Six Mirnas Identified Serving As Prognostic And Predictive Markers For Osteoporosis By Mirna High-Throughput Method
INTERNATIONAL JOURNAL OF CLINICAL AND EXPERIMENTAL MEDICINE(2016)
摘要
Osteoporosis (OS) is the most common skeletal disease resulting in fracture. The aim of this study was to identify potential miRNAs that could function as specific biomarkers for identification and treatment of OS with miRNA-seq. MiRNA high-throughput data were downloaded from The Cancer Genome Atlas (TCGA) dataset. There were total 10 samples, including five normal specimen and five patient samples with spinal cord injury. Differentially expressed miRNAs were screened using SAMR package in R language with FDR<0.05 and Log FC>1. Target genes of OS were predicted by Targetscan software. And functional enrichment analysis was performed on the up- and down-regulated miRNAs by DAVID software basing on the hypergeometric analysis. Meanwhile, differentially expressed genes (DEGs) of mRNA were analyzed to construct the regulating network. Totally, 799 differentially expressed miRNAs were identified, among which six screened miRNAs, that is miR365, miR-10b, miR-129-3P, miRNA671-5p, miR-141 and miR-25, had the potential to serve as biomarkers of OS. GO and KEGG analysis revealed that three pathways, including pathway in cancer, phosphatidylinositol signaling system and endocytosis, had the greatest effect on osteoporosis disease, Meanwhile, the miRNAs related with these pathways were almost up-regulated. Moreover, the most enriched GO and KEGG terms were both related to plasma membrane, indicating its association with the occurrence of OS disease. Six miRNAs identified in the present study could serve as prognostic and predictive markers for OS patients. Phosphatidylinositol signaling system, the most enriched GO term, possessed potential application in diagnosis and treatments of OS patients.
更多查看译文
关键词
Differentially expressed miRNA, osteoporosis, miRNAs-seq, functional analysis
AI 理解论文
溯源树
样例
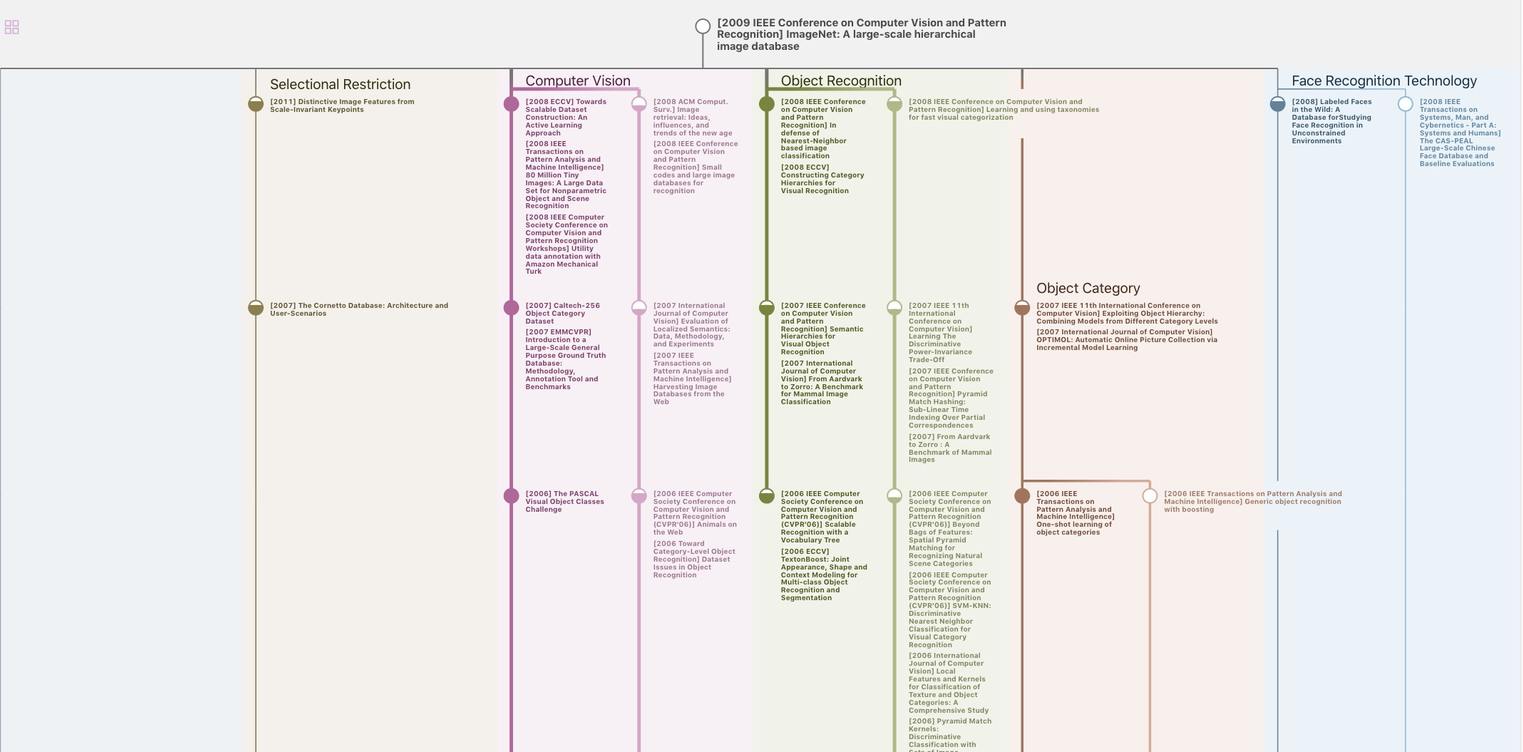
生成溯源树,研究论文发展脉络
Chat Paper
正在生成论文摘要