Image Inpainting Based On Improved Deep Convolutional Auto-Encoder Networkkey Words
CHINESE JOURNAL OF ELECTRONICS(2020)
摘要
This paper proposes an effective image inpainting method using an improved deep convolutional auto-encoder network. By analogy with exiting methods of image inpainting based on auto-decoders, inpainting methods using the deep convolutional auto-encoder networks are significantly more effective in capturing high-level features than classical methods based on exemplar. However, the inpainted regions would appear blurry and global inconsistency. To alleviate the fuzzy problem, we improved the network model by adding skip connections between mirrored layers in encoder and decoder stacks, so that the generative process of the inpainting area can directly use the low-level features information of the processing image. For making the inpainted result look both more plausible and consistent with its surrounding contexts, the model is trained with a combination of standard pixel-wise reconstruction loss and two adversarial losses which ensures pixel-accurate and local-global contents consistency. With extensive experimental on the ImageNet and Paris Streetview datasets, we demonstrate qualitatively and quantitatively that our approach performs better than state of the art.
更多查看译文
关键词
Image inpainting, Convolutional autoencoder network, Image completion, Skip connections
AI 理解论文
溯源树
样例
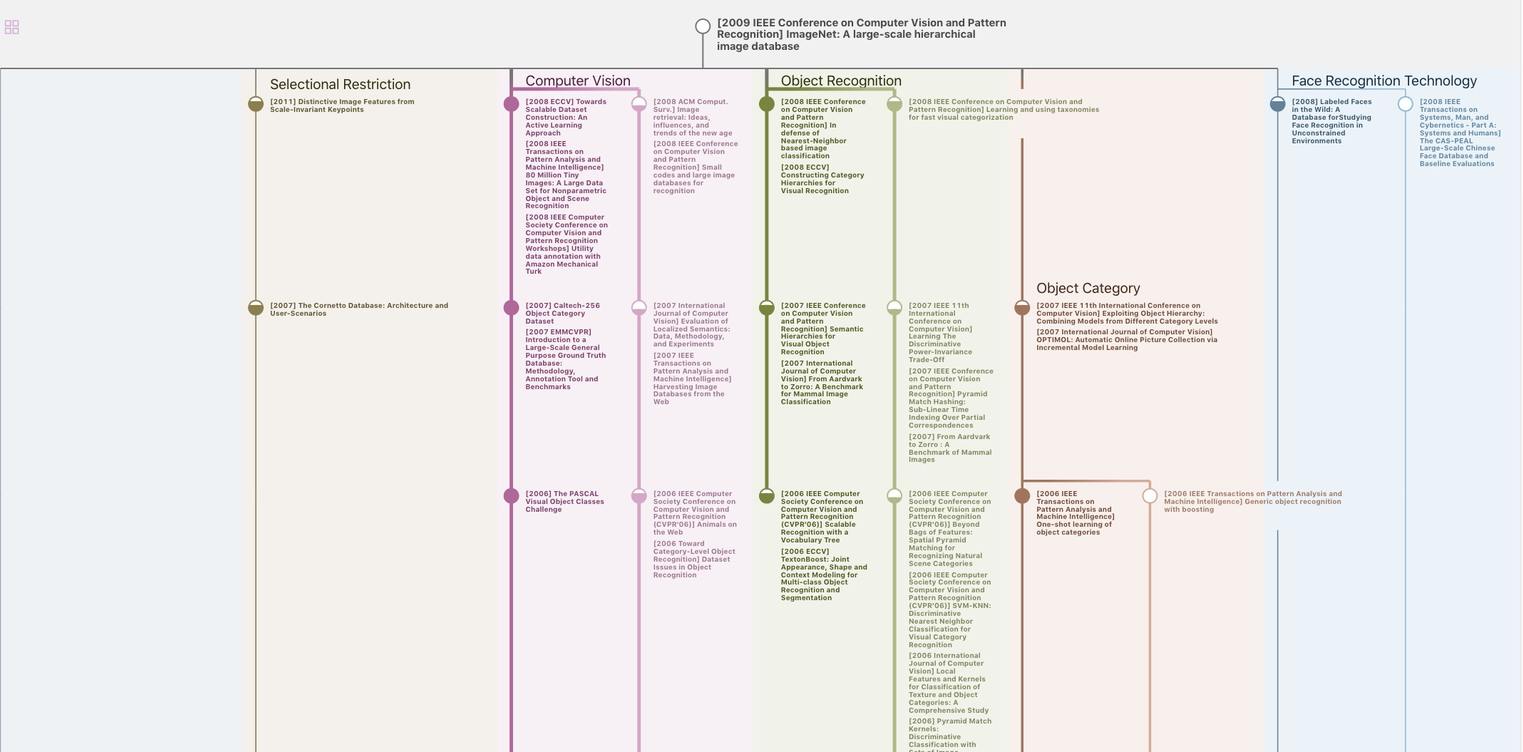
生成溯源树,研究论文发展脉络
Chat Paper
正在生成论文摘要