Cognitive Antijam Receiver System (Cars) For Gnss
PROCEEDINGS OF THE 2010 INTERNATIONAL TECHNICAL MEETING OF THE INSTITUTE OF NAVIGATION - ITM 2010(2010)
摘要
Existing antijam (AJ) and interference mitigation techniques are based on the assumption that the nature of interference is known a priori. Generally, one or more fixed antijam techniques (FATs) are applied to reduce the impact of the jammer or interferer to a receiver.A more general approach is to apply cognitive radio technology, whereby the receiver's antijam processing and receiver processing adapt to the incoming interference environment by determining the location and characteristics of the interference, then jointly optimizing signal processing to mitigate the characterized jammer or interferer. This approach is known as a Cognitive Antijam Receiver System (CARS).In order to demonstrate this approach, we use a configurable AJ scheme that will be used as a front end to a generic and configurable CDMA receiver. The scheme is based on a parameterizable fast-Fourier transform (FFT) and time domain antijam processor that characterizes and adapts to the interference/jamming signal present. This adaptation is performed jointly with adaptation of the receiver's tracking approach to optimize receiver performance subject to the characterized jammer.Bit error rate (BER) performance and tracking jitter are the primary metrics that are used in this optimization. A noncoherent early-late delay-locked loop is assumed for the purposes of signal tracking.By analyzing tracking and data demodulation performance in response to a general jamming signal, we propose an algorithm to select the antijam and receiver parameters as a function of the jamming signal properties. Performance will be assessed against common categories of jamming signals for both FATs and CARS approaches. Simulation results show that the CARS approach allows data demodulation and tracking tasks can be reliably performed even in severe jamming conditions, whereas FATs approaches fail to achieve such performance levels.
更多查看译文
AI 理解论文
溯源树
样例
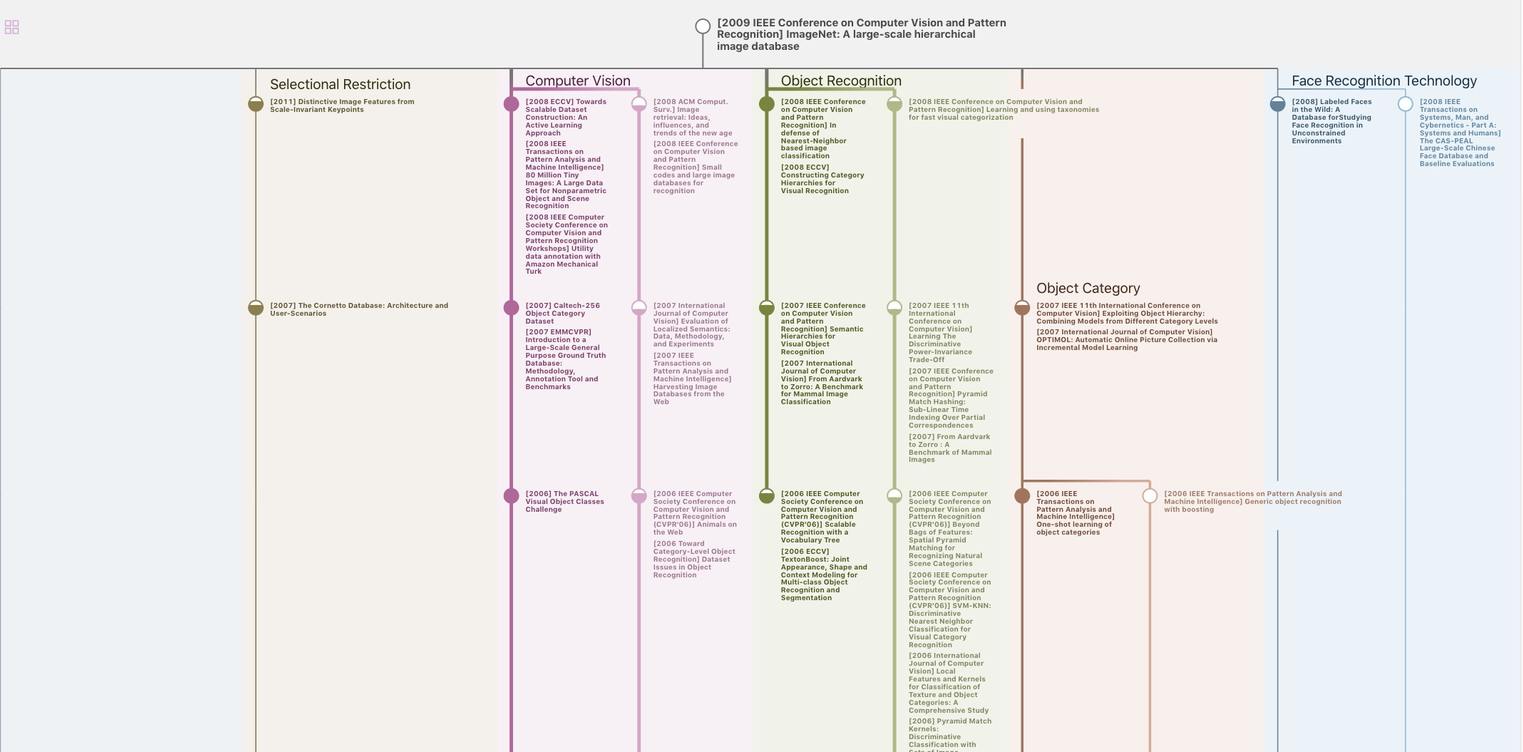
生成溯源树,研究论文发展脉络
Chat Paper
正在生成论文摘要