Prediction Of Enhancer Rna Activity Levels From Chip-Seq-Derived Histone Modification Combinatorial Codes
2017 IEEE INTERNATIONAL CONFERENCE ON BIOINFORMATICS AND BIOMEDICINE (BIBM)(2017)
摘要
Motivation: Transcription-regulatory elements (TREs) are critical modulators of gene transcription, with promoters and enhancers being major hubs for gene regulation. Of these, enhancers are distal regulatory elements, typically short 50-150 basepairs DNA regions and are identified via the large numbers of genomic regions displaying enhancer-like chromatin features, such as histone modifications at regulatory sites. Given that enhancers operate at sites vastly removed from their cognate genes, their computational prediction is challenging. While ChIP-seq datasets have been used to map out parts of the epigenome, only a fraction of predicted enhancers have been found to be functionally active. Thus, recent methods, such as GRO-seq, have been used to elucidate functionally active enhancers. However, GRO-seq data is sparse, being experimentally complex, and often at insufficient resolution. Hence, in this paper, we evaluate the ability of ChIP-seq data to predict the eRNA activity, as given by GRO-seq, using as inputs the signatures of key histone modifications that are known to be predictive of enhancers. We implement two models with accurate performance for the entire range of eRNA activity, spanning 5 orders of magnitude, for the human embryonic stem cell type H1. We then perform a detailed comparison with a prior computational approach for (binary) enhancer activity classification.Results: Our best performing model is the Convolutional Neural Network (CNN)-based regression model, indicating that the epigenetic patterns are location invariant. We achieve an overall RMSE of 0.82 compared to the baseline's (ZeroR) 1.30. In the comparison with a prior approach, our model achieves AUC of 0.75 against the prior approach's 0.56.
更多查看译文
关键词
component, Enhancer prediction, GRO-seq, convolutional neural network, histone modification signatures
AI 理解论文
溯源树
样例
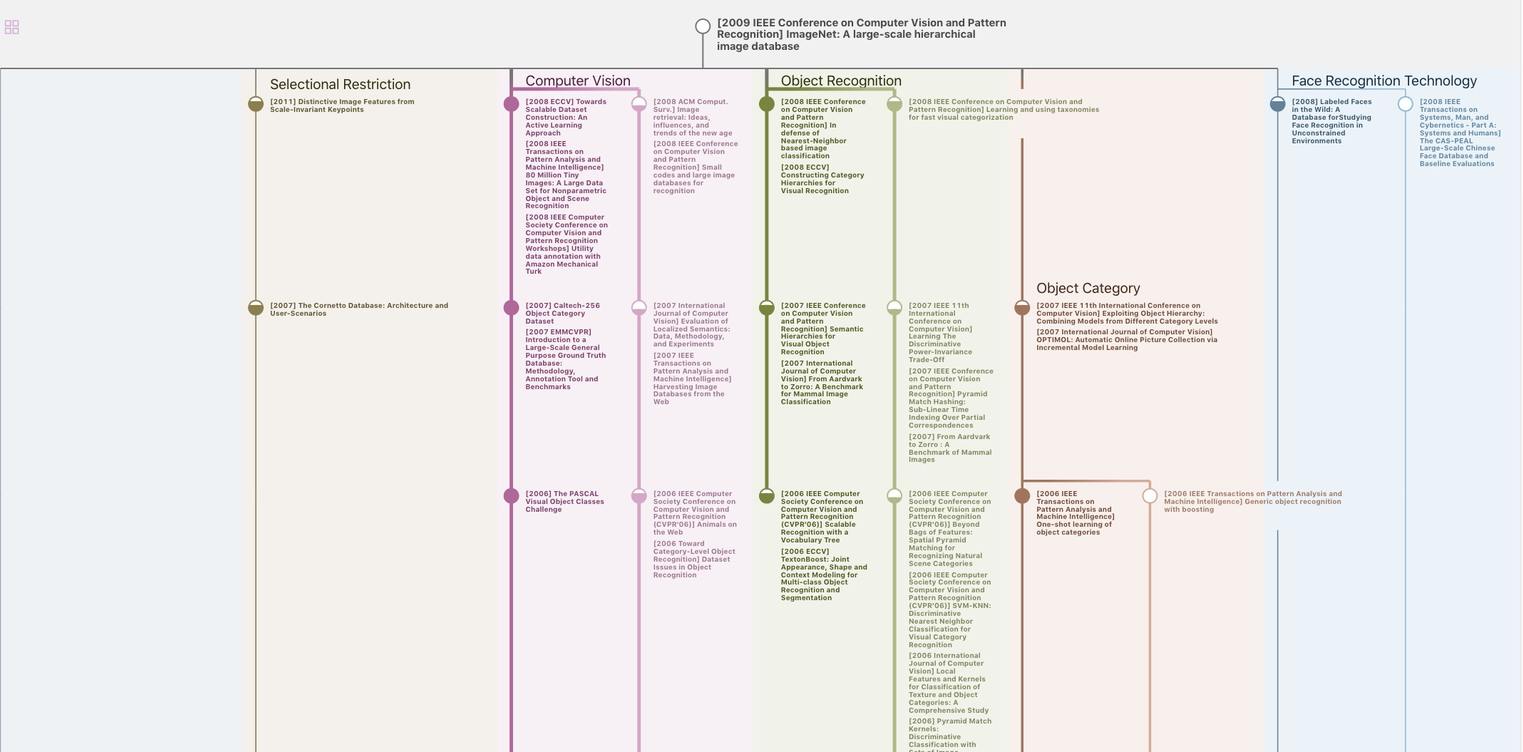
生成溯源树,研究论文发展脉络
Chat Paper
正在生成论文摘要