Variational Inference Over Nonstationary Data Streams For Exponential Family Models Dagger
MATHEMATICS(2020)
摘要
In many modern data analysis problems, the available data is not static but, instead, comes in a streaming fashion. Performing Bayesian inference on a data stream is challenging for several reasons. First, it requires continuous model updating and the ability to handle a posterior distribution conditioned on an unbounded data set. Secondly, the underlying data distribution may drift from one time step to another, and the classic i.i.d. (independent and identically distributed), or data exchangeability assumption does not hold anymore. In this paper, we present an approximate Bayesian inference approach using variational methods that addresses these issues for conjugate exponential family models with latent variables. Our proposal makes use of a novel scheme based on hierarchical priors to explicitly model temporal changes of the model parameters. We show how this approach induces an exponential forgetting mechanism with adaptive forgetting rates. The method is able to capture the smoothness of the concept drift, ranging from no drift to abrupt drift. The proposed variational inference scheme maintains the computational efficiency of variational methods over conjugate models, which is critical in streaming settings. The approach is validated on four different domains (energy, finance, geolocation, and text) using four real-world data sets.
更多查看译文
关键词
latent variable models, nonstationary data streams, concept drift, variational inference, power priors, exponential forgetting
AI 理解论文
溯源树
样例
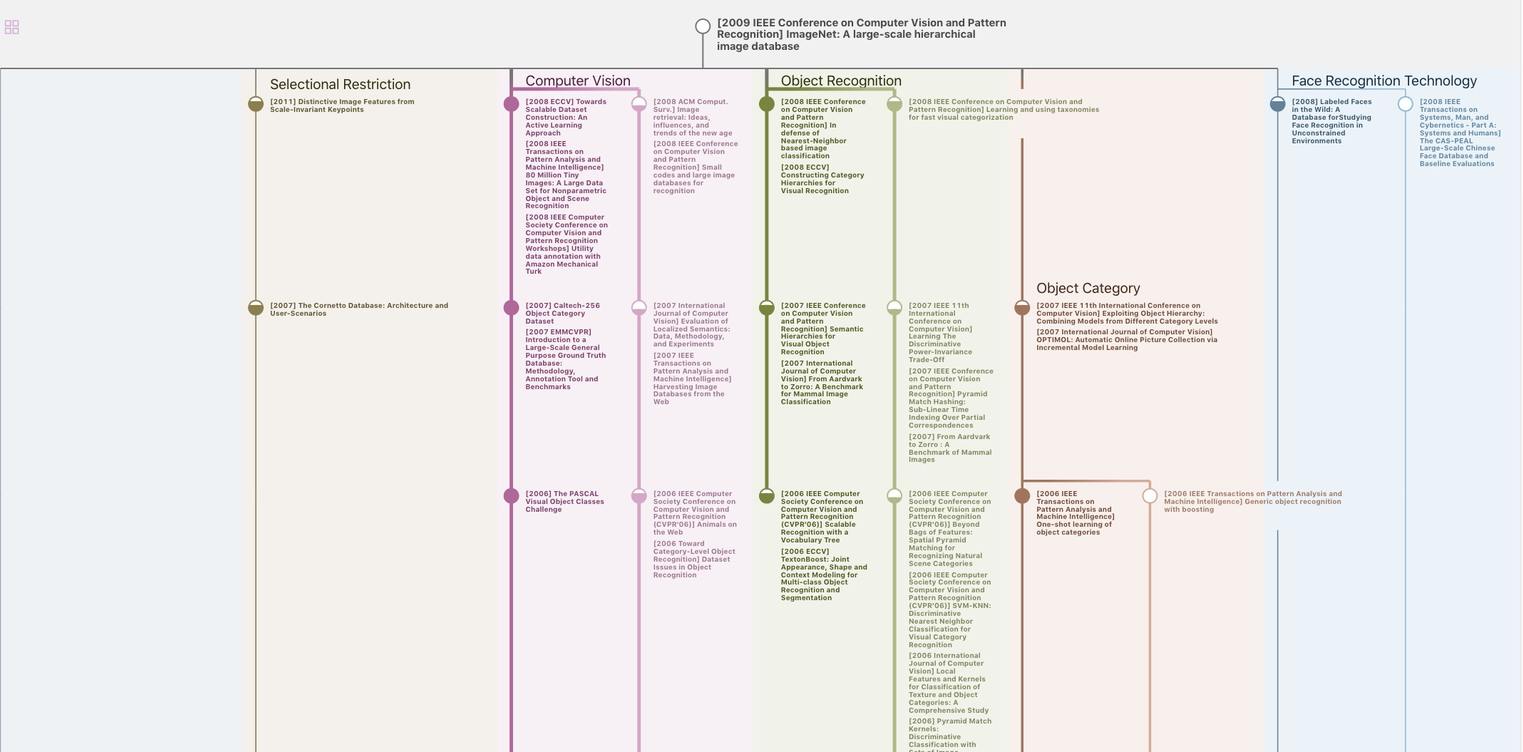
生成溯源树,研究论文发展脉络
Chat Paper
正在生成论文摘要