Digital Predistortion for Wideband Wireless Power Amplifiers Using Real-value Neural Network with Attention Mechanism
2019 PHOTONICS & ELECTROMAGNETICS RESEARCH SYMPOSIUM - FALL (PIERS - FALL)(2019)
摘要
Artificial neural networks (ANNs) have been widely used in predistorter design due to their excellent approximation capability. Generally, the inputs of existing models include Cartesian in-phase and quadrature phase (I/Q) components and envelope-dependent terms with a certain memory depth. However, these methods do not distinguish between I/Q components and envelope-dependent terms of different delay signals, resulting in complex network structures and high computational complexity.In this paper, a novel digital predistortion (DPD) technique, which uses the real-value neural network (NN) structure with the attention mechanism, is proposed for linearization of the wideband wireless power amplifier (PA). The input of this structure considers the I/Q components and the amplitude terms of the signal. Then, items that contribute more to linearization are screened from them using the real-valued NN with the attention mechanism. Finally, the screened items are injected into the real-valued NN to design the predistorter. The inputs considered in the algorithm contain rich predistortion information, and other information needed is easily fitted through them, which require a small network size. Since the screened input items contain almost all of the predistortion information, this approach further reduces network size while ensuring no degradation in linearization performance.Doherty PA is tested with a 100-MHz OFDM signal to verify the effectiveness of the proposed DPD method. Experimental results show that the proposed DPD method can achieve the same linearization performance as traditional ANN models while requiring fewer input items. Meanwhile, the proposed DPD method shows fewer model coefficients than the DNN model and the NN model.
更多查看译文
关键词
wideband wireless power amplifier,attention mechanism,artificial neural networks,computational complexity,real-value neural network structure,digital predistortion,linearization performance
AI 理解论文
溯源树
样例
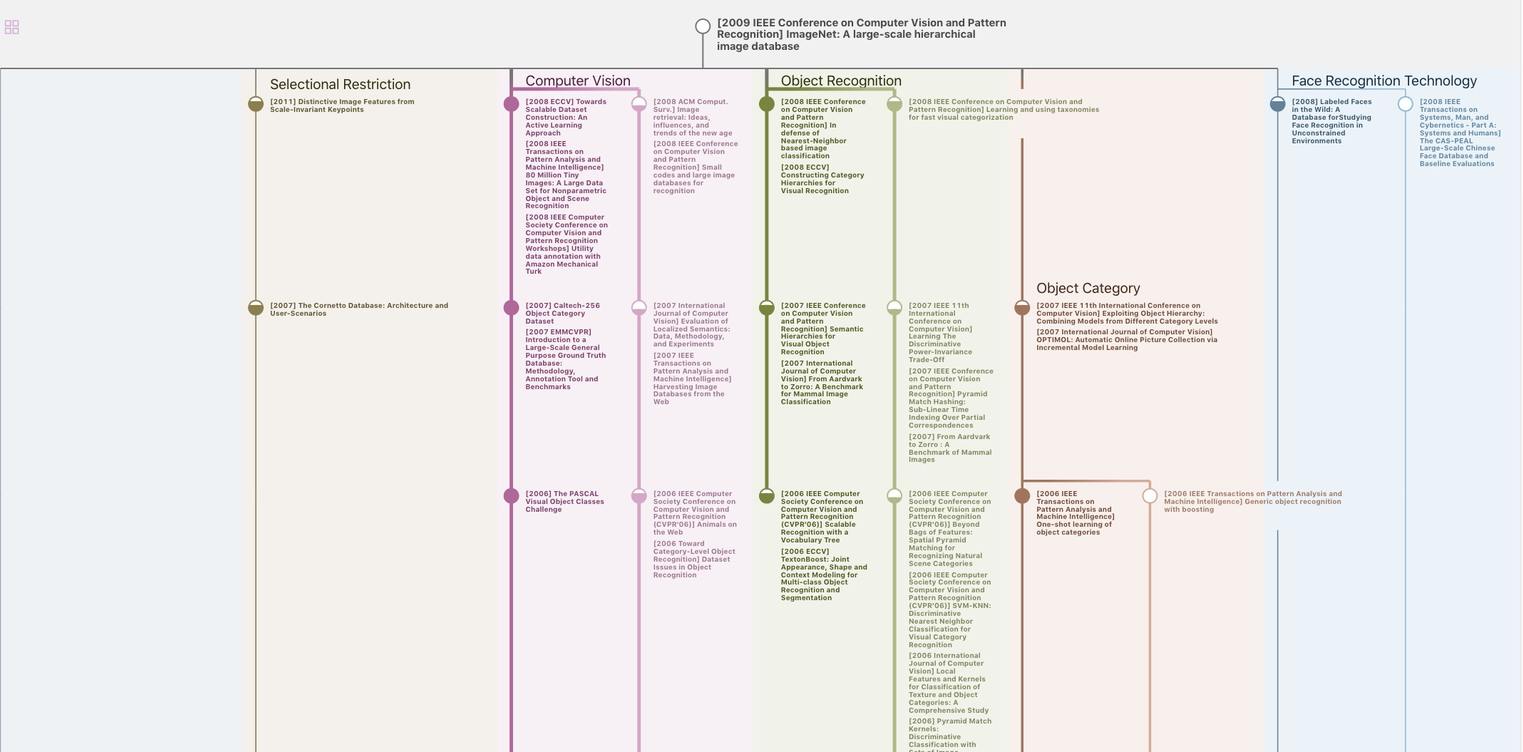
生成溯源树,研究论文发展脉络
Chat Paper
正在生成论文摘要