The Evaluation Of Word Embedding Models And Deep Learning Algorithms For Turkish Text Classification
2019 4TH INTERNATIONAL CONFERENCE ON COMPUTER SCIENCE AND ENGINEERING (UBMK)(2019)
摘要
The use of word embedding models and deep learning algorithms are currently the most common and popular trends to enhance the overall performance of a text classification/categorization system. Word embedding models are vectors that provide a mapping of words with similar meaning to own a similar representation which is learned from a corpus. Deep learning algorithms successful produce more successful results in many areas of their applications when they are compared to the conventional machine learning algorithms. In this study, three different word embedding models Word2Vec, Glove, and FastText are employed fur word representation. Instead of using conventional classification algorithms, three different deep learning architectures Recurrent Neural Networks (RNN), Long Short Term Memory Networks (LSTM) and Convolutional Neural Networks (CNN) are used for classification task by performing experiments on collections of different Turkish documents. Experimental results show that the usage of deep learning algorithms together with word embedding models advances the performance of text classification systems.
更多查看译文
关键词
Word2Vec, Glove, FastText, recurrent neural networks, long short term memory, convolutional neural networks, text categorization
AI 理解论文
溯源树
样例
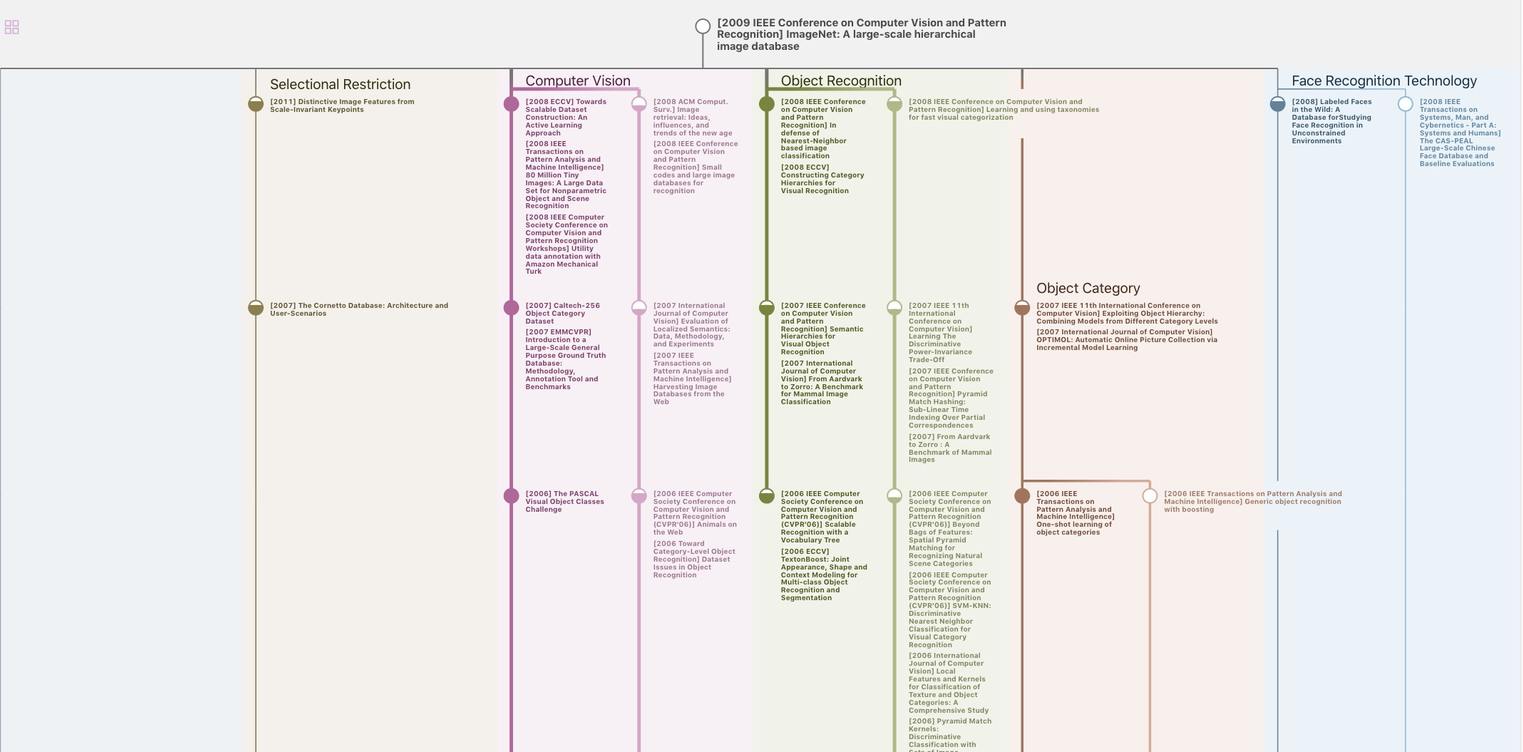
生成溯源树,研究论文发展脉络
Chat Paper
正在生成论文摘要