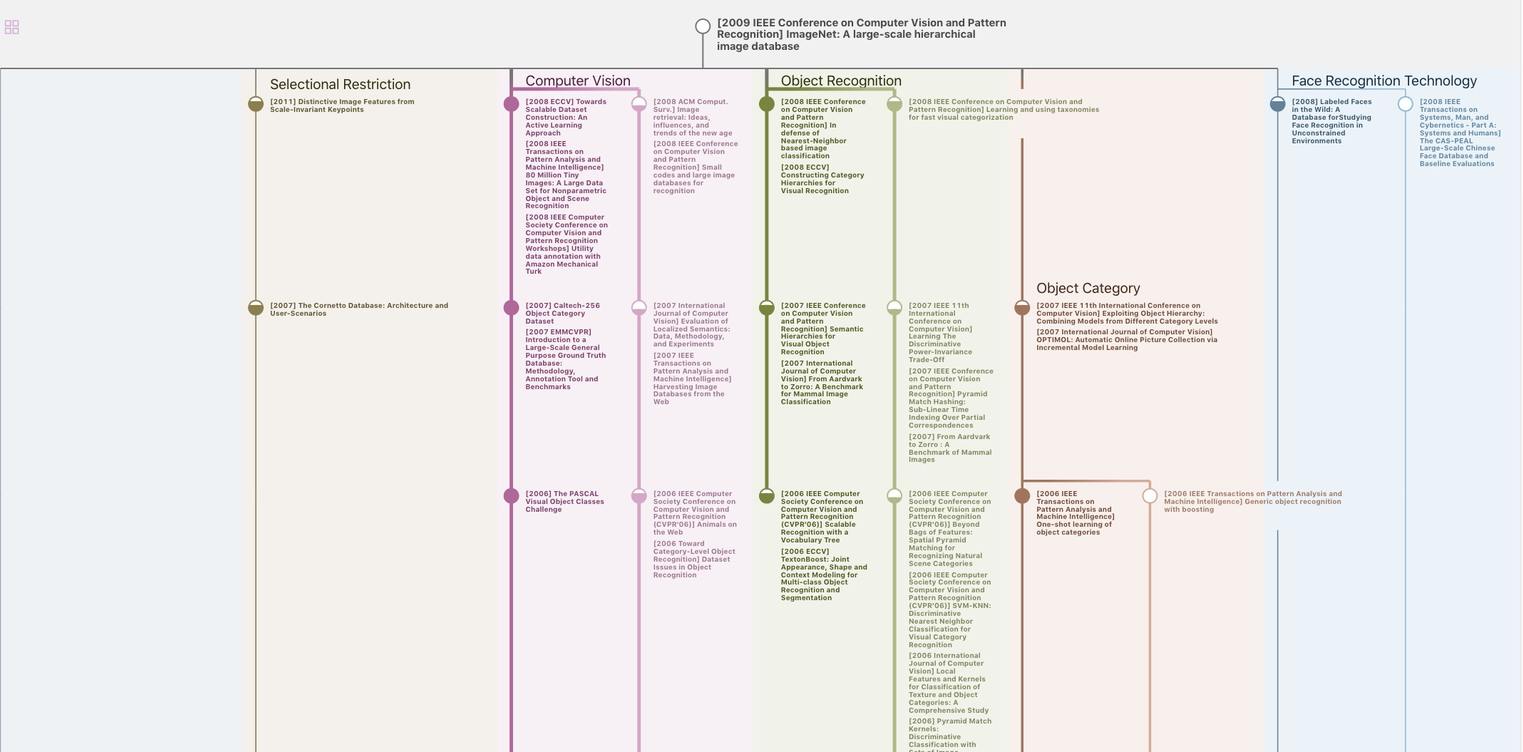
生成溯源树,研究论文发展脉络
Chat Paper
正在生成论文摘要