Detection of live breast cancer cells in bright-field microscopy images containing white blood cells by image analysis and deep learning
JOURNAL OF BIOMEDICAL OPTICS(2022)
摘要
Significance: Circulating tumor cells (CTCs) are important biomarkers for cancer management. Isolated CTCs from blood are stained to detect and enumerate CTCs. However, the staining process is laborious and moreover makes CTCs unsuitable for drug testing and molecular characterization. Aim: The goal is to develop and test deep learning (DL) approaches to detect unstained breast cancer cells in bright-field microscopy images that contain white blood cells (WBCs). Approach: We tested two convolutional neural network (CNN) approaches. The first approach allows investigation of the prominent features extracted by CNN to discriminate in vitro cancer cells from WBCs. The second approach is based on faster region-based convolutional neural network (Faster R-CNN). Results: Both approaches detected cancer cells with higher than 95% sensitivity and 99% specificity with the Faster R-CNN being more efficient and suitable for deployment presenting an improvement of 4% in sensitivity. The distinctive feature that CNN uses for discrimination is cell size, however, in the absence of size difference, the CNN was found to be capable of learning other features. The Faster R-CNN was found to be robust with respect to intensity and contrast image transformations. Conclusions: CNN-based DL approaches could be potentially applied to detect patient-derived CTCs from images of blood samples. (C) The Authors. Published by SPIE under a Creative Commons Attribution 4.0 International License.
更多查看译文
关键词
cancer cell, deep learning, imaging, tumor, convolutional neural networks, cell detection, cell classification, cell localization
AI 理解论文
溯源树
样例
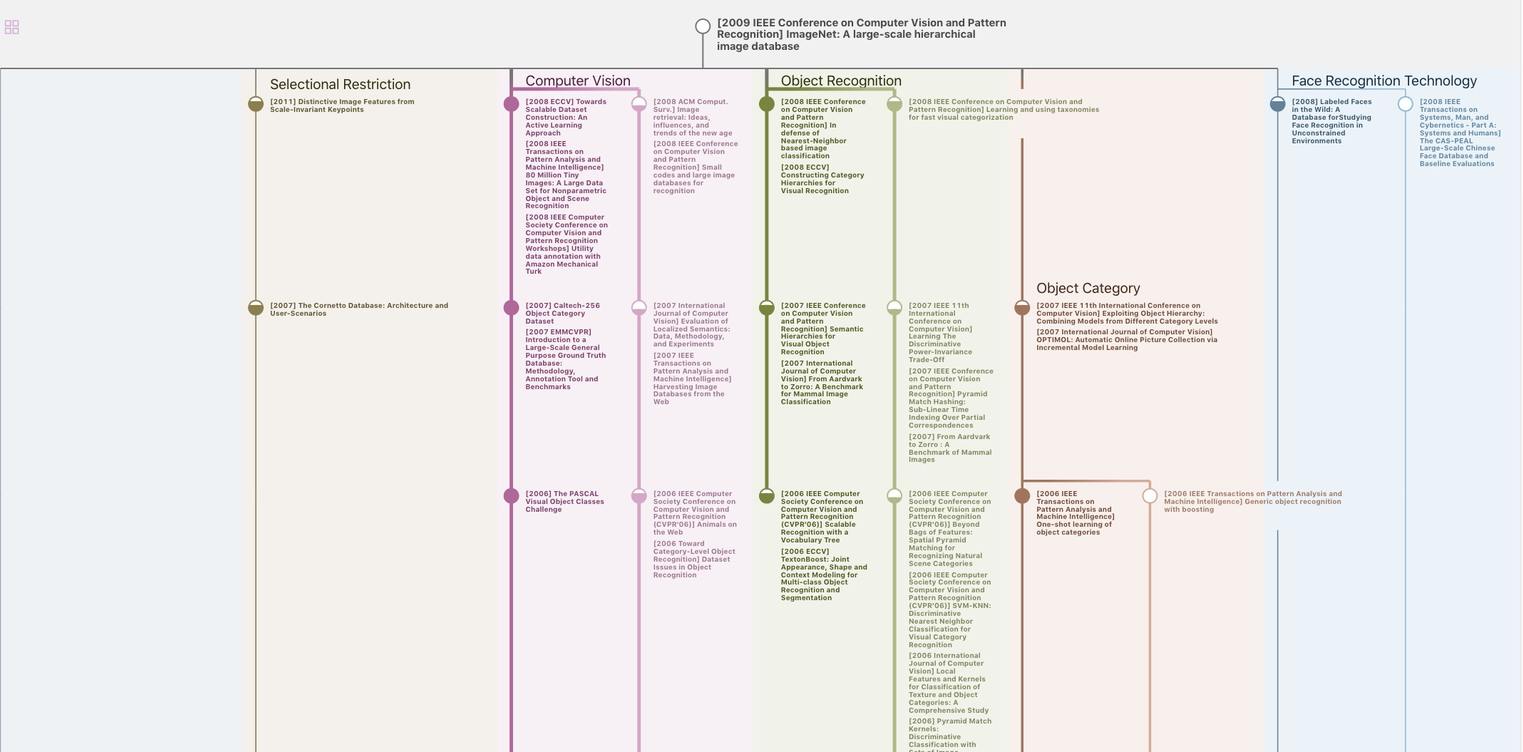
生成溯源树,研究论文发展脉络
Chat Paper
正在生成论文摘要