A Complex Systems Approach To Feature Extraction For Chaotic Behavior Recognition
2020 6th Iranian Conference on Signal Processing and Intelligent Systems (ICSPIS)(2020)
摘要
Chaotic behavior may be observed in many natural and human-made time series, thus one of the first things to acquire is the knowledge on their chaotic behavior. Several approaches including model-based and data-driven classifications are utilized to address this issue; however, the computational burden arisen with the higher performance seems to be a challenge in real world problems. Chaos theory and the complexity theory share considerable similarities, which inspired us to exploit complex system characteristics, such as complexity, emergence, self-organization and predictability as the features of chaotic time series. The degree of complexity in Lorenz model is a well-known instance of chaotic behavior, demonstrated from periodic nonlinear to a chaotic state by analysis of Hopf and Pitchfork bifurcations and then the corresponding time series are gathered in a dataset. Subsequently, the complex systems characteristics are introduced as proper features for the generated dataset, and finally these features are extracted and validated via different machine learning methods with different evaluation metrics. The simulation results reveal the excellent performance of 92 percent precision on the test data, based on the extracted features. It is shown that the most important features to recognize the chaotic behavior are complexity, emergence, predictability, and self-organization.
更多查看译文
关键词
Chaotic Time Series,Complex Systems,Lorenz System,Emergence,Self-organization,Predictability,Hurst Exponent
AI 理解论文
溯源树
样例
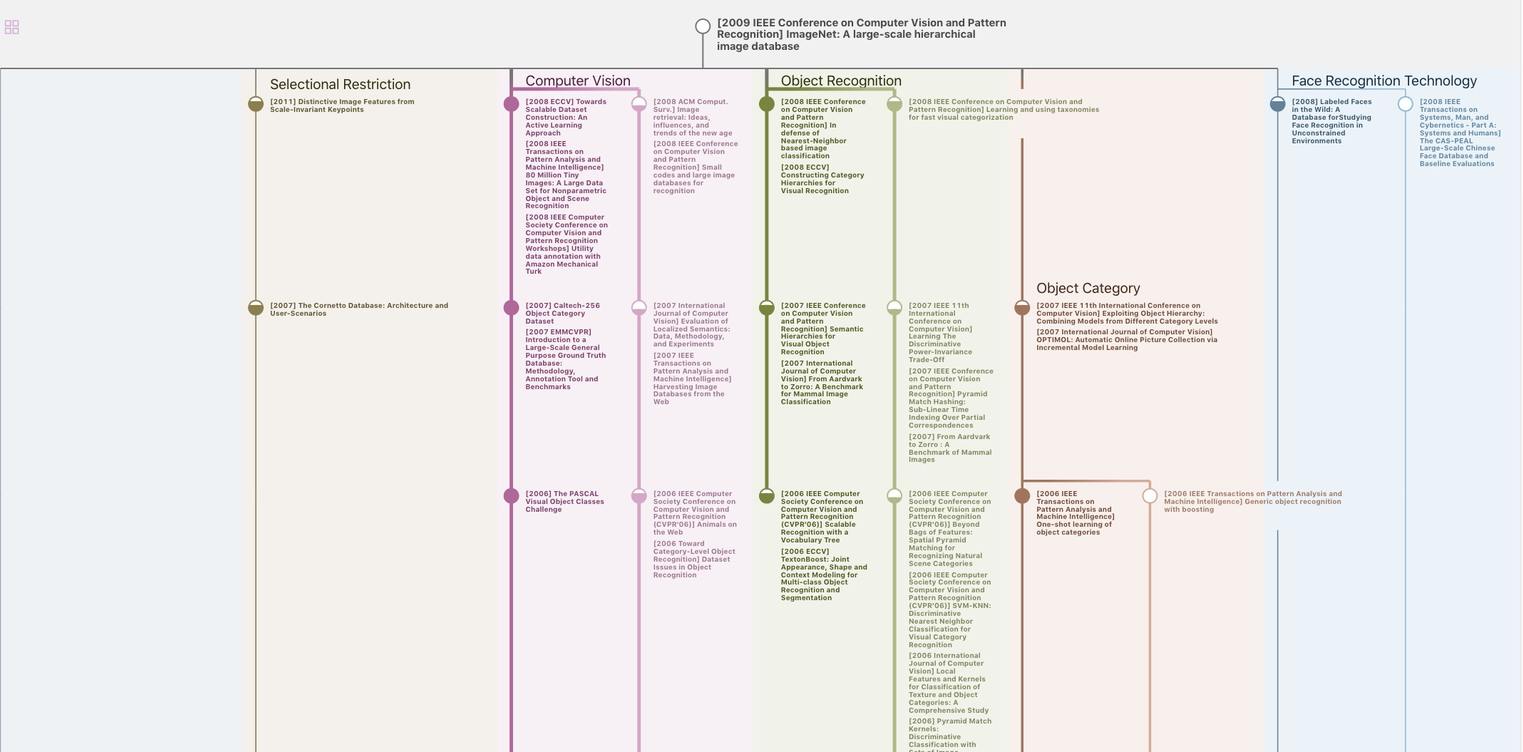
生成溯源树,研究论文发展脉络
Chat Paper
正在生成论文摘要