Machine learning-based library design improves packaging and diversity of adeno-associated virus (AAV) libraries
biorxiv(2021)
摘要
AAVs hold tremendous promise as delivery vectors for clinical gene therapy. Yet the ability to design libraries comprising novel and diverse AAV capsids, while retaining the ability of the library to package DNA payloads, has remained challenging. Deep sequencing technologies allow millions of sequences to be assayed in parallel, enabling large-scale probing of fitness landscapes. Such data can be used to train supervised machine learning (ML) models that predict viral properties from sequence, without mechanistic knowledge. Herein, we leverage such models to rationally trade-off library diversity with packaging capability. In particular, we show a proof-of-principle application of a general approach for ML-guided library design that allows the experimenter to rationally navigate the trade-off between sequence diversity and fitness of the library. Consequently, this approach, instantiated with an AAV capsid library designed for packaging, enables the selection of starting libraries that are more likely to yield success in downstream selections for therapeutics and beyond. We demonstrated this increased success by showing that the designed libraries are able to more easily infect primary human brain tissue. We expect that such ML-guided design of AAV libraries will have broad utility for the development of novel variants for therapeutic applications in the near future.
One Sentence Summary Computational, data-driven re-design of a state-of-the-art therapeutically relevant AAV initial library improves downstream selection for therapeutic uses.
### Competing Interest Statement
D.Z., D.H.B., J.L., and D.V.S. are inventors on patent related to improving packaging and diversity of AAV libraries with machine learning. David V. Schaffer is a co-founder of 4D Molecular Therapeutics. Jennifer Listgarten is on the Scientific Advisory Board for Foresite Labs and Patch Biosciences. Other authors declare no competing interests.
更多查看译文
AI 理解论文
溯源树
样例
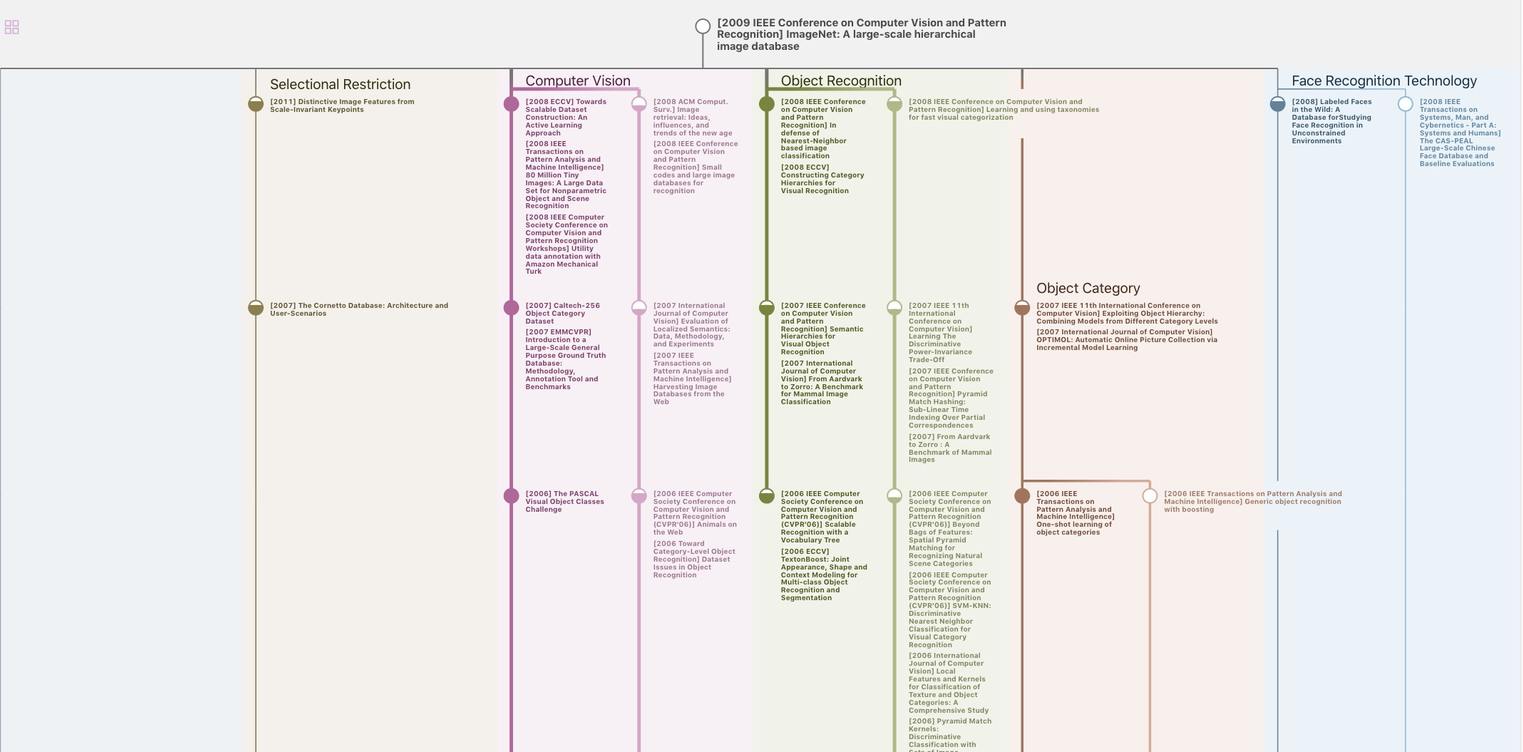
生成溯源树,研究论文发展脉络
Chat Paper
正在生成论文摘要