Improving Predictive Power Through Deep Learning Analysis Of K-12 Online Student Behaviors And Discussion Board Content
INFORMATION DISCOVERY AND DELIVERY(2020)
摘要
PurposeFor studies in educational data mining or learning Analytics, the prediction of student's performance or early warning is one of the most popular research topics. However, research gaps indicate a paucity of research using machine learning and deep learning (DL) models in predictive analytics that include both behaviors and text analysis.Design/methodology/approachThis study combined behavioral data and discussion board content to construct early warning models with machine learning and DL algorithms. In total, 680 course sections, 12,869 students and 14,951,368 logs were collected from a K-12 virtual school in the USA. Three rounds of experiments were conducted to demonstrate the effectiveness of the proposed approach.FindingsThe DL model performed better than machine learning models and was able to capture 51% of at-risk students in the eighth week with 86.8% overall accuracy. The combination of behavioral and textual data further improved the model's performance in both recall and accuracy rates. The total word count is a more general indicator than the textual content feature. Successful students showed more words in analytic, and at-risk students showed more words in authentic when text was imported into a linguistic function word analysis tool. The balanced threshold was 0.315, which can capture up to 59% of at-risk students.Originality/valueThe results of this exploratory study indicate that the use of student behaviors and text in a DL approach may improve the predictive power of identifying at-risk learners early enough in the learning process to allow for interventions that can change the course of their trajectory.
更多查看译文
关键词
Educational data mining, Deep learning, Performance prediction, Early warning, Educational text mining, Optimal threshold
AI 理解论文
溯源树
样例
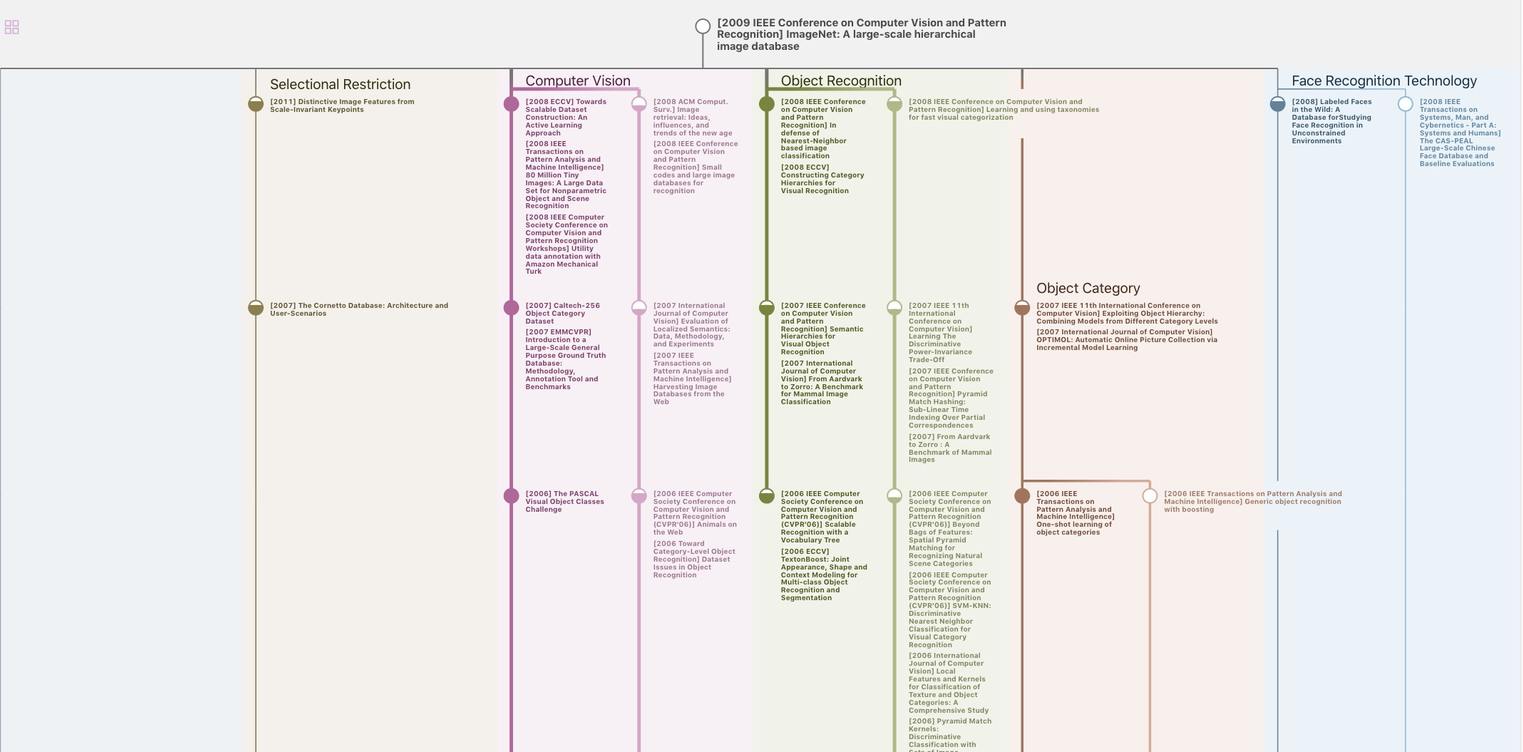
生成溯源树,研究论文发展脉络
Chat Paper
正在生成论文摘要