Detecting Mammographic Masses Via Image Retrieval And Discriminative Learning
ARTIFICIAL INTELLIGENCE IN DECISION SUPPORT SYSTEMS FOR DIAGNOSIS IN MEDICAL IMAGING(2018)
摘要
During the past half century, numerous computer-aided diagnosis (CAD) approaches have been proposed to assist the detection of masses in mammograms. Most of these methods are based on either machine learning or content-based image retrieval (CBIR) techniques. Nevertheless, either category has its limitations. Learning-based methods are affected by the fact that masses have large variation in shape and size and are often indistinguishable from surrounding tissues. CBIR-based methods, on the other hand, rely heavily on radiologist-specified suspicious regions and cannot work fully automatically. To overcome the drawbacks of both kinds of methods, we introduce an automatic CAD approach that integrates image retrieval and discriminative learning. A large set of previously diagnosed mammographic masses are collected to form an exemplar database. A query mammogram is first matched with all the exemplar masses, getting a series of similarity maps. Then, these maps are subtracted by discriminatively learned thresholds to eliminate noise. At last, individual similarity maps are aggregated, and local maxima in the final map are selected as masses. For each detected mass, the most similar exemplar masses are also presented to the radiologist. Compared with learning-based methods, our approach could achieve better mass detection accuracy since it utilizes rare exemplar masses to detect "unusual" query masses. Moreover, it provides radiologists with relevant diagnosed cases as decision support. Compared with CBIR-based methods, our approach serves as a fully automated "double reading" aid without radiologists' labeling of suspicious regions. Our approach is validated on a dataset constructed from the digital database for screening mammography (DDSM), which consists of 2,021 exemplar masses, 500 mammograms containing masses and 500 mammograms depicting healthy breasts. The proposed approach achieves high mass detection accuracy and retrieval precision, comparing favorably with traditional methods.
更多查看译文
关键词
Mammography, Breast masses, Computer-aided diagnosis (CAD) Content-based image retrieval (CBIR), Machine learning
AI 理解论文
溯源树
样例
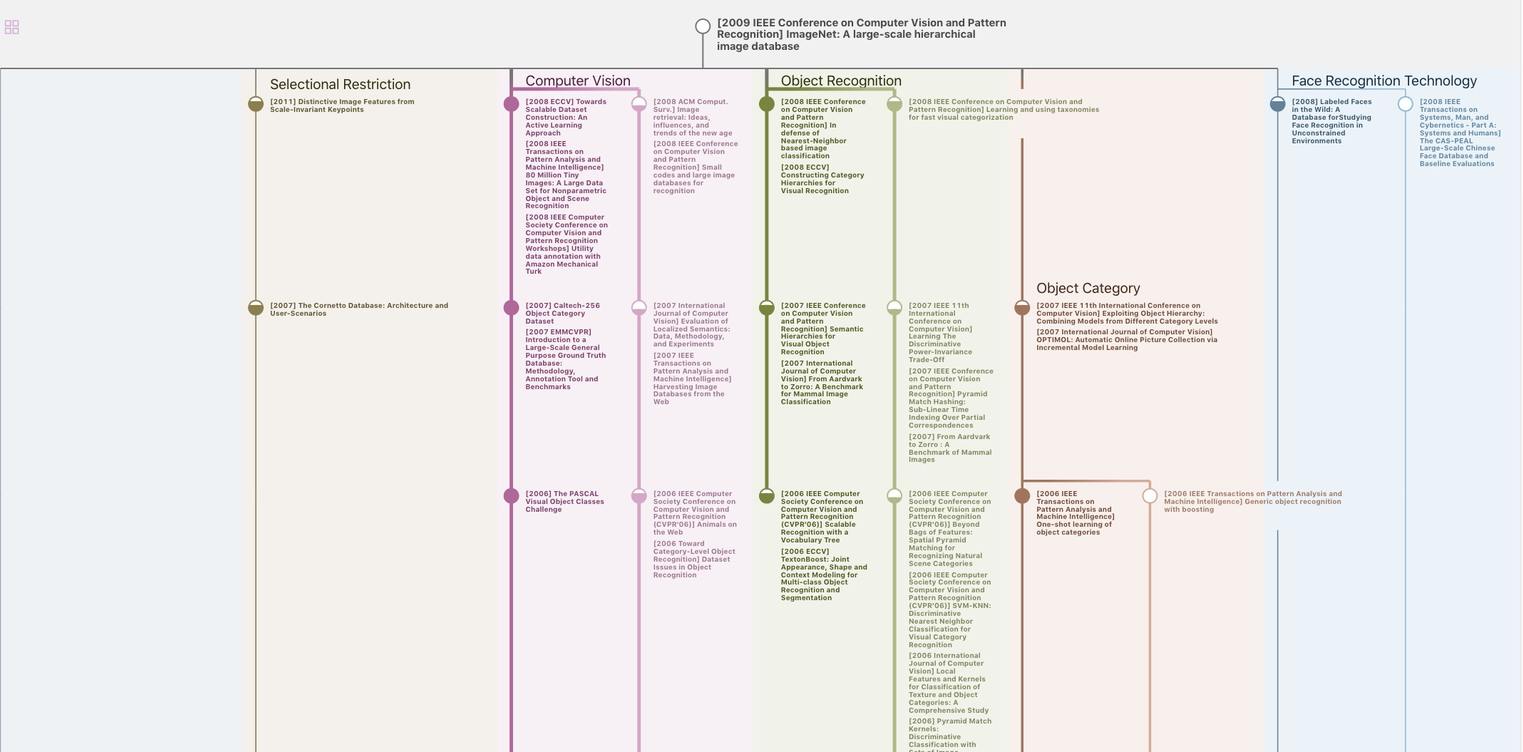
生成溯源树,研究论文发展脉络
Chat Paper
正在生成论文摘要