Meta BCI : Hippocampus-striatum network inspired architecture towards flexible BCI
2018 6th International Conference on Brain-Computer Interface (BCI)(2018)
摘要
Classifying neural signals is a crucial step in the brain-computer interface (BCI). Although Deep Neural Network (DNN) has been shown to be surprisingly good at classification, DNN suffers from long training time and catastrophic forgetting. Catastrophic forgetting refers to a phenomenon in which a DNN tends to forget previously learned task when it learns a new task. Here we argue that the solution to this problem may be found in the human brain, specifically, by combining functions of the two regions: the striatum and the hippocampus, which is pivotal for reinforcement learning and memory recall relevant to the current context, respectively. The mechanism of these brain regions provides insights into resolving catastrophic forgetting and long training time of DNNs. Referring to the hippocampus-striatum network we discuss design principles of combining different types of DNNs for building a new BCI architecture, called “Meta BCI”.
更多查看译文
关键词
LSTM,Catastrophic forgetting,learning rate,Deep neural network,pattern completion,neuroscience-inspired AI
AI 理解论文
溯源树
样例
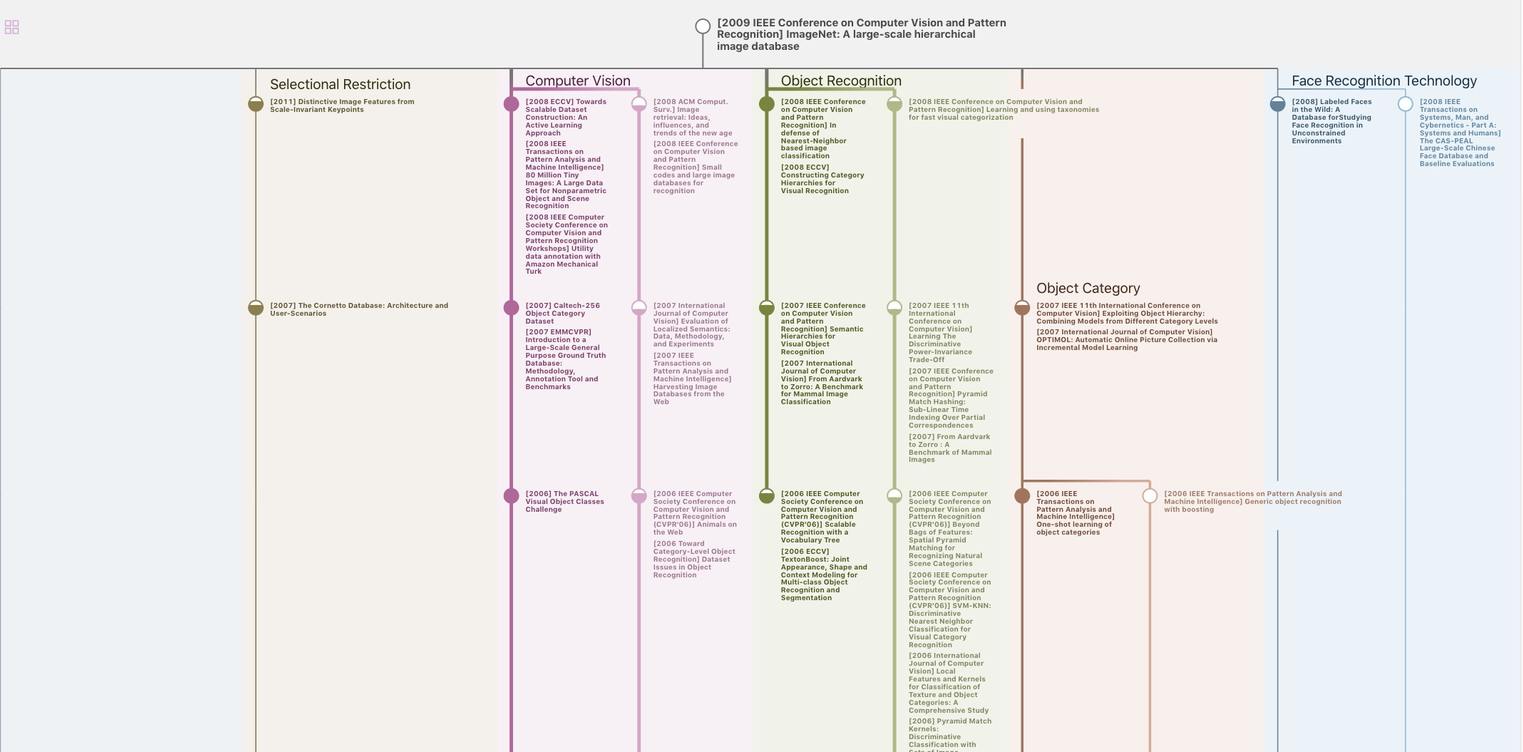
生成溯源树,研究论文发展脉络
Chat Paper
正在生成论文摘要