An Efficient Deep Learning Strategy: Its Application in Sclera Segmentation
2020 IEEE Applied Signal Processing Conference (ASPCON)(2020)
摘要
Neural networks require normalized inputs which are generally small floating point numbers. Convolutional Neural Networks (CNNs) use filters that are applied to multiple layers of a color image. A technique is used in this paper to reduce the input size by converting three layers of a RGB-color image to a single matrix with floating point values at each cell. This conversion preserves the distribution of colors and inherently normalizes the input data for Deep Learning Framework such that the data is meaningful. Objective is to reduce the number of trainable parameters in a U-Net framework and increase its efficiency. The process is implemented and tested for segmentation of sclera regions from eye images using the SBVPI data-set. It shows considerable reduction in number of trainable parameters and better results in less computation time. Practically, the model executes four times faster by reducing the number of trainable parameters to one-sixteenth. It also shows increase in cross-validation F1-score to 0.939 for U-Net.
更多查看译文
关键词
Meta Learning,Probability-based representation of color images,Sclera Segmentation,U-Net based Deep Learning framework,U-Net-P
AI 理解论文
溯源树
样例
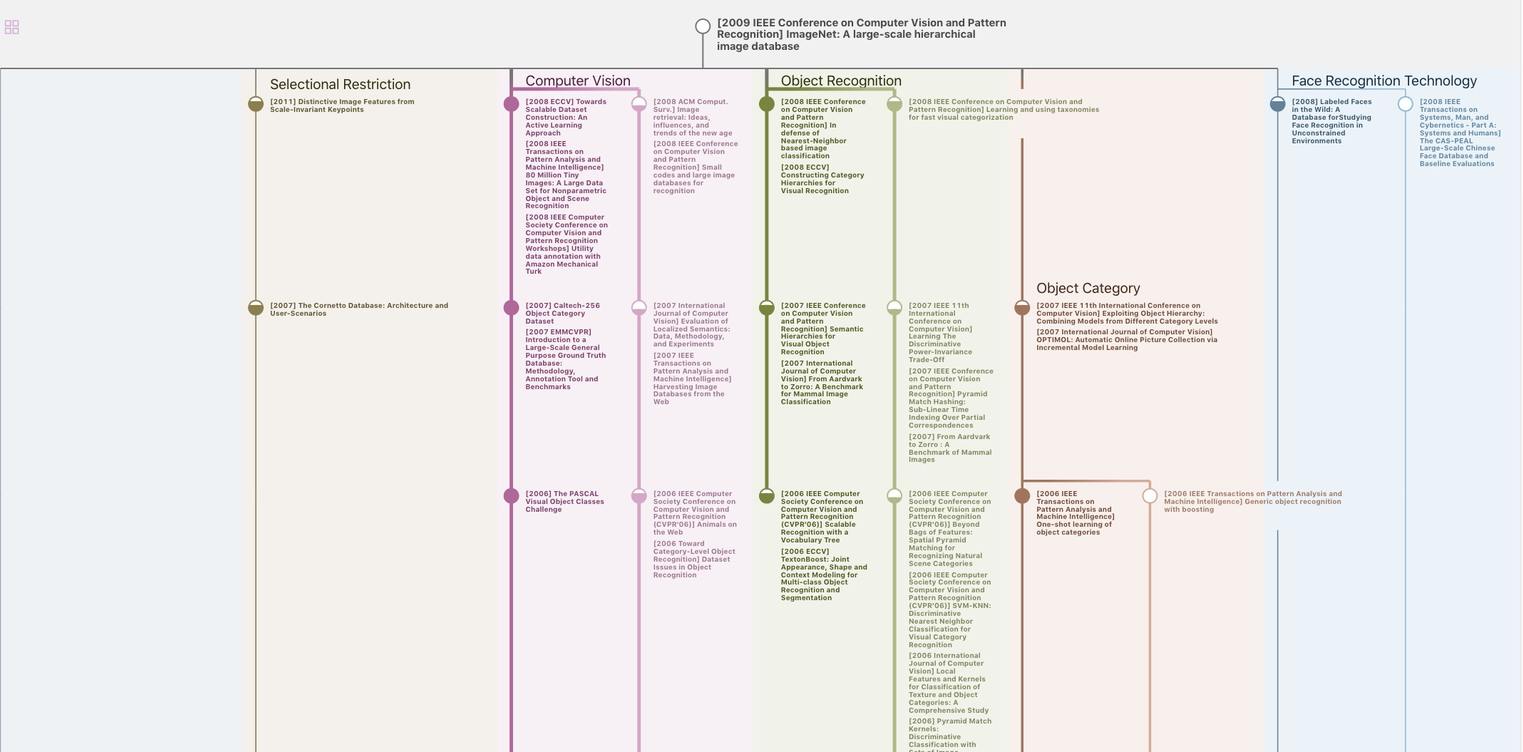
生成溯源树,研究论文发展脉络
Chat Paper
正在生成论文摘要