An Opencl(Tm) Deep Learning Accelerator On Arria 10
FPGA'17: PROCEEDINGS OF THE 2017 ACM/SIGDA INTERNATIONAL SYMPOSIUM ON FIELD-PROGRAMMABLE GATE ARRAYS(2017)
摘要
Convolutional neural nets (CNNs) have become a practical means to perform vision tasks, particularly in the area of image classification. FPGAs are well known to be able to perform convolutions efficiently, however, most recent efforts to run CNNs on FPGAs have shown limited advantages over other devices such as GPUs. Previous approaches on FPGAs have often been memory bound due to the limited external memory bandwidth on the FPGA device. We show a novel architecture written in OpenCL (TM), which we refer to as a Deep Learning Accelerator (DLA), that maximizes data reuse and minimizes external memory bandwidth. Furthermore, we show how we can use the Winograd transform to significantly boost the performance of the FPGA. As a result, when running our DLA on Intel's Arria 10 device we can achieve a performance of 1020img/s, or 23img/s/W when running the AlexNet CNN benchmark. This comes to 1382 GFLOPs and is 10x faster with 8.4x more GFLOPS and 5.8x better efficiency than the state-of-the-art on FPGAs. Additionally, 23 img/s/W is competitive against the best publicly known implementation of AlexNet on nVidia's TitanX GPU.
更多查看译文
关键词
Deep Neural Network,Convolution Neural Network
AI 理解论文
溯源树
样例
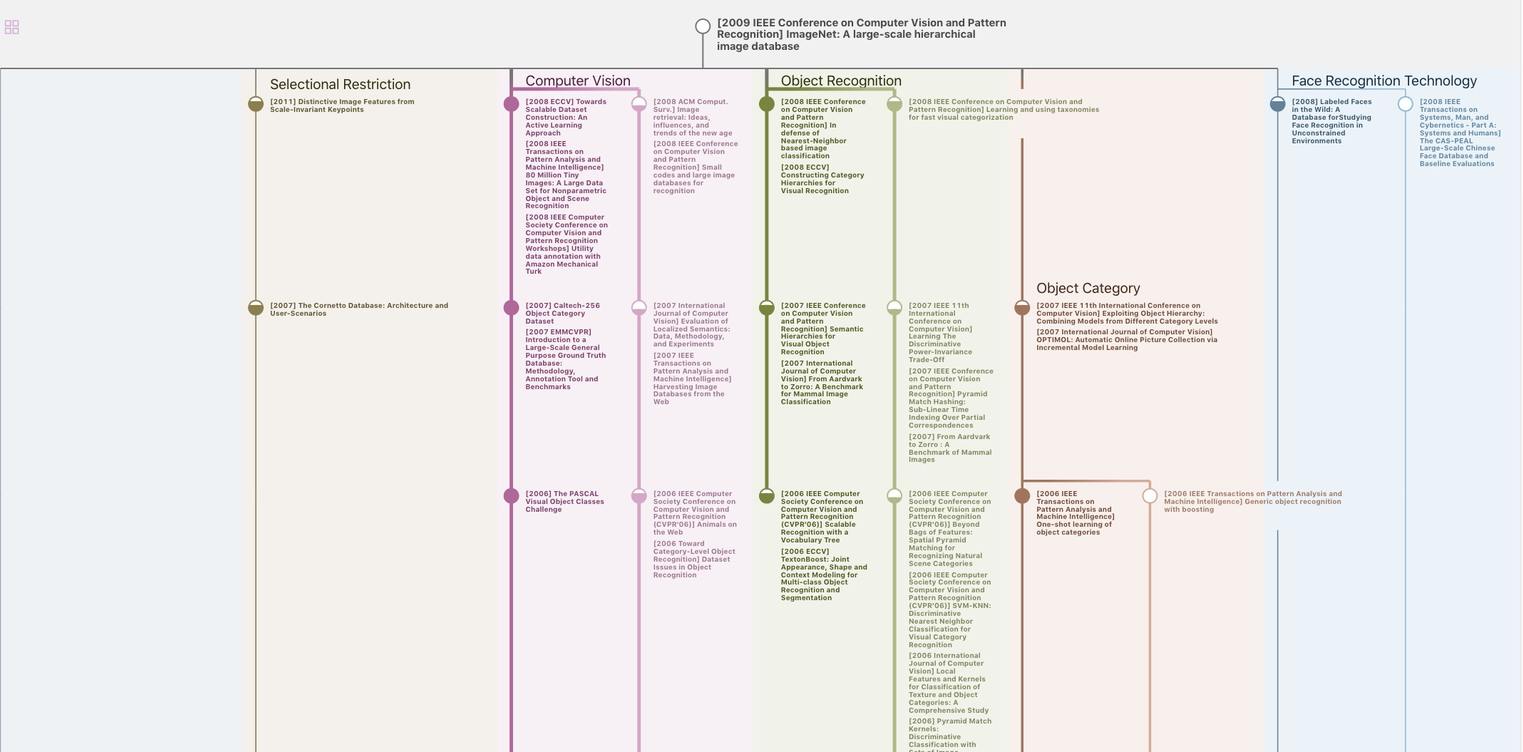
生成溯源树,研究论文发展脉络
Chat Paper
正在生成论文摘要