Bidirectional internal memory gate recurrent neural networks for spoken language understanding
International Journal of Speech Technology(2020)
摘要
Recurrent neural networks have encountered a wide success in different domains due to their high capability to code short- and long-term dependencies between basic features of a sequence. Different RNN units have been proposed to well manage the term dependencies with an efficient algorithm that requires few basic operations to reduce the processing time needed to learn the model. Among these units, the internal memory gate (IMG) have produce efficient accuracies faster than LSTM and GRU during a SLU task. This paper presents the bidirectional internal memory gate recurrent neural network (BIMG) that codes short- and long-term dependencies in forward and backward directions. Indeed, the BIMG is composed with IMG cells made of an unique gate managing short- and long-term dependencies by combining the advantages of the LSTM, GRU (short- and long-term dependencies) and the leaky unit (LU) (fast learning). The effectiveness and the robustness of the proposed BIMG-RNN is evaluated during a theme identification task of telephone conversations. The experiments show that BIMG reaches better accuracies than BGRU and BLSTM with a gain of 1.1 and a gain of 2.1 with IMG model. Moreover, BIMG requires less processing time than BGRU and BLSTM with a gain of 12% and 35% respectively.
更多查看译文
关键词
Bidirectional recurrent neural network, Internal memory gate, Spoken language understanding
AI 理解论文
溯源树
样例
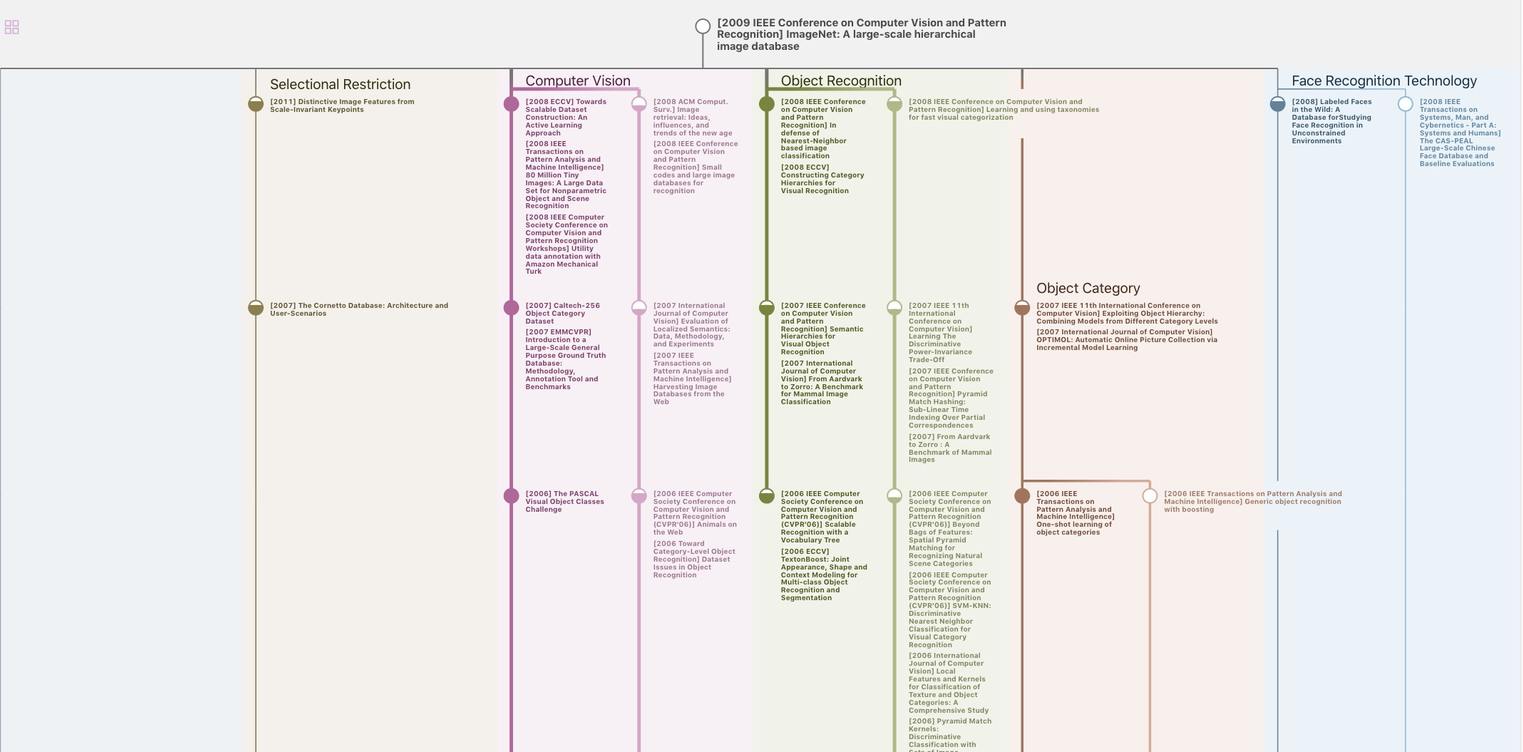
生成溯源树,研究论文发展脉络
Chat Paper
正在生成论文摘要