Surface Roughness Prediction Based On Surface Temperature And Tool Vibration Using Bp Neural Network On Turning Machine
JOURNAL OF THE CHINESE SOCIETY OF MECHANICAL ENGINEERS(2020)
摘要
In machining process, the quality of surface finish is an important requirement for many turned workpieces. Thus the controlling of machining conditions is very important for improving surface quality. This paper proposes an in-process monitoring system of surface temperature and tool vibration and discusses on surface roughness prediction based on surface temperature and tool vibration. The authors incorporate a new training scheme to BP (back propagation) neural network, namely reinforced strategy of variable learning rate (RVLR), to predict surface roughness using cutting parameters and performance characteristics, surface temperature and tool vibration. Finally, the paper shows surface roughness prediction results. Compared with SD (steepest descent) update method and traditional strategy of variable learning rate (TVLR), the RVLR method required shorter processing time to converge to the global minimum of least mean squared error. SD update method lead neural network fall into local minimum, 26.18 m(2)/min(2). With either the TVLR or the RVLR method, the network was able to avoid settling at the local minimum and reach the global minimum. However, their respective least mean square errors were 9.23 m(2)/min(2) and 8.21 m(2)/min(2) as the best-record. In addition, the new learning algorithm only required a quarter of the TVLR processing time to reach the "stable" region. This method would be helpful in selecting cutting parameters and controlling of surface temperature and tool vibration for the required surface quality.
更多查看译文
关键词
RVLR, Surface quality, Surface temperature, Tool vibration
AI 理解论文
溯源树
样例
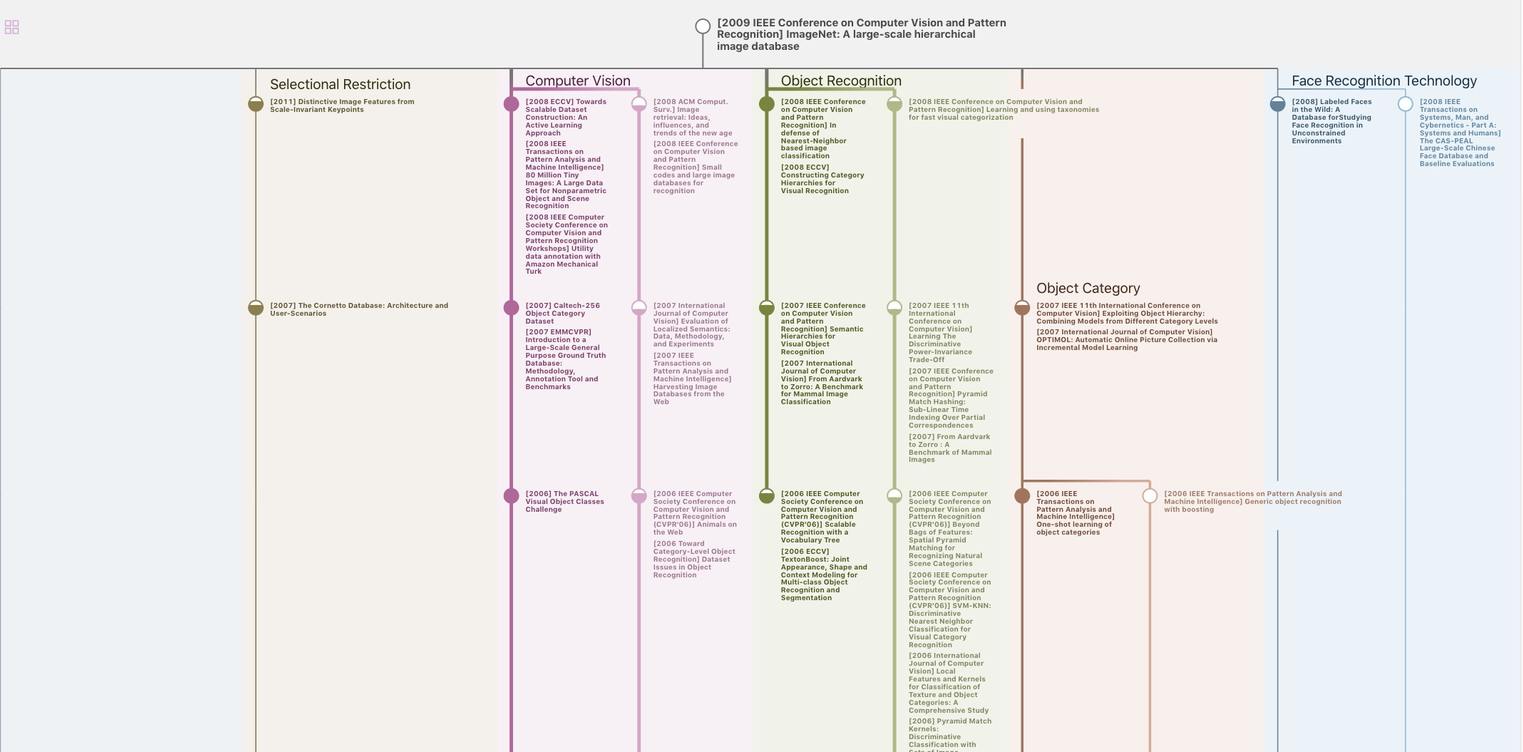
生成溯源树,研究论文发展脉络
Chat Paper
正在生成论文摘要