Multiscale convolutional neural network for detecting paroxysmal atrial fibrillation from single lead ECG signals
2020 IEEE Applied Signal Processing Conference (ASPCON)(2020)
摘要
Atrial fibrillation (AF) is one of the most common chronic cardiac arrhythmias often associated with stroke, heart failure, coronary artery disease, etc. Based on the stage of progression and chances of restorability, AF can be categorized into paroxysmal, persistent and permanent. Among all, automatic detection of paroxysmal (early stage) AF (PAF), is challenging as it is clinically silent and occurs in short duration (episodic pattern) an has a high chances of being undiagnosed. In this paper, we present a multiscale deep convolution neural network (MS-DCNN) framework for automatic detection of paroxysmal AF episodes from single lead short electrocardiogram (ECG) signals. The MS-DCNN employs the architecture of multiple-stream CNNs for the multiscale decomposed single lead ECG signal. The proposed method is evaluated on the Physionet/CinC Challenge 2017 ECG dataset consists of 5048 normal sinus rhythm (NSR) and 756 AF rhythm subjects acquired using AliveCor hand-held device. The proposed method achieves an average accuracy, sensitivity, specificity and F1-score of 84.31%, 84.80%, 83.82% and 84.31% respectively on the validation dataset.
更多查看译文
关键词
Atrial Fibrillation (AF),Convolutional Neural Network (CNN),Electrocardiogram (ECG),Feature Concatenation,Multi-Scale,Spectrogram
AI 理解论文
溯源树
样例
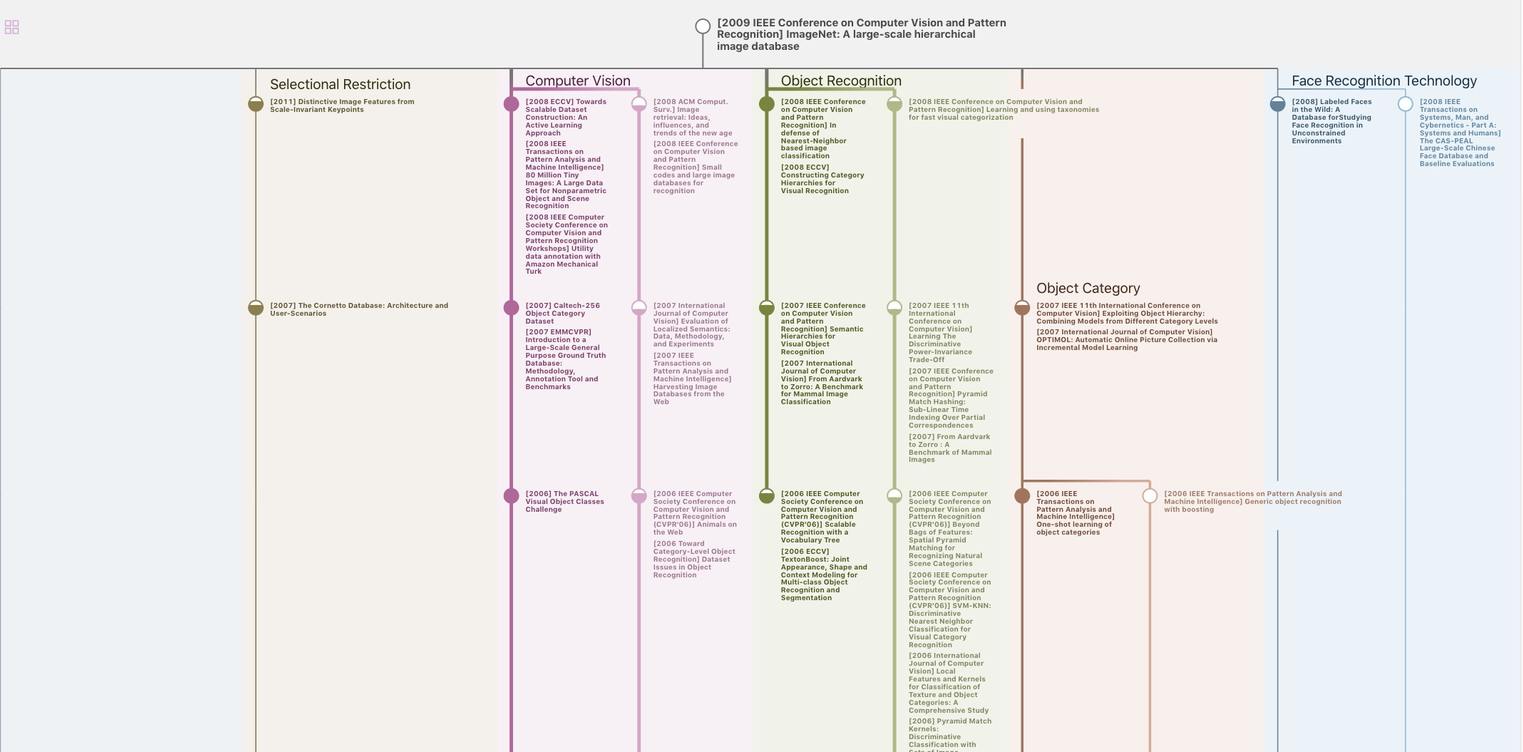
生成溯源树,研究论文发展脉络
Chat Paper
正在生成论文摘要