Personalized Tumor Growth Prediction Using Multiscale Modeling
JOURNAL OF BASIC AND CLINICAL HEALTH SCIENCES(2020)
摘要
Purpose: Cancer is one of the most complex phenomena in biology and medicine. Extensive attempts have been made to work around this complexity. In this study, we try to take a selective approach; not modeling each particular facet in detail but rather only the pertinent and essential parts of the tumor system are simulated and followed by optimization, revealing specific traits. This leads us to a pellucid personalized model which is noteworthy as it closely approximates existing experimental results.Methods: In the present study, a hybrid modeling approach which consists of cellular automata for discrete cell state representation and diffusion equations to calculate distribution of relevant substances in the tumor microenvironment is favored. Moreover, naive Bayesian decision making with weighted stochastic equations and a Bayesian network to model the temporal order of mutations is presented. The model is personalized according to the evidence using Markov Chain Monte Carlo. To validate the tumor model, a data set belonging to the A549 cell line is used. The data represents the growth of a tumor for 30 days. We optimize the coefficients of the stochastic decision-making equations using the first half of the timeline.Results: Simulation results of the developed model are promising with their low error margin (all correlation coefficients are over 0.8 under different microenvironment conditions) and simulated growth data is in line with laboratory results (r=0.97, p<0.01).Conclusions: Our approach of using simulated annealing for parameter estimation and the subsequent validation of the prediction with in-vitro tumor growth data are, to our knowledge, is novel.
更多查看译文
关键词
neoplasms, patient-specific modeling, adenocarcinoma of lung, precision medicine
AI 理解论文
溯源树
样例
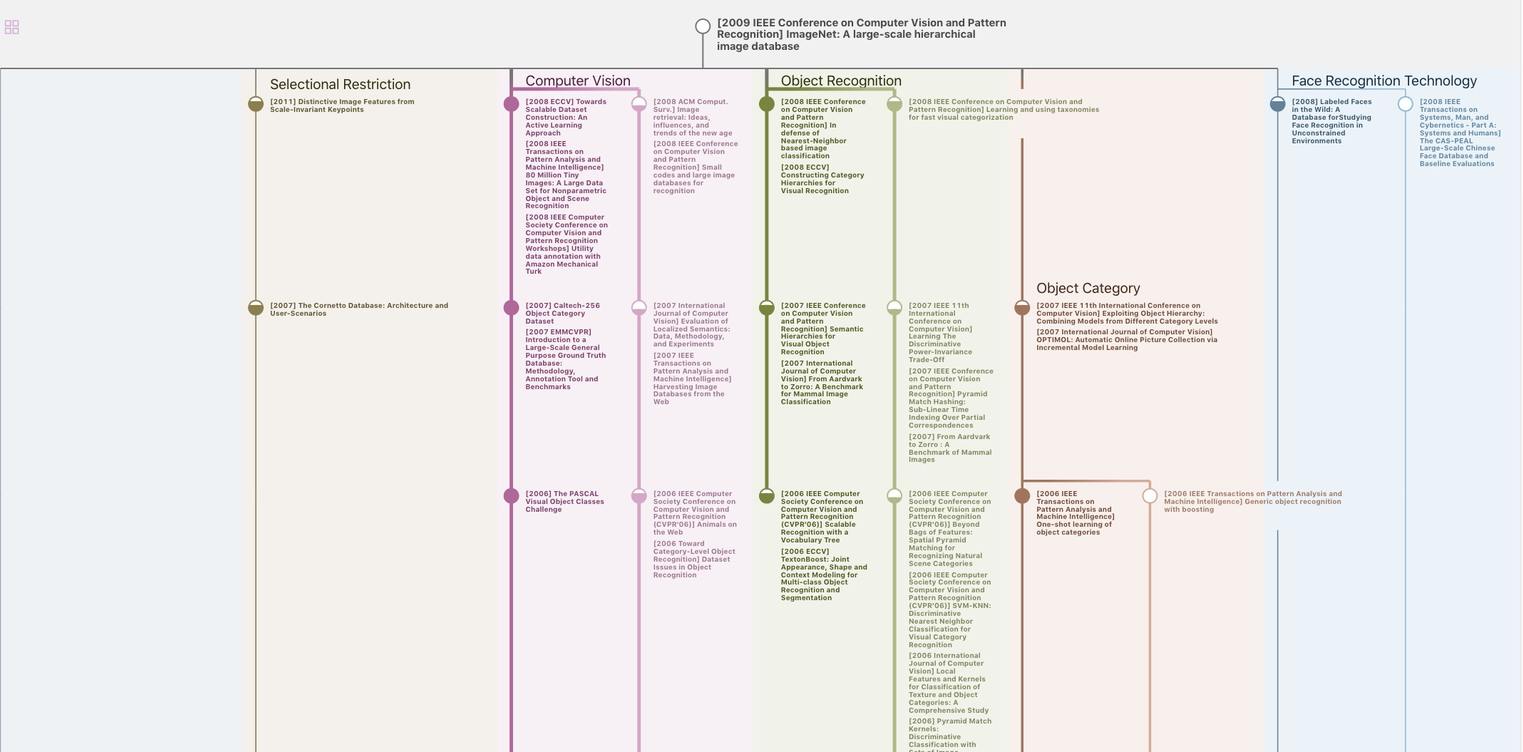
生成溯源树,研究论文发展脉络
Chat Paper
正在生成论文摘要