SpeedyIBL: A Solution to the Curse of Exponential Growth in Instance-Based Learning Models of Decisions from Experience
arxiv(2021)
摘要
Computational cognitive modeling is a useful methodology to explore and validate theories of human cognitive processes. Often cognitive models are used to simulate the process by which humans perform a task or solve a problem and to make predictions about human behavior. Cognitive models based on Instance-Based Learning (IBL) Theory rely on a formal computational algorithm for dynamic decision making and on a memory mechanism from a well-known cognitive architecture, ACT-R. To advance the computational theory of human decision making and to demonstrate the usefulness of cognitive models in diverse domains, we must address a practical computational problem, the curse of exponential growth, that emerges from memory-based tabular computations. When more observations accumulate, there is an exponential growth of the memory of instances that leads directly to an exponential slow down of the computational time. In this paper, we propose a new Speedy IBL implementation that innovates the mathematics of vectorization and parallel computation over the traditional loop-based approach. Through the implementation of IBL models in many decision games of increasing complexity, we demonstrate the applicability of the regular IBL models and the advantages of their Speedy implementation. Decision games vary in their complexity of decision features and in the number of agents involved in the decision process. The results clearly illustrate that Speedy IBL addresses the curse of exponential growth of memory, reducing the computational time significantly, while maintaining the same level of performance than the traditional implementation of IBL models.
更多查看译文
关键词
exponential growth,learning models,speedyibl,instance-based
AI 理解论文
溯源树
样例
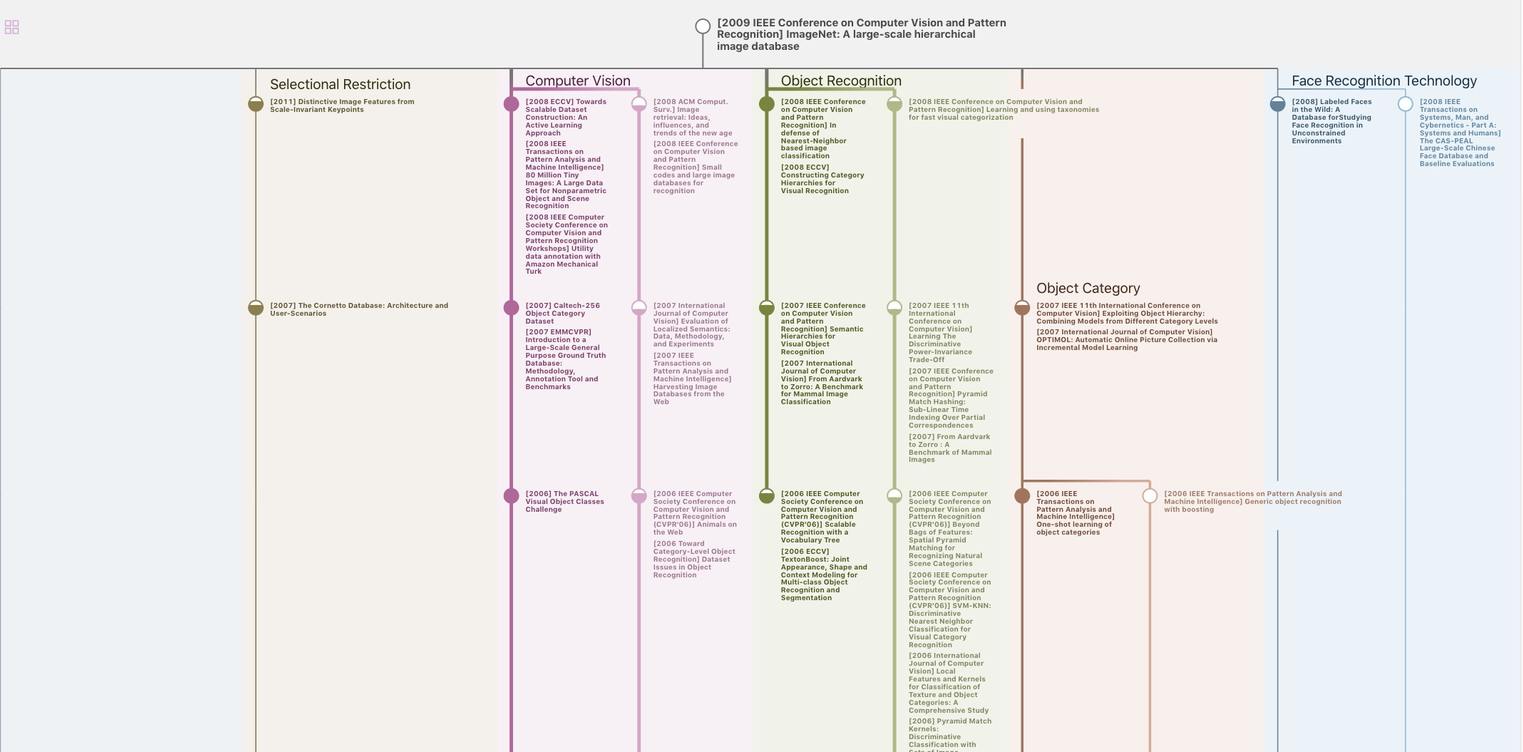
生成溯源树,研究论文发展脉络
Chat Paper
正在生成论文摘要