Meta Clustering Learning for Large-scale Unsupervised Person Re-identification
International Multimedia Conference(2022)
摘要
ABSTRACTUnsupervised Person Re-identification (U-ReID) with pseudo labeling recently reaches a competitive performance compared to fully-supervised ReID methods based on modern clustering algorithms. However, such clustering-based scheme becomes computationally prohibitive for large-scale datasets, making it infeasible to be applied in real-world application. How to efficiently leverage endless unlabeled data with limited computing resources for better U-ReID is under-explored. In this paper, we make the first attempt to the large-scale U-ReID and propose a "small data for big task" paradigm dubbed Meta Clustering Learning (MCL). MCL only pseudo-labels a subset of the entire unlabeled data via clustering to save computing for the first-phase training. After that, the learned cluster centroids, termed as meta-prototypes in our MCL, are regarded as a proxy annotator to softly annotate the rest unlabeled data for further polishing the model. To alleviate the potential noisy labeling issue in the polishment phase, we enforce two well-designed loss constraints to promise intra-identity consistency and inter-identity strong correlation. For multiple widely-used U-ReID benchmarks, our method significantly saves computational cost while achieving a comparable or even better performance compared to prior works.
更多查看译文
关键词
clustering,meta,learning,large-scale,re-identification
AI 理解论文
溯源树
样例
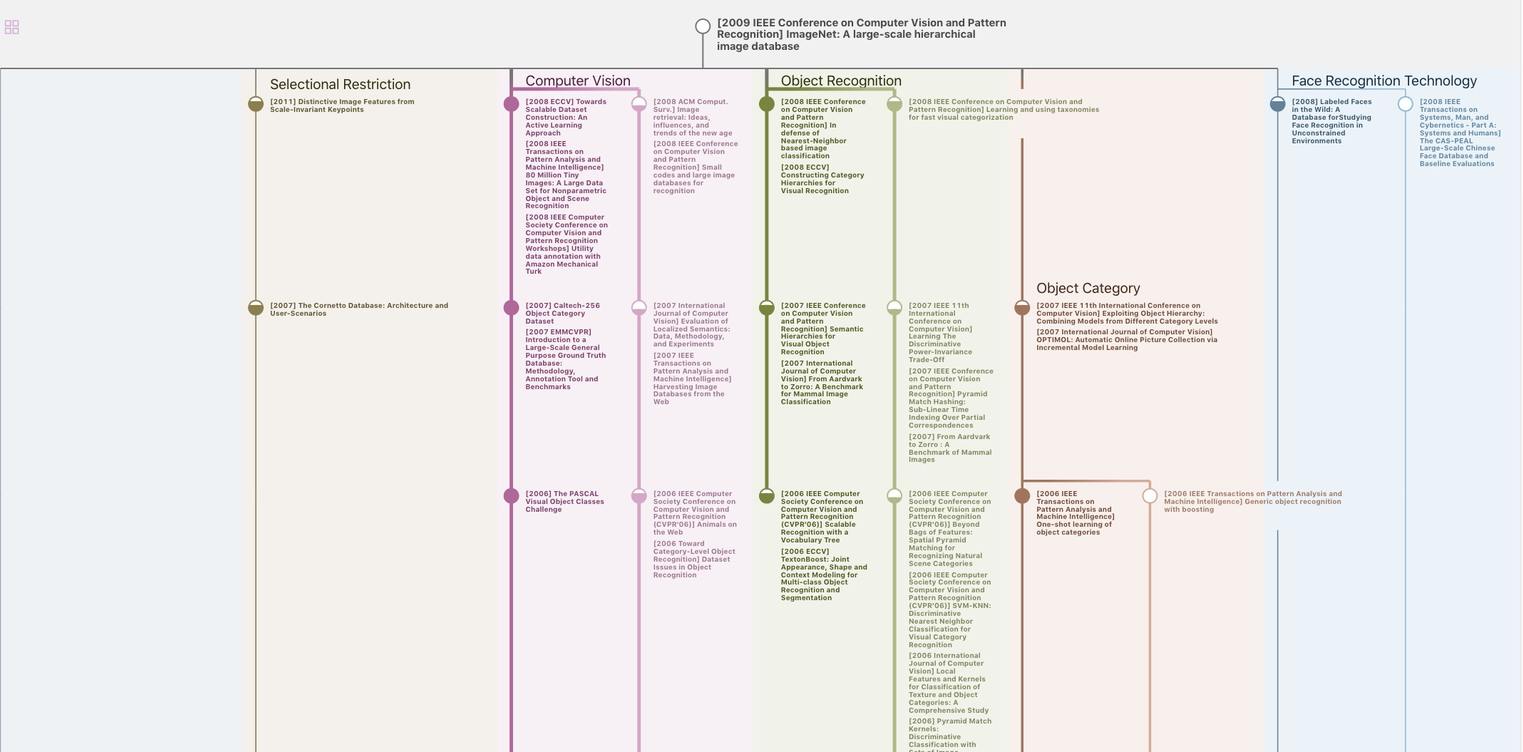
生成溯源树,研究论文发展脉络
Chat Paper
正在生成论文摘要