PM2.5 Forecasting Using LSTM Sequence to Sequence Model in Taichung City
wos(2020)
摘要
Accuracy and speed are crucial in the machine learning forecasting. Specifically, when encountering high variance segments like sequence forecasting case. For example, air quality data has various time-series variables such as temperature, CO, rainfall, wind speed, O3, SO2, and many more. To predict such as the PM2.5 which based on various parameters needs state of the art methods on a combination of forecasting models and machine learning methods. The Long Short Term Memory Networks (LSTM) autoencoders are capable of handling with a sequence of input. In this case, the predictive modeling problems involving sequence to sequence prediction problems called seq2seq network. In this paper, a sequence forecasting model is proposed for the air quality in Taichung City Taiwan, that is consist of five areas, Xitun, Chungming, Fengyuan, Dali, and Shalu. Statistic correlation analysis was implemented to find better accuracy and speed. A comparison of before and after using statistic correlation analysis in the LSTM seq2seq modeling is provided to examine the accuracy, speed, and variance score.
更多查看译文
关键词
LSTM, seq2seq, PM2.5, Deep learning
AI 理解论文
溯源树
样例
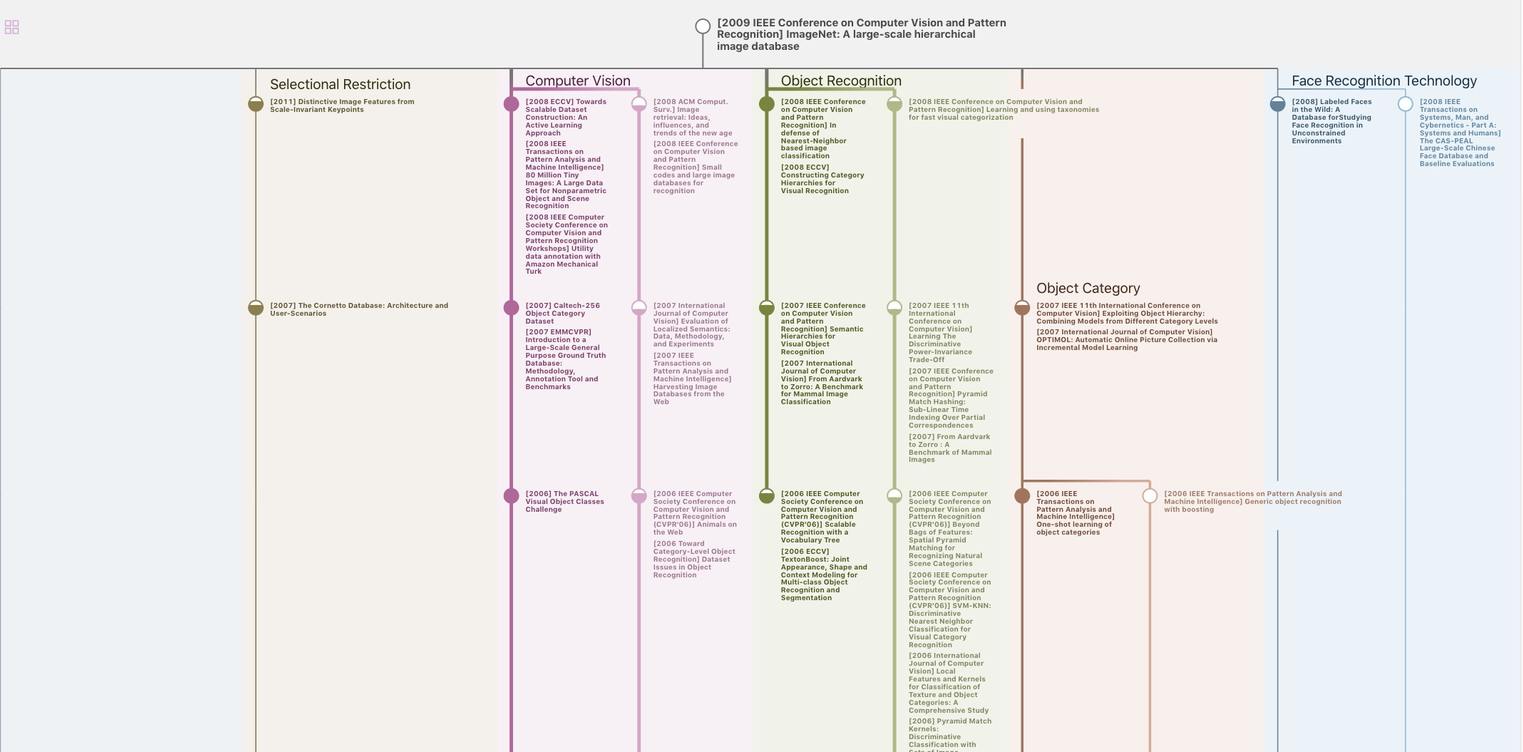
生成溯源树,研究论文发展脉络
Chat Paper
正在生成论文摘要