Non-Small Cell Lung Cancer (Nsclc) Detection By Dna Methylation Profiling And Computational Deconvolution
CANCER RESEARCH(2020)
摘要
Purpose: Early detection of pulmonary malignancies is critical to achieving positive treatment outcomes. However, due to the minimal symptom burden often associated with early-stage neoplasms, in addition to the cost and limitations of advanced imaging modalities, detection of disease is often delayed until nodal or metastatic spread has occurred. Ongoing cellular turnover releases DNA into systemic circulation where it is able to achieve a steady state level. We postulated that we could identify methylation sites specific to pulmonary Squamous Cell Carcinoma (SCC) and Adenocarcinoma (AD) and then computationally determine the presence or absence of neoplastic signatures from a heterogeneous tissue sample, such as is found in cell free DNA isolated from plasma. Methods: We designed a computational framework for the detection of informative methylation sites based on an iterative Euclidean Distance algorithm. After generation of a reference matrix comprised of 25 cells of origin, SCC and AD (via 444 biopsy-derived methylomes), we sought informative methylation sites which had unique expression patterns among the tested cell types. To quantify the reliability of this approach, we generated synthetic In sillico “plasma” samples comprised of varying compositions of SCC, AD and whole blood methylation data which we then deconvolved using a NNLS algorithm. Results: We identified 200 informative sites out of 390,000 tested. Based on the 200 identified informative sites, we were able to define a Euclidean Space with robust separation of individual cell types (L2>5). Pairings of CD4+/CD8+ T-Cells and SCC/AD had the closest Euclidean distances, likely related to cellular origin and biologic similarities. However, we accurately distinguished between these cell type pairs in >95% of In silico experiments. Additionally, we demonstrated accurate detection of neoplastic signature within synthetic heterogeneous mixtures ranging from .1 to 100% of neoplastic “spike-in” (PearsonR =.92, p=1.5e-42). Going forward, we hope to further define the operating characteristics of this approach In vivo and develop its capability as we look to recapitulate our results with plasma samples from patients with and without pulmonary malignancies. Conclusion: Here, we describe a computational framework for the detection of pulmonary malignancy via methylation signatures. Using this framework we identified 200 informative methylation sites which allow for In silico deconvolution and detection of NSCLC within heterogeneously complex samples. Citation Format: Nicholas J. Hornstein, Zorawar S. Noor, Matteo Pellegrini, Edward Garon. Non-small cell lung cancer (NSCLC) detection by DNA methylation profiling and computational deconvolution [abstract]. In: Proceedings of the Annual Meeting of the American Association for Cancer Research 2020; 2020 Apr 27-28 and Jun 22-24. Philadelphia (PA): AACR; Cancer Res 2020;80(16 Suppl):Abstract nr 149.
更多查看译文
AI 理解论文
溯源树
样例
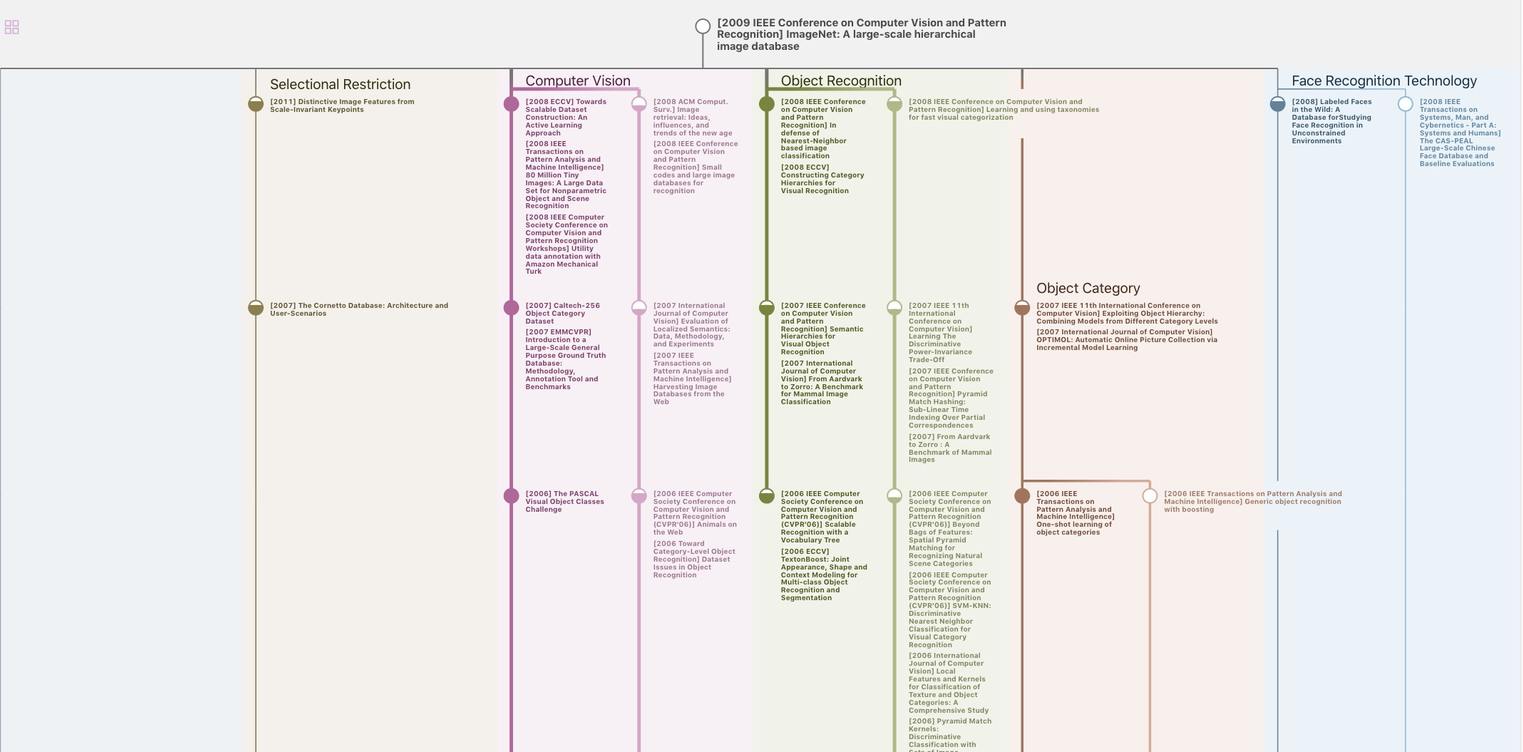
生成溯源树,研究论文发展脉络
Chat Paper
正在生成论文摘要