Anomaly Detection Using Unsupervised Machine Learning For Grid Computing Site Operation
24TH INTERNATIONAL CONFERENCE ON COMPUTING IN HIGH ENERGY AND NUCLEAR PHYSICS (CHEP 2019)(2020)
摘要
A Grid computing site is composed of various services including Grid middleware, such as Computing Element and Storage Element. Text logs produced by the services provide useful information for understanding the status of the services. However, it is a time-consuming task for site administrators to monitor and analyze the service logs every day. Therefore, a support framework has been developed to ease the site administrator's work. The framework detects anomaly logs using Machine Learning techniques and alerts site administrators. The framework has been examined using real service logs at the Tokyo Tier2 site, which is one of the Worldwide LHC Computing Grid sites. In this paper, a method of the anomaly detection in the framework and its performances at the Tokyo Tier2 site are reported.
更多查看译文
AI 理解论文
溯源树
样例
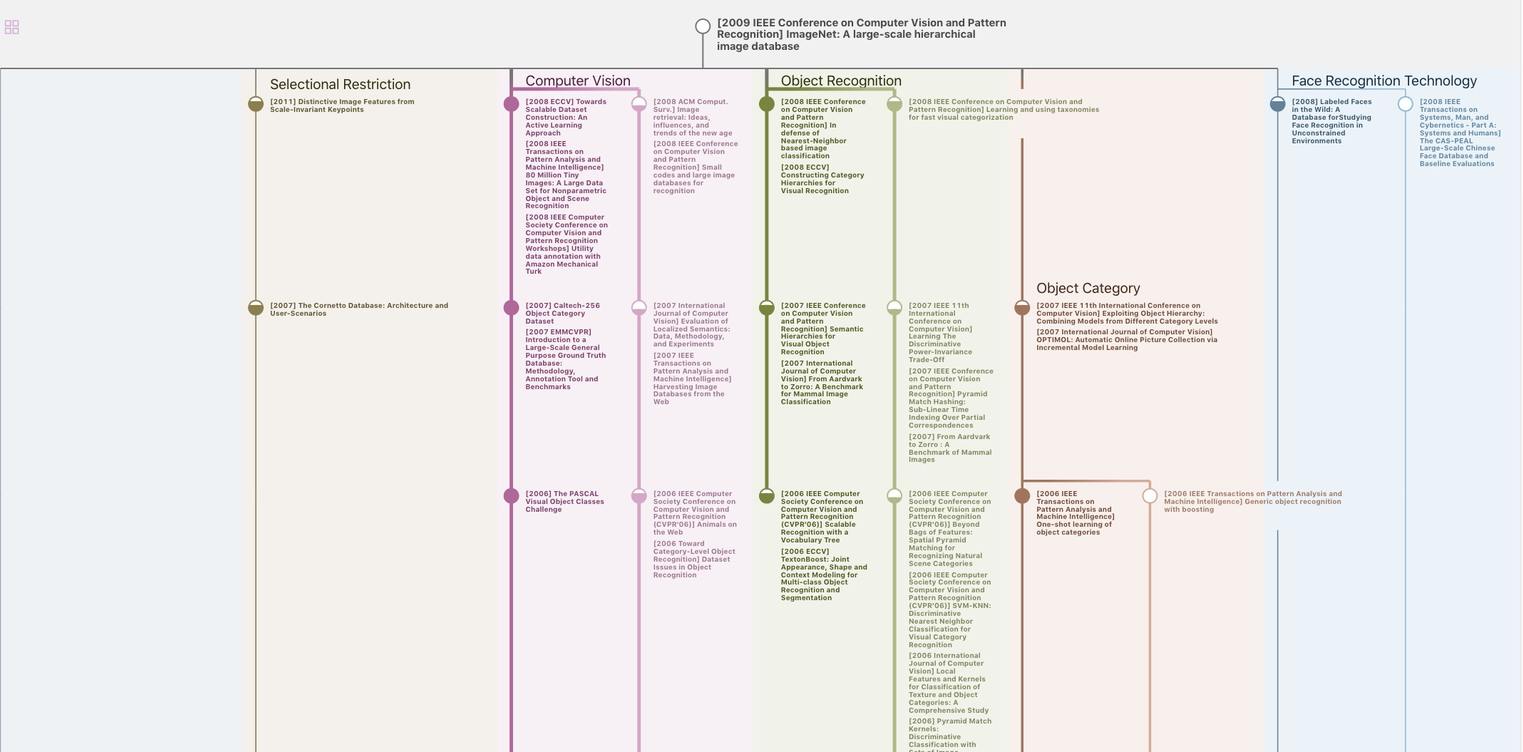
生成溯源树,研究论文发展脉络
Chat Paper
正在生成论文摘要