Quality Control Framework For Large Mr Datasets: Automated Approaches To Outlier Detection
XXVI BRAZILIAN CONGRESS ON BIOMEDICAL ENGINEERING, CBEB 2018, VOL. 2(2019)
摘要
Magnetic resonance (MR) imaging has been largely used as a diagnostic imaging modality. Especially in brain image analyses, MR allows the detection of subtle abnormalities, aiding in the patient diagnosis and follow-up. Driven by improved acquisition techniques, the amount of MR data to be processed and analyzed have risen exponentially. So too has its variability, particularly in multi-center studies which potentially include images acquired at different centers or using different acquisition sequences and parameters. Methods that aim to automatically evaluate quality in these large datasets are required to assure data correctness and completeness, and also to identify image artifacts and potentially patient abnormalities. We propose a quality control framework to detect prospective outliers sets in brain MR imaging datasets that is based on imaging features, such as image contrast, intensity and texture. The framework allows the selection of individual or the combination of features, over the entire database or over a subset data based on metadata information, such as gender or an age range. Experiments have shown that the proposed framework is capable of detecting imaging datasets with artefacts, such as motion, and also brain abnormalities, such as lesions.
更多查看译文
关键词
Magnetic resonance (MR) imaging, Quality control (QC), Outlier detection, Imaging features
AI 理解论文
溯源树
样例
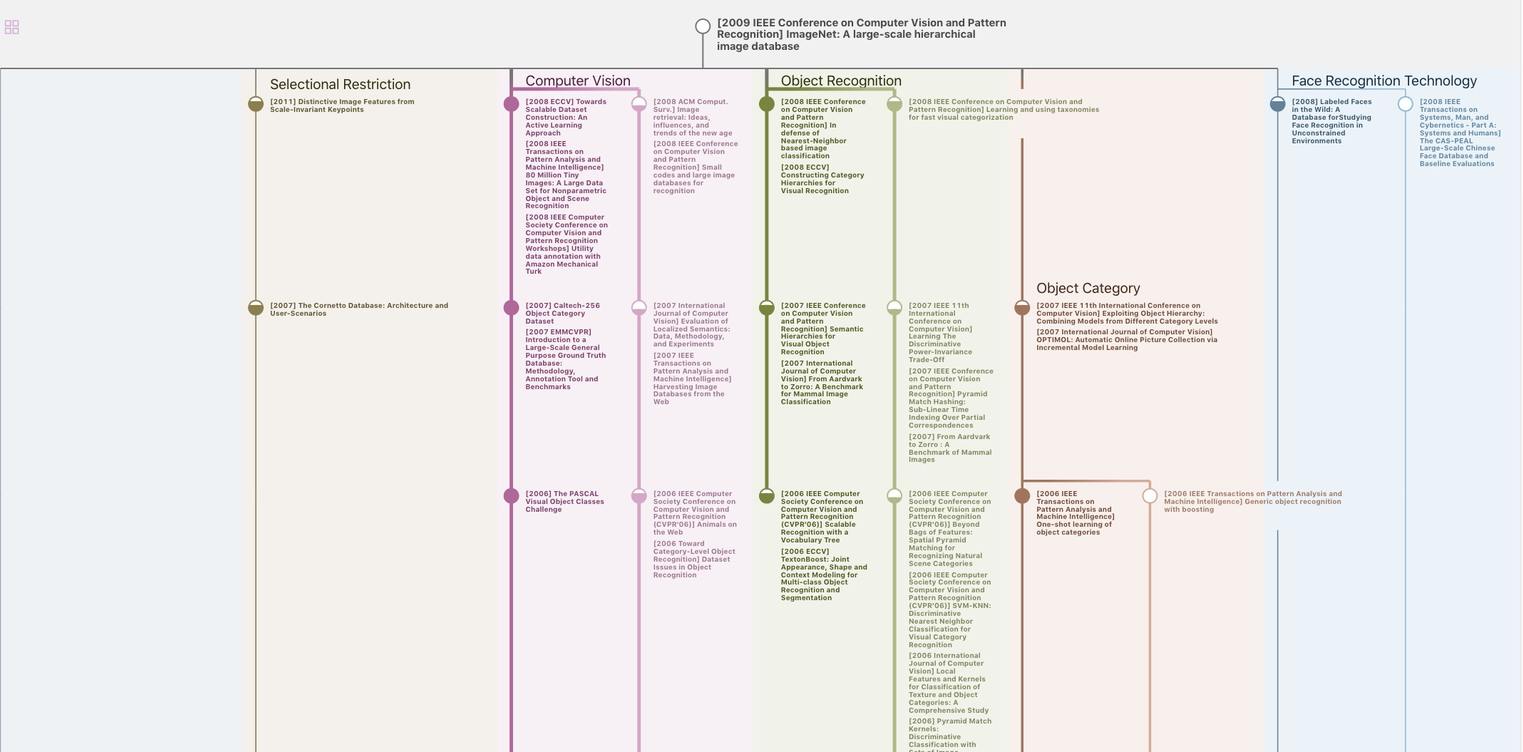
生成溯源树,研究论文发展脉络
Chat Paper
正在生成论文摘要