Performance Evaluation Of Convolutional Neural Network At Hyperspectral And Multispectral Resolution For Classification
IMAGE AND SIGNAL PROCESSING FOR REMOTE SENSING XXV(2019)
摘要
Convolutional Neural Network (CNN) has established as an effective deep learning model for hyperspectral image classification by considering both spectral and spatial information. In this study, the performance of two-dimensional (2D) CNN architecture is evaluated at hyperspectral and multispectral resolution. Two types of multispectral data are analyzed viz., original and transformed multispectral data. Hyperspectral bands are transformed to spectral resolution of multispectral bands by averaging the reflectances of specific hyperspectral narrow bands which are falling within the spectral ranges of multispectral bands. The well-known Pavia University dataset and a new dataset of Pear orchard are investigated in this study. In case of Pear orchard dataset, classification is performed with both types of multispectral data. All the experiments are carried out with the same 2D CNN architecture. In case of Pavia University dataset, hyperspectral and transformed multispectral data achieve OA(%) of 94.29 +/- 1.28 and 94.27 +/- 2.01 respectively considering 20% samples as training. In case of Pear orchard dataset, hyperspectral, multispectral and transformed multispectral data achieve OA(%) of 91.59 +/- 0.89, 88.65 +/- 1.35, and 93.24 +/- 0.16 respectively considering 20% samples as training. It is evident that transformed multispectral data, which comprises of inherent hyperspectral information, provides similar or better performance compared to hyperspectral data. Further, with the use of 3D CNN architecture, classification performance improves in case of Pavia University dataset, whereas it remains statistically similar in case of Pear orchard dataset. The present promising results illustrates the performance of CNN even in small dataset which is comparable to several published state-of-the art results on the same dataset.
更多查看译文
关键词
Convolutional neural network, deep learning, hyperspectral data, multispectral data, spectral-spatial classification, 2D CNN, 3D CNN
AI 理解论文
溯源树
样例
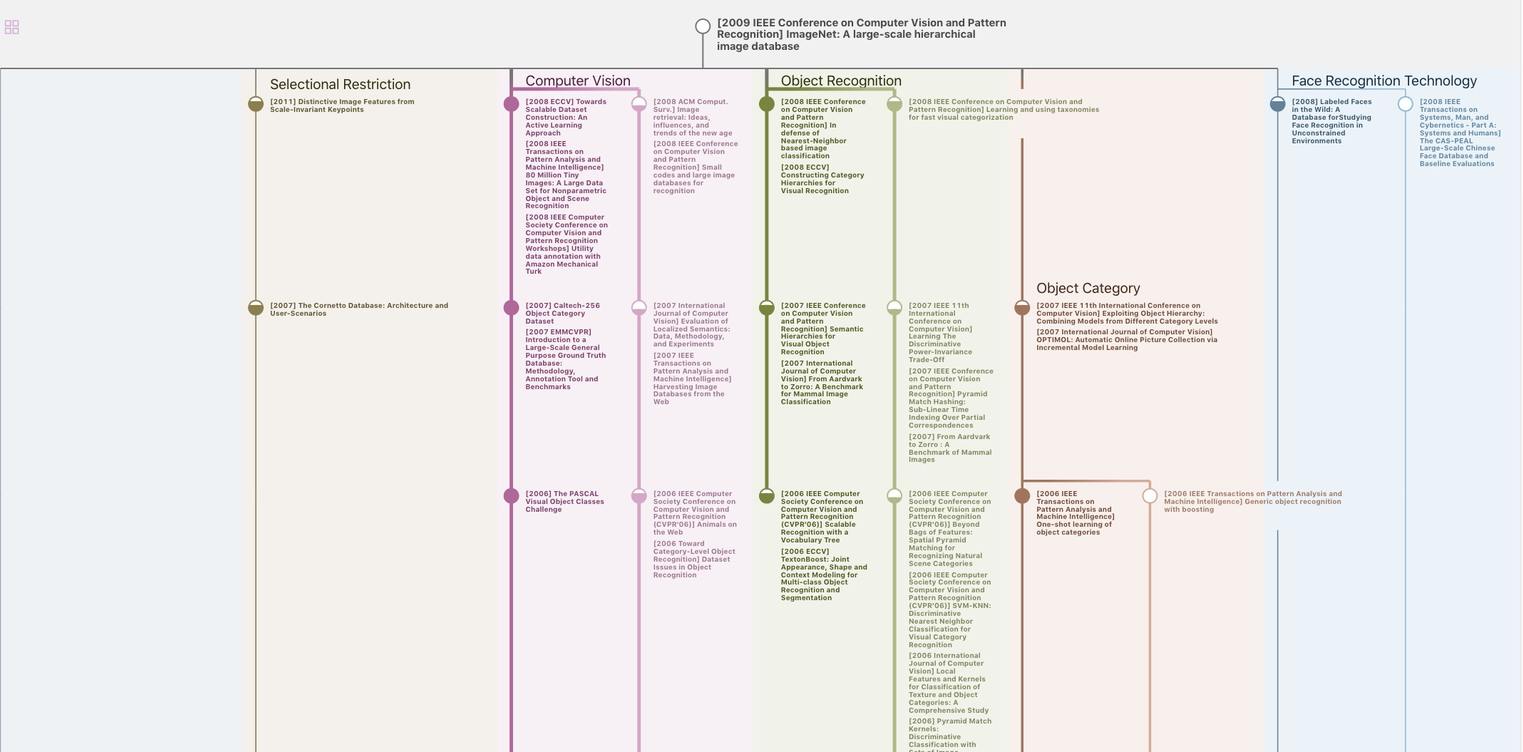
生成溯源树,研究论文发展脉络
Chat Paper
正在生成论文摘要