Leveraging Initial Hints for Free in Stochastic Linear Bandits
International Conference on Algorithmic Learning Theory (ALT)(2022)
摘要
We study the setting of optimizing with bandit feedback with additional prior knowledge provided to the learner in the form of an initial hint of the optimal action. We present a novel algorithm for stochastic linear bandits that uses this hint to improve its regret to $\tilde O(\sqrt{T})$ when the hint is accurate, while maintaining a minimax-optimal $\tilde O(d\sqrt{T})$ regret independent of the quality of the hint. Furthermore, we provide a Pareto frontier of tight tradeoffs between best-case and worst-case regret, with matching lower bounds. Perhaps surprisingly, our work shows that leveraging a hint shows provable gains without sacrificing worst-case performance, implying that our algorithm adapts to the quality of the hint for free. We also provide an extension of our algorithm to the case of $m$ initial hints, showing that we can achieve a $\tilde O(m^{2/3}\sqrt{T})$ regret.
更多查看译文
关键词
stochastic,initial hints
AI 理解论文
溯源树
样例
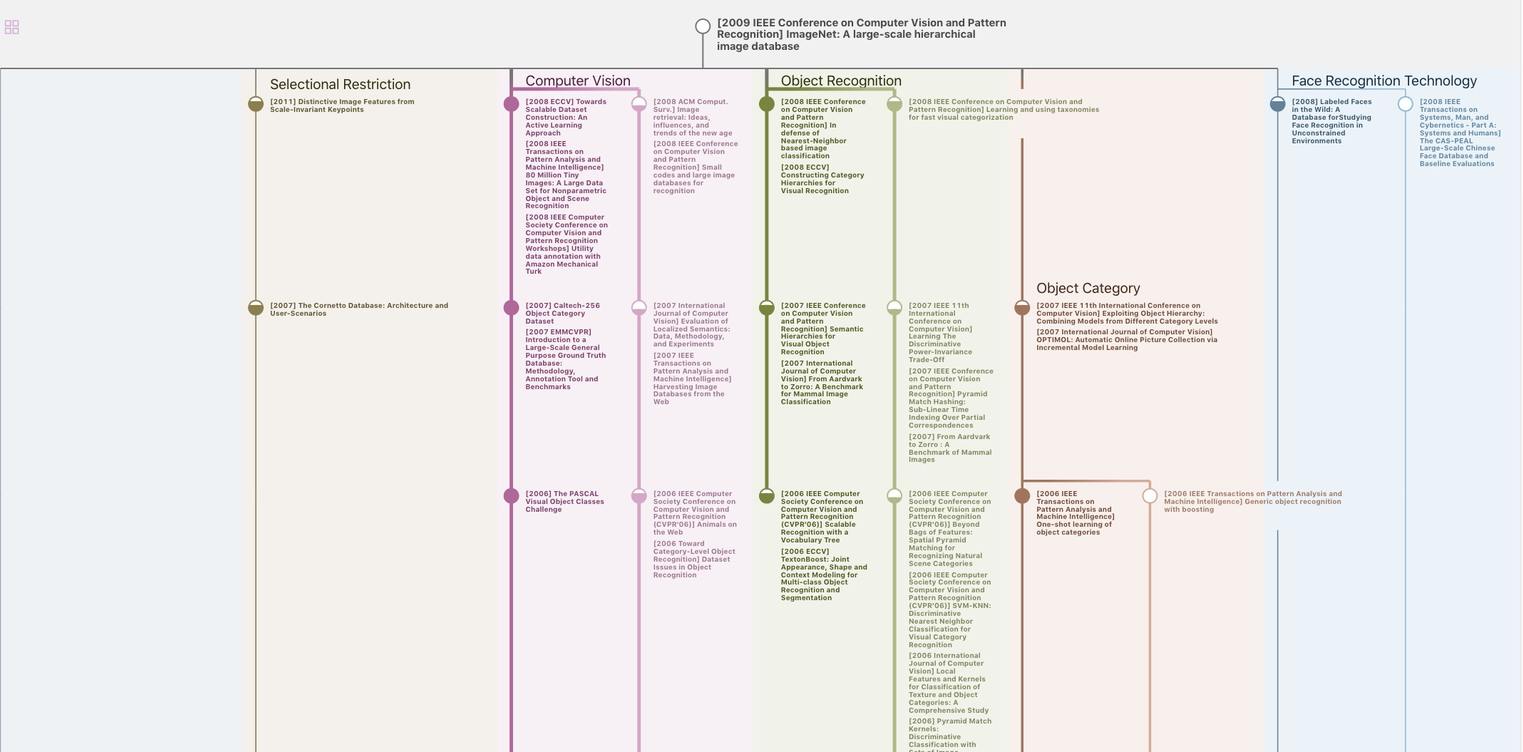
生成溯源树,研究论文发展脉络
Chat Paper
正在生成论文摘要