Feature subset selection using mutual standard deviation in sentiment mining
2017 IEEE Conference on Big Data and Analytics (ICBDA)(2017)
摘要
The complexity of an optimal feature subset selection for sentiment classification problem grows exponentially based on the number of features. The paper aims to enhance the selection of feature subset in high-dimensional feature space based on filter approach. Filter-based methods evaluate features relevance by considering only at the properties of the data. The score of the feature is calculated, then low-scoring features will be removed. In most feature selection methods, the selection of a feature subset is based on the feature space while the model hypothesis space is ignored. To assess the relevance of features, a feature ranking by distance measure is conducted to minimize intra-class and maximize inter-class distance. A filter method based on distribution and dispersion of features on feature space namely mutual standard deviation is proposed. Wide ranges of comparative experiments are performed on two widely used datasets, namely, movie, and book review dataset, in sentiment analysis. The results showed that proposed feature ranking method outperforms the other baseline methods regarding accuracy.
更多查看译文
关键词
mutual standard deviation,filter method,feature selection,sentiment analysis
AI 理解论文
溯源树
样例
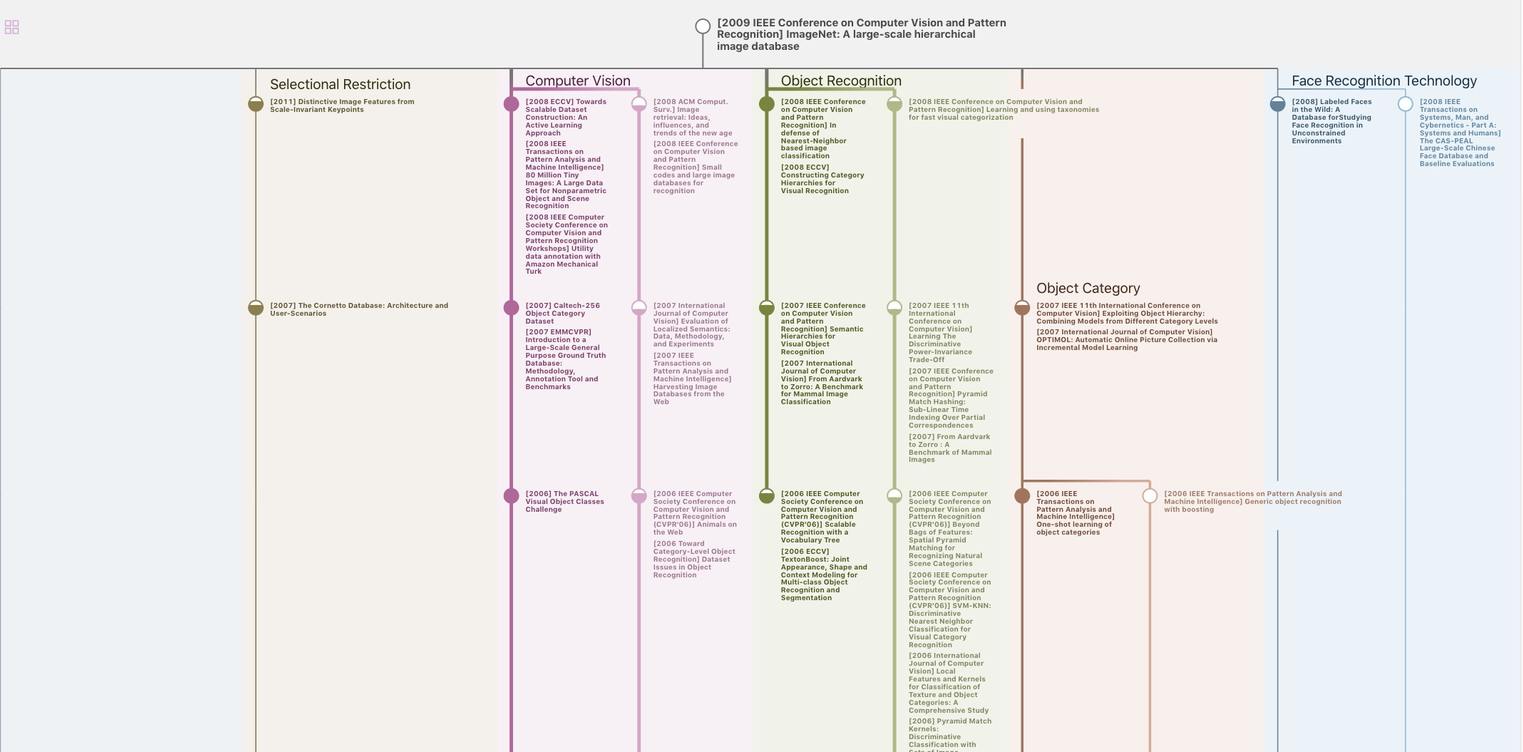
生成溯源树,研究论文发展脉络
Chat Paper
正在生成论文摘要