Learning Classifier Families For Object Detection And Parameter Estimation
COMPUTATIONAL VISION AND MEDICAL IMAGE PROCESSING: VIPIMAGE 2011(2012)
摘要
We describe methods that can learn a single family of classifiers for detecting object classes that exhibit large within-class variation. One common solution is to use a divide-and-conquer strategy, where the space of possible within-class variations is partitioned, and different detectors are trained for different partitions. However, these discrete partitions tend to be arbitrary in continuous spaces, and the classifiers have limited power when there are too few training samples in each subclass. To address this shortcoming, explicit feature sharing has been proposed, but it also makes training more expensive. We show that foreground-background classification (detection) and within-class classification of the foreground class (pose estimation) can be jointly solved in a multiplicative form of two kernel functions. One kernel measures similarity for foreground-background classification. The other kernel accounts for latent factors that control within-class variation and implicitly enables feature sharing among foreground training samples. The multiplicative kernel formulation enables feature sharing implicitly; the solution for the optimal sharing is a byproduct of SVM learning. The resulting detector family is tuned to specific variations in the foreground. The effectiveness of this framework is demonstrated in experiments that involve detection, tracking, and pose estimation of human hands, faces, and vehicles in video.
更多查看译文
AI 理解论文
溯源树
样例
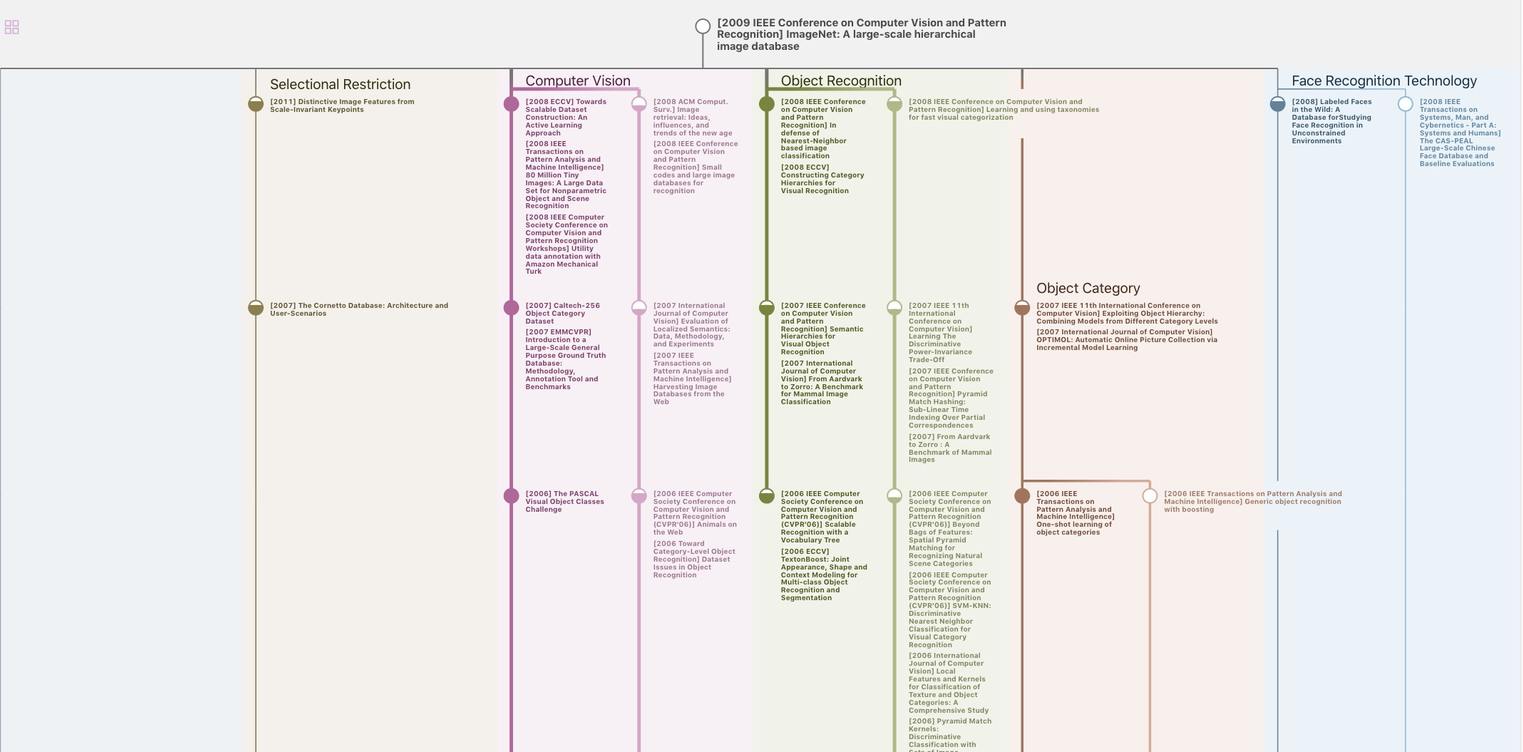
生成溯源树,研究论文发展脉络
Chat Paper
正在生成论文摘要