PUFs Physical Learning: Accelerating the Enrollment via Delay-Based Model Extraction
IEEE Transactions on Emerging Topics in Computing(2022)
摘要
The introduction of Physical Unclonable Functions (PUFs) has been originally motivated by their ability to resist physical attacks, particularly in anti-counterfeiting scenarios. In these one-way functions, machine learning, cryptanalysis, and side-channel attacks are common attack vectors threatening the promised PUF's property of unclonability. These attacks often emulate a PUF by employing a large number of Challenge-Response Pairs (CRPs). Some solutions to defeat such attacks are based on a protocol, where a model of the underlying PUF primitives should be extracted during the enrollment phase. In this article, we introduce a novel physical cloning approach applicable to FPGA-based implementations, which allows extracting the PUF's unique physical characteristics with a few number of Challenge-Response Pairs (CRPs), that increases only linearly for a higher number of PUF components. Indeed, our proposed approach significantly accelerates the enrollment phase and makes complex enrollment protocols feasible. Our core idea relies on an on-chip delay sensor, which can be realized by ordinary FPGA components, measuring the unique characteristic of the PUF elements. We demonstrate the feasibility of our introduced technique by practical experiments on different FPGA platforms, cloning a couple of (complex) PUF constructions, i.e., XOR APUF, iPUF, composed of delay-based Arbiter PUFs.
更多查看译文
关键词
Physical unclonable functions,machine learning,FPGA,voltage drop sensor,physical learning,cloning
AI 理解论文
溯源树
样例
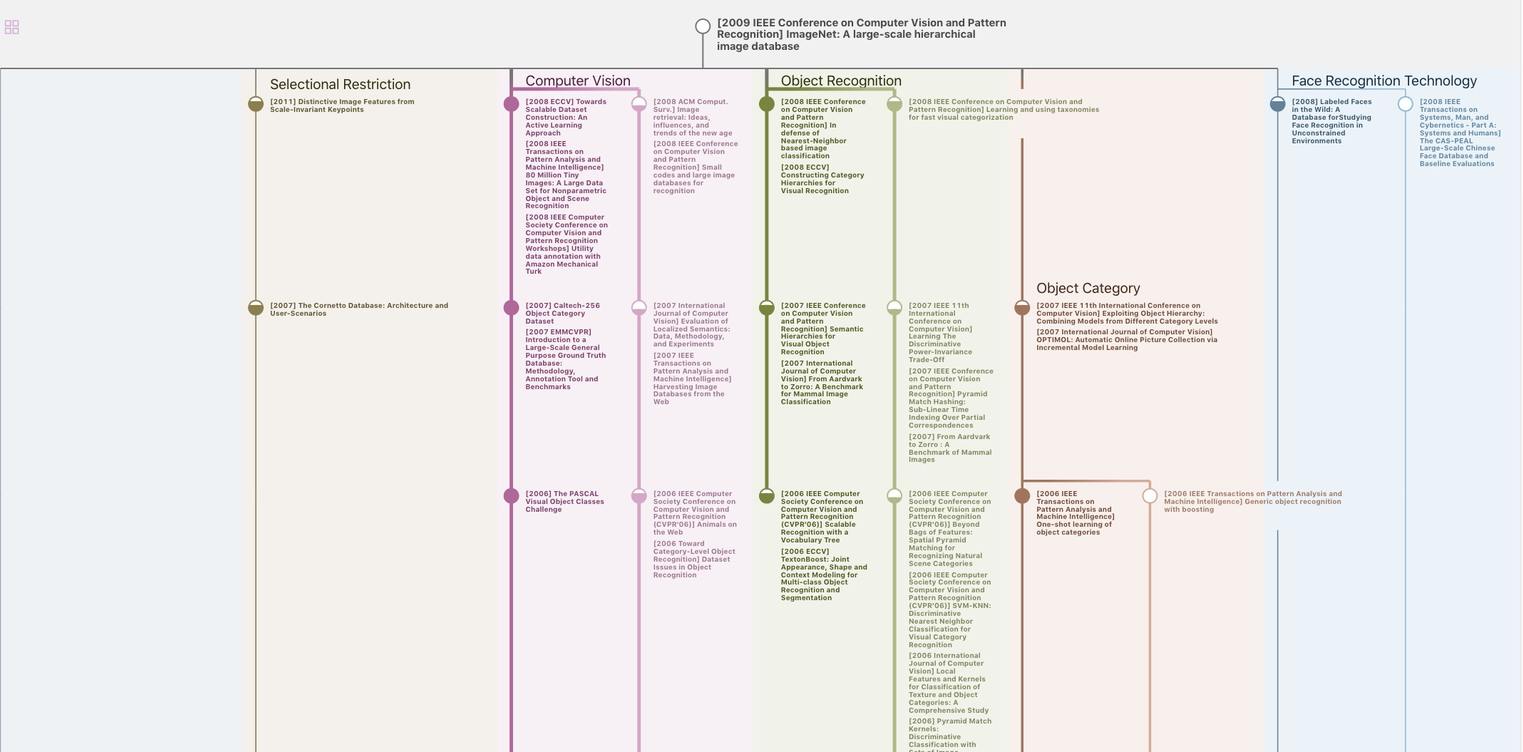
生成溯源树,研究论文发展脉络
Chat Paper
正在生成论文摘要