Study On Feature Selection And Identification Method Of Tool Wear States Based On Svm
INTERNATIONAL JOURNAL ON SMART SENSING AND INTELLIGENT SYSTEMS(2013)
摘要
This paper presents an on-line tool wear condition monitoring system for milling. The proposed system was developed taking the cost and performance in practice into account, in addition to a high success rate. The cutting vibration signal is obtained during the cutting process, and then extracting features using time-domain statistical and wavelet packet decomposition algorithms. It would result in two major disadvantages if creating a tool wear states identification model based on all extracted features, i.e. high computational cost and inefficient complexity of the model, which leads to overfitting. It is crucial to extract a smaller feature set by an effective feature selection algorithm. In this paper, an approach based on one-versus-one multi-class Support Vector Machine Recursive Feature Elimination (SVM-RFE) is proposed to solve the feature selection problem in tool wear condition monitoring. Moreover, in order to analyze a performance degradation process on the machine tool, Least Squares Support Vector Machines (LS-SVM) is introduced. In order to estimate the effectiveness of feature selection algorithm, the comparative analysis among Fisher Score (FS) Information Gain (IG) and SVM-RFE is exploited to real milling datasets. The identification result proves that: The selected feature set based on SVM-RFE is more effective to recognize tool wear state; LS-SVM wear identification method is superior to BP neural network, and it has higher identification accuracy; the proposed feature selection and identification method for tool wear states is efficient and feasible.
更多查看译文
关键词
Tool condition monitoring, feature selection, multi-class support vector machine recursive feature elimination (SVM-RFE), least squares support vector machines (LS-SVM)
AI 理解论文
溯源树
样例
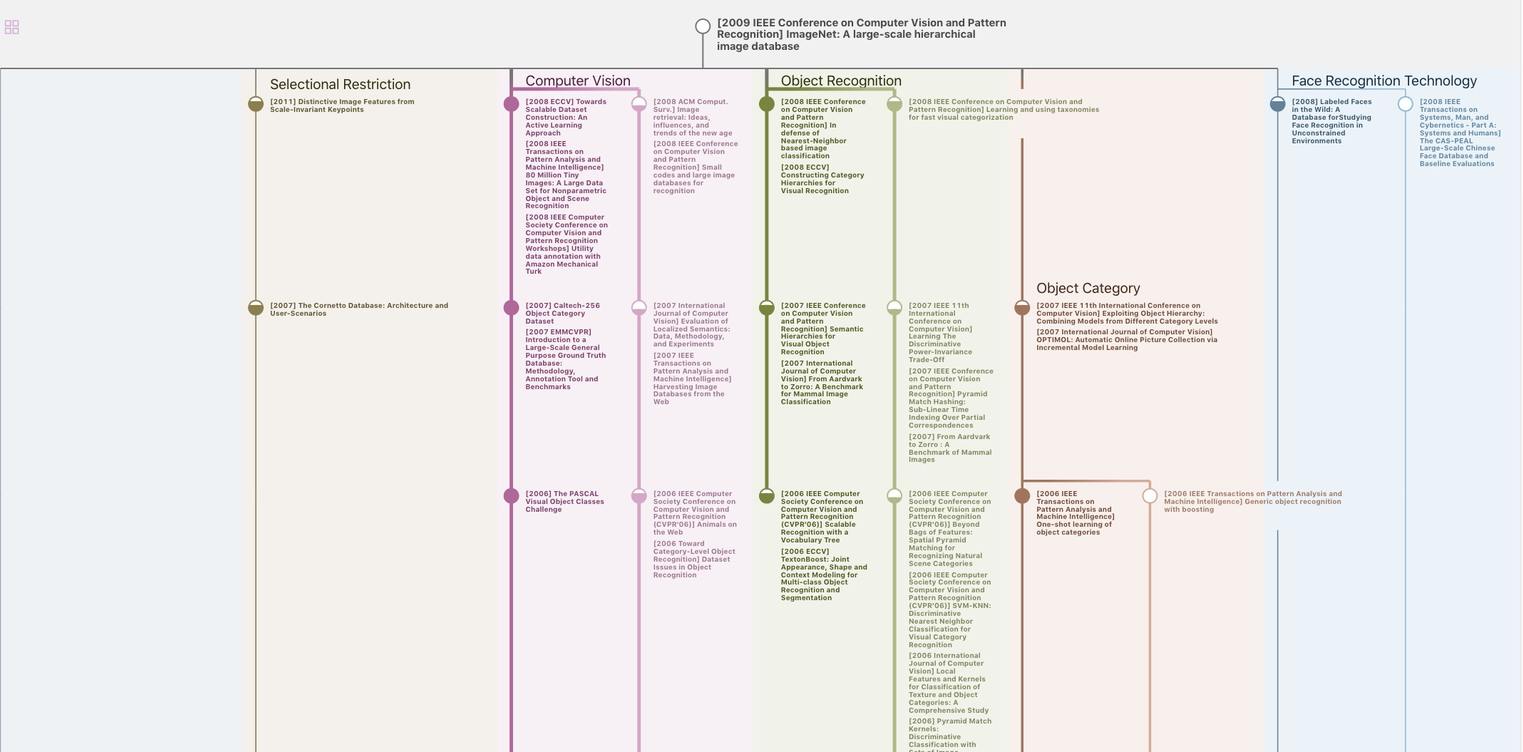
生成溯源树,研究论文发展脉络
Chat Paper
正在生成论文摘要