Directional Grid-Based Search For Simulation Metamodeling Using Active Learning
INTELLIGENT TRANSPORT SYSTEMS(2020)
摘要
Within dense urban environments, real-world transportation systems are often associated with extraordinary modeling complexity. Where standard analytic methods tend to fail, simulation tools emerge as reliable approaches to study such systems. Despite their versatility, simulation models can prove to be computational burdens, exhibiting prohibitive simulation runtimes. To address this shortcoming, metamodels are used to aid in the simulation modeling process.In this paper, we propose a directional training scheme, combining both active learning and simulation metamodeling, to address the challenge of exploring the input space, within the context of computationally expensive simulation models. Using a Gaussian Process (GP) as a simulation metamodel, we guide the exploration process towards the identification of specific regions of the input space that trigger a particular simulation output search value of interest defined a priori by the user, saving a significant amount of simulation time in the process.The results obtained from applying our methodology to an Emergency Medical Service (EMS) simulator, show that it is capable of identifying such important input regions while minimizing the number of simulation runs at the same time, thus making the simulation input space exploration process more efficient.
更多查看译文
关键词
Machine learning, Active learning, Simulation metamodeling, Gaussian Processes
AI 理解论文
溯源树
样例
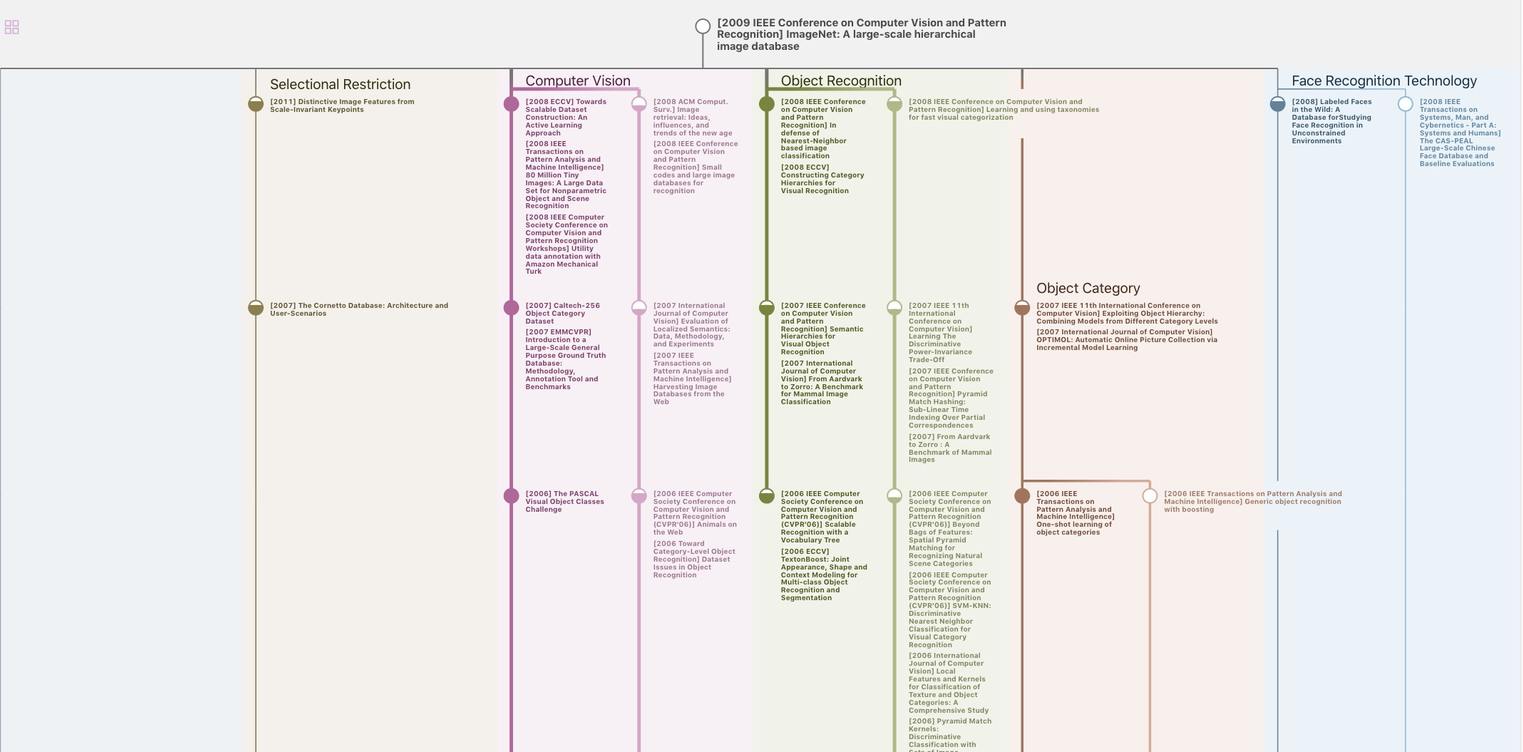
生成溯源树,研究论文发展脉络
Chat Paper
正在生成论文摘要