Enhanced Production Surveillance Using Probabilistic Dynamic Models
INTERNATIONAL JOURNAL OF PROGNOSTICS AND HEALTH MANAGEMENT(2018)
摘要
Production surveillance is the task of monitoring oil and gas production from every well in a hydrocarbon field. A key opportunity in this domain is to improve the accuracy of flow measurements per phase (oil, water, gas) from a multi-phase flow. Multi-phase flow sensors are costly and therefore not instrumented for every production well. Instead, several low fidelity surrogate measurements are performed that capture different aspects of the flow. These measurements are then reconciled to obtain per-phase rate estimates. Current practices may not appropriately account for the production dynamics and the sensor issues, thus, fall far short in terms of achieving a desired surveillance accuracy. To improve surveillance accuracy, we pose rate reconciliation as a state estimation problem. We begin with hypothesizing a model that describes the dynamics of production rates and their relationship with the field measurements. The model appropriately accounts for the uncertainties in field conditions and measurements. We then develop robust probabilistic estimators for reconciliation to yield the production estimates and the uncertainties therein. We highlight recent advancements in the area of probabilistic programming that can go a long way in improving the performance and the portability of such estimators. The exposition of our methods is accompanied by experiments in a simulation environment to illustrate improved surveillance accuracy achieved in different production scenarios.
更多查看译文
关键词
production surveillance,probabilistic rate reconciliation,probabilistic programming
AI 理解论文
溯源树
样例
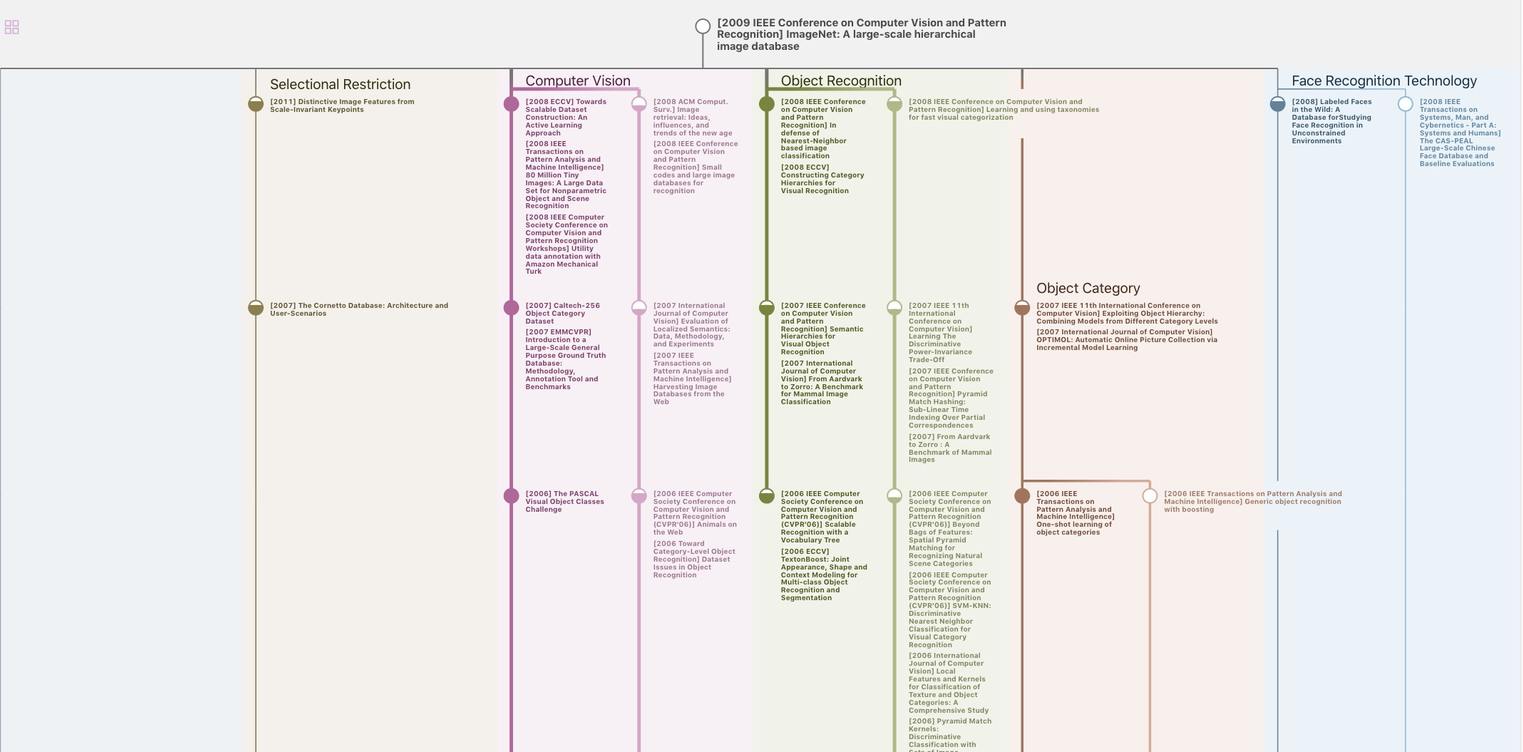
生成溯源树,研究论文发展脉络
Chat Paper
正在生成论文摘要