Time-Resolved Denoising Using Model Order Reduction, Dynamic Mode Decomposition, And Kalman Filter And Smoother
JOURNAL OF COMPUTATIONAL DYNAMICS(2020)
摘要
In this research, we investigate the application of Dynamic Mode Decomposition combined with Kalman Filtering, Smoothing, and Wavelet Denoising (DMD-KF-W) for denoising time-resolved data. We also compare the performance of this technique with state-of-the-art denoising methods such as Total Variation Diminishing (TV) and Divergence-Free Wavelets (DFW), when applicable. Dynamic Mode Decomposition (DMD) is a data-driven method for finding the spatio-temporal structures in time series data. In this research, we use an autoregressive linear model resulting from applying DMD to the time-resolved data as a predictor in a Kalman Filtering-Smoothing framework for the purpose of denoising. The DMD-KF-W method is parameter-free and runs autonomously. Tests on numerical phantoms show lower error metrics when compared to TV and DFW, when applicable. In addition, DMD-KF-W runs an order of magnitude faster than DFW and TV. In the case of synthetic datasets, where the noise-free datasets were available, our method was shown to perform better than TV and DFW methods (when applicable) in terms of the defined error metric.
更多查看译文
关键词
Data driven, dynamic mode decomposition, model order reduction, Kalman Filter, denoising
AI 理解论文
溯源树
样例
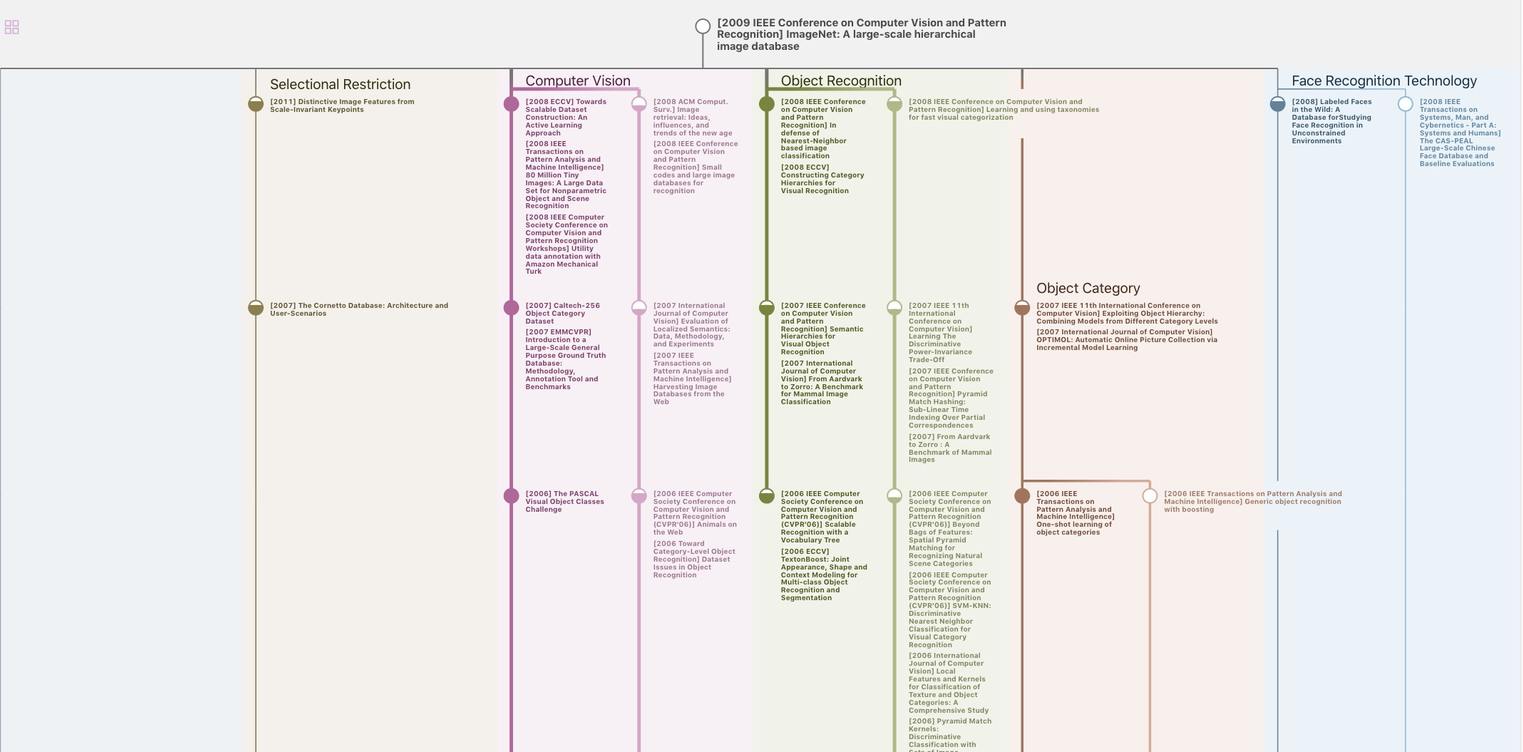
生成溯源树,研究论文发展脉络
Chat Paper
正在生成论文摘要