A Deep Neural Network Model For Hazard Classification
ARTIFICIAL INTELLIGENCE AND MACHINE LEARNING IN DEFENSE APPLICATIONS(2019)
摘要
Hazard learning algorithms employing ground penetrating radar (GPR) data for purposes of discrimination, detection, and classification suffer from a pernicious robustness problem; models trained on a particular physical region using a given sensor (antenna system) typically do not transfer effectively to diverse regions interrogated with differing sensors. We implement a novel training paradigm using region-based stratified cross-validation that improves learning induction across disparate data sets. We test this training paradigm on a novel deep neueral network architecture (DNN) and report empirical results from testing/training on data collected from multiple sites. Furthermore, we discuss the relationship between penalty loss and evaluation metrics.
更多查看译文
关键词
Ground penetrating radar, explosive hazard classification, Kolmogorov complexity, data compression
AI 理解论文
溯源树
样例
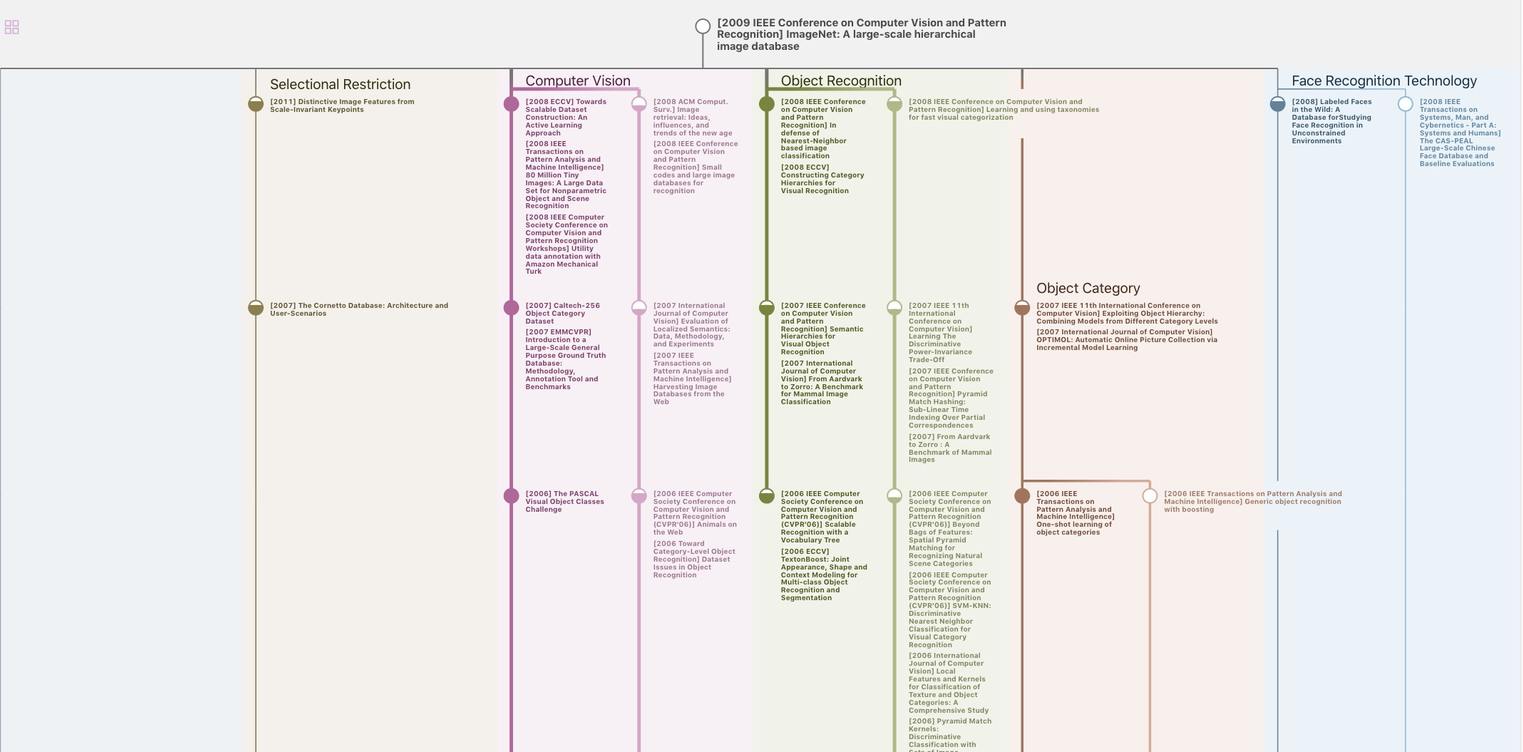
生成溯源树,研究论文发展脉络
Chat Paper
正在生成论文摘要