Non-rigid Shape Correspondence Using Surface Descriptors and Metric Structures in the Spectral Domain
wos(2016)
摘要
Finding correspondence between non-rigid shapes is at the heart of three-dimensional shape processing. It has been extensively addressed over the last decade, but efficient and accurate correspondence detection still remains a challenging task. Generalized Multidimensional Scaling (GMDS) is an approach that finds correspondence by mapping one shape into another, while attempting to preserve distances between pairs of corresponding points on the two shapes. A different approach consists in detecting correspondence between shapes by matching their pointwise surface descriptors. Recently, the Spectral GMDS (SGMDS) approach was introduced, according to which the GMDS was re-formulated in the natural spectral domain of the shapes. Here, we propose a method that combines matching based on geodesic distances and pointwise surface descriptors . Following SGMDS, in the proposed solution the entire problem is translated into the spectral domain, resulting in efficient correspondence computation. Efficiency and accuracy of the proposed method are demonstrated by comparing it to state-of-the-art approaches, using a standard correspondence benchmark.
更多查看译文
关键词
Geodesic Distance, Spectral Domain, Shape Match, Maximally Stable Extremal Region, Surface Descriptor
AI 理解论文
溯源树
样例
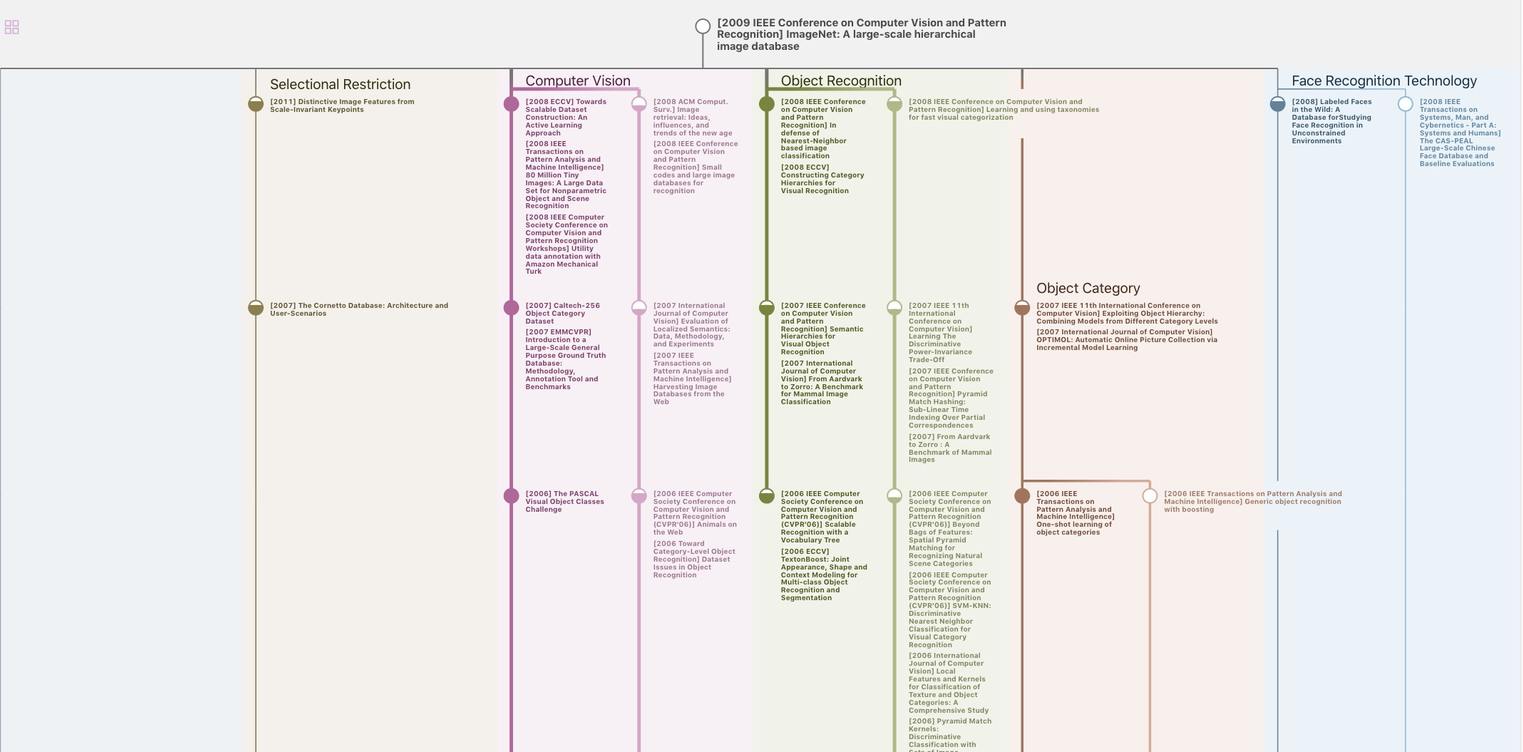
生成溯源树,研究论文发展脉络
Chat Paper
正在生成论文摘要