Enhanced Gans For Satellite Behavior Discovery
SENSORS AND SYSTEMS FOR SPACE APPLICATIONS XIII(2020)
摘要
Space superiority includes space protection and space situational awareness (SSA), which require rapid and accurate space object behavioral motion and operational intent discovery. The presence of clutter in addition to real-time and hidden information constraints greatly complicates the space awareness decision-making to control both ground-based and space-based surveillance assets. To increase SSA, generative adversarial networks (GANs) are realizable for rapid discovery of evasive satellite behaviors. Although GANs have shown good results in synthesizing real-world images, GANs "remain remarkably difficult to train" and "approaches to attacking this problem still rely on heuristics that are extremely sensitive to modifications". This paper describes a modification to a game-theoretic approach to incorporate GANs and train the networks using a general sum game theory. The enhanced GAN model for satellite behavior discovery is called Space unveiled Behavior GAN (SuB-GAN) in this paper. The structure includes training the GANs as a repeated game using a Fictious play concept framework, within which the discriminator (resp. generator) is updated according to the best response to the mixture outputs from a sequence of previously trained. In particular, the discriminator outputs converge to the optimum discriminator function and the mixed output from the sequence of trained generators converges to the data distribution. The simulated training datasets are used to demonstrate the enhanced GANs in the SSA domain. The performance the SuB-GAN is compared with the convolutional neural network (CNN) models showing promising results.
更多查看译文
关键词
Space situational awareness, GANs, satellite characterization, sensor models, simulated training data, training performance, general-sum games, CNN
AI 理解论文
溯源树
样例
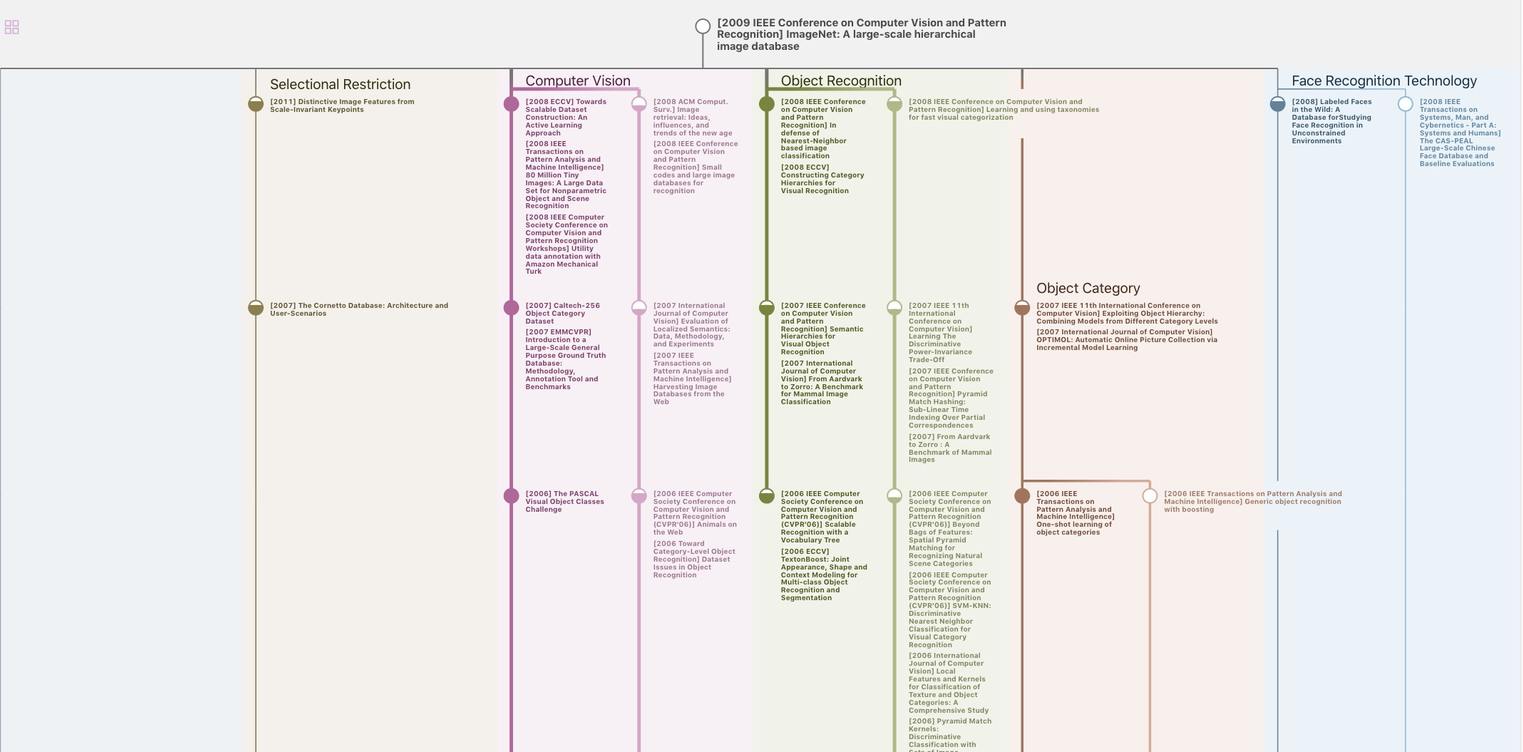
生成溯源树,研究论文发展脉络
Chat Paper
正在生成论文摘要